When Counting Steps and Tracking Sleep Is Just The Beginning – What’s Next for Fitbit?
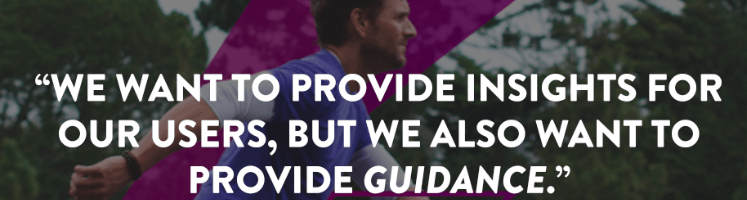
With the advent and further sophistication of machine learning, Fitbit is positioned to do more than just track your fitness and activity data.
US-based Fitbit is one of the leaders of the Wearable Device segment also known as “fitness trackers.” These products generate a lot of data as it relates to people’s sleep and activity cycles, data that the organization can use for product development purposes. Specifically, tracking and analyzing a user’s data can help Fitbit, through the use of machine learning, to recommend tailored and customized workout plans and sleep optimization strategies. [1]
The ability to provide customized fitness and sleep recommendations is incredibly powerful because it makes Fitbit’s fitness trackers that more valuable to consumers. [1] [2] Originally, in the early days of the fitness tracker industry, there was value in simply being aware of one’s activity and sleep levels, ie. how many steps have I taken and how many hours have I slept? Currently, now these trackers should be able to go one level deeper, providing provide users with personalized products in the form of customized workouts. [2]
Via their Health Solutions Group, Fitbit has focused on taking their use of user-generated data to a whole new level, focusing on how healthcare treatment can be optimized for users based on their unique data. [3] [4]
In the medium term, Fitbit is focused on providing additional machine learning-powered insights to their users via the integration of their recent 2/18/18 acquisition of Twine Health, a HIPAA-compliant health platform where patients can collaborate with doctors on the care plan for chronic diseases such as diabetes and hypertension. [3] Given Twine Health requires an element of tracking someone’s activity, weight, food intake, blood pressure, etc. this provides another area for machine learning to be able to help patients/providers. Through machine learning, not only will patients and providers know quickly if people are improving or worsening in terms of the symptoms of their conditions, but the technology can provide actionable and real-time recommendations on changes that will improve patient health. [5]
In the medium term, they are focused on building out their 4/30/18 partnership with Google to collaborate on the Wearables and Health space. [4] While some of the collaboration is geared towards combining activity data with people electronic medical records, the real value will be in their ability to use Google’s capabilities to analyze the fitbit user data [6]. Specifically, Fibit will move their user data to the Google Cloud platform where they will be able to leverage “Google’s AI and machine learning capabilities and new predictive analytic algorithms to bring more meaningful data and insights to consumers.” [4]
In my humble opinion, while Supervised Machine Learning enables recommending personalized changes in activity, food intake, sleep, etc. that increase positive individual health outcomes, the true value for the Company will come from its ability to better understand the broader hidden “patterns and insights” within all of Fibit’s user data.
With this in mind, additional steps to take in the short run are to further look at the Fitbit user data via Unsupervised Machine Learning once they’re able to merge their data with patient electronic medical records. Are there certain predictors of chronic diseases? At this point, Fitbit should have a large enough user base that you can look at historical data and ask, what were some of the characteristics exhibited by people who ultimately exhibited hypertension, diabetes, and other chronic conditions? Additionally, what sort of behaviors (poor sleep, poor activity, high saturated fat diets, etc.) potentially led to those diseases? To accomplish this, you will need additional medical record data to further augment the data set so that you can include user data related to age, medical conditions, medicines taken, smoking status, etc. By reviewing all of this data in aggregate and understanding its structure, over time you can start identifying correlations between poor health and specific actions taken in the past. Although there are multiple attributes/actions that may ultimately lead to disease (gene predisposition, smoking, low activity, poor eating habits etc.) and it could be hard to isolate actions, you can see across a population, which of these attributes/actions are present in the average person with diabetes, for example. As a result, as time passes on, we are seeing a more holistic approach to medicine where many things contribute to someone’s well being, and armed with the insights from machine learning, you will be able to systematically employ preventive medicine.
However, a few more things are definitely worth considering as it relates to machine learning used to create actionable insights from wearable devices data. First, wearable devices for the most part are still not FDA endorsed, how can we use machine learning to better support the health insights derived from the data? Finally, more of a fun question, how does machine learning explain how there are people like Warren Buffett who eat McDonald’s and drink Coca Cola regularly but remain in great health?
(Word Count 799)
References:
[1] Mixpanel. (2017). Why 2017 is Fitbit’s year of machine learning – The Signal. [online] Available at: https://mixpanel.com/blog/2017/01/31/fitbit-machine-learning/
[2] Blogs.umass.edu. (2018). Fitbit, Machine Learning, and Sleep Optimization | Techbytes. [online] Available at: https://blogs.umass.edu/Techbytes/2017/02/14/fitbit-machine-learning-and-sleep-optimization/ [Accessed 13 Nov. 2018].
[3] Investor.fitbit.com. (2018). Fitbit, Inc. to Acquire Twine Health. [online] Available at: https://investor.fitbit.com/press/press-releases/press-release-details/2018/Fitbit-Inc-to-Acquire-Twine-Health/default.aspx
[4] Investor.fitbit.com. (2018). Fitbit and Google Announce Collaboration to Accelerate Innovation in Digital Health and Wearables. [online] Available at: https://investor.fitbit.com/press/press-releases/press-release-details/2018/Fitbit-and-Google-Announce-Collaboration-to-Accelerate-Innovation-in-Digital-Health-and-Wearables/default.aspx
[5] Penman, B. (2018). Fitbit Acquires Health-Coaching Company Twine, In A Move To Be A More Serious Tool. [online] News. Available at: https://www.wgbh.org/news/2018/03/05/science-and-technology/fitbit-acquires-health-coaching-company-twine-move-be-more-serious
[6] MobiHealthNews. (2018). Google, Fitbit collaboration aims to deliver comprehensive health data to care teams. [online] Available at: https://www.mobihealthnews.com/content/google-fitbit-collaboration-aims-deliver-comprehensive-health-data-care-teams
As a long-time Fitbit wearer, I am always intrigued monitoring my activity patterns as they relate to my health and well-being. I constantly track my steps, heart rate and sleeping patterns with no real conclusions besides I don’t sleep enough…
I would think, to your point, there would be a way to mine this data on an individual basis to make broader conclusions about health, locate precursors to certain diseases, recommend activities and habitual changes that would help make individuals more healthy. I would think too with more and more data there would be a way to partner with hospitals as well as insurance companies to promote a healthy lifestyle. I would think that all these collaborators would be in favor of improved machine learning to learn and make predictions about health based on the data from Fitbit; the machine could serve as such a tremendous partner to physicians in this capacity. Never replacing a physician in my opinion but could certainly serve as a lethal partner.
Like Christie above, I too am a long-time Fitbit wearer and am constantly checking my sleep and steps to ensure that I am “healthy”. The problem I have is that healthy is a metric I define and the conclusions I make are essentially my opinions (i.e. I don’t sleep enough, I should be more active). I really like the above thoughts on using machine learning to understand health conditions. If it was scientifically proven that if I took 15K steps per day I had a better chance of avoiding disease, I’m sure I would make the time. The one issue I do see is people being okay with their data used in this manner. I as a consumer would be concerned that you were pairing my medical records with my Fitbit patterns. Though you said “Unsupervised Machine Learning” as an everyday consumer I am not totally sure what that means and how private or unsupervised it truly is.
I am also quite intrigued by your fun question above. While I am not sure how you use machine learning to see how people with unhealthy habits live long lives, I do wonder if there is any common factor in these people besides genetics. Maybe that is something that machine learning can look into!
You mentioned in your analysis that “the true value for the Company will come from its ability to better understand the broader hidden “patterns and insights” within all of Fibit’s user data”. While I agree with your assessment, I also wonder how human intelligence can help augment and make decisions based off of the prediction element of the Machine-Learning-powered insight that comes with analyzing the data. I also wonder whether there is an inherent bias that exists from the data set that is being collected by Fitbit. In other words, most people who wear a Fitbit device are already health conscious and likely making healthy decisions and taking health-oriented actions on a day to day basis. I wonder how useful is this data when Fitbit attempts to scale the application to the general population. Can it be scaled and applied on an apple to apple basis or do we do need to start from scratch and use a completely new set of data, in which case would render Fitbit’s data useless?
As another consumer who tracks my steps and activity regularly, I think this has great potential to improve people’s health. However, there are some regulatory issues with this, where does the FDA come in with regards to personalized medical recommendations that are made through AI and how does industry government and the public come together to solve this? This is a question is common with several other areas of AI and one that will permeate out society in the decades ahead.
Thank you for the thorough research and thoughtful article! I totally agree with your opinion that a large part of the value Fitbit (and similar technologies) can provide are in providing individual, and collective, pattern recognitions and recommendations. An aspect of health that Fitbit and competitors seem to have not looked into is mental health – how are people’s activity level, sleep cycle, etc. affecting their mental health (not just physical health such as indicated by diseases)? One reason they might not have looked into it is that while diseases (or prevention of) can be quantified, quality of life measures such as mental health are less susceptible to be valued. Another related reason is that while Fitbit can draw on clinical data to measure relationship between disease and input data, mental health is a spectrum that might require people to self-report their health level – and data collection that requires actions on the end of the customer is difficult. I do hope that some day this would be addressed though!
In addition to many of the comments above, I too am a fitness device wearing individual (albeit Apple watch and not Fitbit). Given my love for running and active workouts, I am extremely intrigued about the next level FitBit and other companies can take this technology. I too find it extremely valuable to have a watch tell me that if I take x number of steps in a day or increase my heart rate for y minutes per day then I will not get a certain disease. Obviously, the risk is that an individual might be predisposed to the disease and it doesn’t matter how much he or she works out. Machine learning can only help so much – I don’t know how a company will be able to account for an individual’s predisposition to a certain disease. Nonetheless, I find it extremely valuable that a piece of wearable technology can provide me with a preventative treatment plan and can monitor my progress on that plan.
Intriguing article! I would be curious to see what Fitbit does to integrate the data that exists across the healthcare provider landscape. One of the major challenges of the U.S. healthcare industry is the lack of coordination of data for any particular patient, both longitudinal data as well as data scattered across multiple providers. One area that Fitbit could focus on is becoming the central repository of the data on any specific individual through the course of their life, and play a key role in the penetration of outcomes-based payments.
The second question is also interesting, but in the realm of what Fitbit does, we are only talking about behavioral health outcomes, rather than genetic!
I believe there is a lot of potential in personalized nutrition with FitBit and other biometric tracking devices. The co-founder of a 3-D food printer has said “You [could] connect your Fitbit to your food printer and it can print a breakfast bar, for example, that’s appropriate for you on that given day,” (https://www.businessinsider.com/3d-printed-food-foodini-2016-4). The devices already exist (FitBit, 3-D food printers); what still needs to be refined to make this a reality is the recommendation engine that links biometrics to nutrition, and the communication ability between these two different devices.