Uber: Future challenges in the era of AI and ML
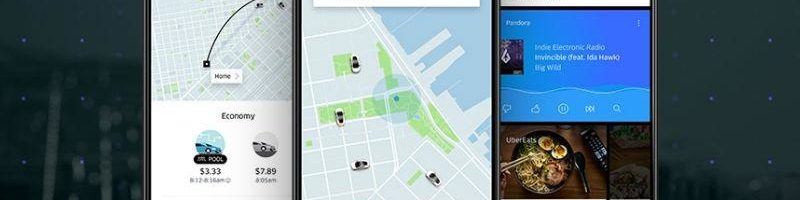
Uber has been successful in implementing machine learning to solve critical business problems and bridge the gap between the physical and digital world. Claiming itself a technology platform, Uber collects a huge amount of data from both drivers and riders and iterates quickly with various experiments for product development and operational improvement. The company uses ML for a very diverse set of applications. Rather than applying ML to a few key areas such as ad optimization or content relevance like social media platforms, Uber has a much more even spread of ML solutions. Multiple algorithms are deployed, impacting many drivers and customers and helping Uber get more efficient as it scales. However, as the megatrend of machine learning gets bigger, it is critical that Uber management team needs to keep in mind the ethics aspect in algorithms getting deployed.
Uber’s Marketplace team uses various predicting models to forecast where customer demand and driver availability will be at different times and places in the future. Based on those imbalances between demand and supply, Uber encourages driver-partners ahead of time where and when to drive via a product feature called surge pricing. Also, it also predicts the driving pattern of drivers in order to give out incentives to drivers on a weekly basis. This helps Uber get more efficient compared to taxis and other ride-hailing competitors, riders get reliable rides with low ETAs, and drivers don’t have to drive around to pick up riders. However, when algorithms get implemented, drivers usually get angry. The more they drive, the less incentive they receive; the algorithm predicts that they would drive long hours regardless so there is no point of incentivizing with a weekly bonus. On the other hands, there are cases where drivers work too hard in order to hit the weekly bonus target, severely impacting their health.
This is an important issue because physical and mental health of drivers are at stake, while the algorithm has no idea about the hidden cost as it is designed to gamify and optimize for certain metrics. To address this issue, in the short term, Uber management teams is currently carefully examining algorithms that get deployed and intervene in the case that the algorithms get out of control. Operation teams constantly provide feedback to the data science team whenever there is a backlash from driver partners. Regarding other steps the company should take, short term, it should foster more collaboration between teams to gather better insights from drivers and riders to incorporate ethical elements in the product design and development process. Drivers partners should have a voice in how algorithms are pushed to them and product features that they use every day. Also, it should implement and champion more balanced business metrics like customer satisfaction score, safety metrics, etc. instead of purely focusing on top line growth. This will continue to become a big challenge as Uber is going public next year, facing more pressure from investors to grow more efficiently.
The company is experimenting with the idea of self-driving cars and trucks. In the United States alone, it is estimated to be 3.5 million truck drivers and 8,7 million people employed in the industry. AI experts predict that in the future, those jobs will be replaced by self-driving vehicles. Currently, Uber seems to not be worried too much about the future of replacing human drivers with self-driving cars as the future of self-driving vehicle is still far ahead. It is pushing hard to grow the driver-partners base every day. However, in the long term with self-driving cars and trucks start to get more popular, the company needs to think about the social implications and its responsibilities as a platform. Uber should also consider providing more benefits for drivers who are impacted by the implementation of machine learning and artificial intelligence.
In the context of problems that Uber is facing in the era of AI and ML, 2 open-ended questions for debate are:
- “Given that users and drivers give up a lot of their data to technology platforms like Uber, how much control do users and drivers have and values are they be able to capture?
- What is the role of government in making sure technology platforms positively contribute to society? What are the implications on “losers” in the megatrend of machine learning?
The author questions the role of government in making sure technology platforms positively contribute to society. The government can force big data companies to open APIs and allow 3rd parties to study and work onto consumers’ data. If my data is out there, and owned by Uber, it might as well be public.
Uber is a great example of how technology has led to this incredibly valuable service which has revolutionized transportation and has the potential to continue driving innovation, especially through the use of machine learning. Uber’s rise also shows the second and third order consequences, in this case the detrimental effect on drivers as Uber pushes to become more efficient. In some ways, Uber’s contribution to the gig economy has allowed many people to generate income in a way that is flexible to their schedules. But, it is also forcing drivers to perform more and more work to make any significant profit. I personally think Uber needs to do more to invest in their drivers and begin to treat them more as employees, especially the ones who clearly drive Uber as their full time jobs. Uber should set a model of corporate responsibility by mitigating the impact of machine learners on the “losers” of this megatrend.
Thank you for the article. It was really insightful to provide an overview of the Uber-driver partner relationship. I think the growing complexity and capability of ML algorithms poses as a huge temptation to overexploit the less powerful – the driver. Gaining perfect information on an individual driver’s patters (and therefore ex-ante motivations) is very valuable for Uber and puts the driver at a loss. Proving a dynamically evolving incentive algorithm targeted individually at each driver can easily squeeze all possible efforts out of every driver at the lowest cost possible – just like you pointed in your example. I think the only ethically acceptable solution here is to hold every driver to the same incentive scheme, so that the hard workers earn their fair share. Moreover, since I am learning about these mechanisms from an Uber expert and not my own Uber app (which I use frequently), makes me worry about the transparency of ML applications at the firm. I feel every time news like this break out, I seem to trust Uber less and less. Due to their size and impact on urban ecosystems, they should be held to highest standards of public service and do more than currently with respect to how their new technologies are affecting both sides of the market they co-create.