Doctors in china leverage machine learning to improve efficiency and effectiveness
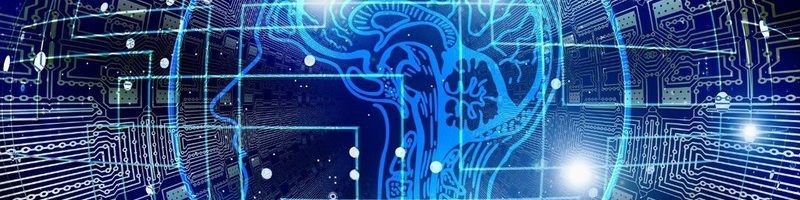
How doctors push AI startups to innovate in medical field.
Hundreds of samples need to be processed under the microscope by the naked eyes of experienced doctors each day at Nanfang hospital, the No.1 hospital in China for cervical cancer diagnosis and treatment.
Cervical cancer is the third most common and among the deadliest cancers in women worldwide, with a third of new cases occurring in China. Due to screening efforts and improved treatment championed by the government, cervical cancer has seen a steady decrease in death over the past few decades. However, the doctors are still in scarcity, especially in rural areas.
“It is normal for us to stay past midnight looking at those samples.” One doctor at Nanfang Hospital said, “We don’t have enough people to read the number of samples we receive each day.”
In October 2017, China’s State Council announced the aim of becoming a global leader in artificial intelligence, which worth $150 billion domestically by 2030. Given the government support, hospitals started to welcome AI startups to collaborate on developing the best in class medical imaging AI technologies. The country’s medical AI industry scale is estimated to reach 20 billion yuan in 2018, surging 53.8%. Nanfang hospital led the initiative in cervical cancer. The AI startup partners benefited from the large sample size of cervical cancer images (>100,000) to train their algorithms. It takes three very experienced doctors to collectively diagnose for hours to reach the accuracy of 83%, whereas the new technology Nanfang hospital and its AI startup partner has co-developed could reach an accuracy of 95%+ in just 2 seconds.
“It is a learning process, not just for machines, but for doctors and software engineers.” The doctors at Nanfang hospital hosted a month-long workshop to teach the CTO and lead engineers of their medical AI technology partner the pathology and etiology of cervical cancer.
“None of us had biology or medical background. It was very difficult to understand. So, we went back, bought textbooks of molecular biology, and other medical books ourselves to read.” The CTO of the medical AI startup said. “After feeding the data, we need to teach the doctors how to read those results and describe how the machine learning and the algorithms work. If pathology is hard for us, coding is equally hard for them doctors. But once the doctors understand how it works, they provide tremendous value on how we could improve our algorithms.”
“AI imaging is just the first step. What we envision is the hospital of the future, where machine learning is improving the patient/doctor interface, diagnosis process, treatment, as well as drug discovery. Patients do not need to travel all the way to our hospital to get diagnosed. We’d like to develop equipment that can help doctors to diagnose remotely and virtually.” The department head of Nanfang hospital said.
In the short term, Nanfang hospital wants to improve the accuracy of the image reading process by leveraging the AI startups. The biggest opportunity here is for those negative readings, given that majority of the readings will be negative, it drastically decreases the time needed from doctors. “95%+ is not enough. We need the technology to be 99%+ accurate, if not 100%, to have real impact.” The head of the department said.
In the long term, Nanfang hospital wants to utilize machine learning and AI to solve the talent gap problem. The long hours of doctors nowadays make students turn away. With machine learning technologies, Nanfang hospital hopes to improve the work/life balance of doctors, therefore to attract more talents. Nanfang hospital plans to build its own college of pathology, equip them with both the medical knowledge as well as cutting edge, disruptive technologies including machine learning and artificial intelligence.
Machine learning is still in its early days, how to make it practical and scalable in the hospital context is still a big question that Nanfang hospital wants to tackle. “There is a knowledge gap between medical imaging AI vs. reality.” The doctor said, “Breast cancer is fairly easy to diagnose. Most medical AI companies developed their algorithms for breast cancer because it is not that hard, which in real life, does not add much value because there is not a bottleneck there. On the other hand, the harder problems, including pathology, really needs talent and technology to improve the accuracy and efficiency. ” Nanfang hospital is fully aware of the buzz around medical AI companies vs. real applications in hospital settings. In the median terms, I’d recommend them to keep the focus on bringing along the software engineers to understand the medical field, to develop technologies that have actual impact.
Do you think Nanfang hospital’s grand vision to build hospital of the future using cutting edge technologies including machine learning is achievable?
(800 words)
References
Chinadaily.com.cn. (2018). Future of healthcare could lie in artificial intelligence technology – Chinadaily.com.cn. [online] Available at: http://www.chinadaily.com.cn/a/201808/07/WS5b68f296a3100d951b8c8f4e.html [Accessed 13 Nov. 2018].
Das, R. (2018). China And Israel Are Ready To Battle: Who Leads the Medical Imaging Artificial Intelligence Market?. [online] Forbes. Available at: https://www.forbes.com/sites/reenitadas/2018/06/26/china-and-israel-are-ready-to-battle-who-leads-the-medical-imaging-artificial-intelligence-market/#e8d99ae59fc3 [Accessed 13 Nov. 2018].
South China Morning Post. (2018). Can AI help fix China’s ailing health care system?. [online] Available at: https://www.scmp.com/tech/china-tech/article/2153144/china-counts-ai-find-cure-its-ailing-health-care-system [Accessed 13 Nov. 2018].
Sun, Y. (2018). AI could alleviate China’s doctor shortage. [online] MIT Technology Review. Available at: https://www.technologyreview.com/s/610397/ai-could-alleviate-chinas-doctor-shortage/ [Accessed 13 Nov. 2018].
Xinhuanet.com. (2018). China Focus: AI beats human doctors in neuroimaging recognition contest – Xinhua | English.news.cn. [online] Available at: http://www.xinhuanet.com/english/2018-06/30/c_137292451.htm [Accessed 13 Nov. 2018].
This is a really interesting space for machine learning! I would be curious to know if (in the short-term or medium-term) Nanfang plans to use this technology for human augmentation (e.g. still relying on a doctor to double-check before diagnosis) or replacement (e.g. use the algorithm’s result as the diagnosis). If it’s the latter, I definitely think that their focus should be on improving accuracy. If it’s the former, I would imaging that they could expand into other forms of cancer detection more quickly.
As the technology progresses, I would be interested to know if the technology can evolve to a point where its detection accuracy is better than that of a human.
Very interesting! I really enjoyed this piece. I would comment here that Nanfang Hospital’s focus on pathology in particular (e.g., with regards to cervical cancer images) is just one piece of the puzzle here. Machine learning is of course extremely applicable to medical fields like pathology, radiology, and dermatology, given that these are visual fields that require straightforward diagnoses. For instance, in pathology, you review a slide of cervical tissue and determine thereafter whether the patient has cancer or not. The same basic process applies in radiology and dermatology (reviewing images to identify if a patient has a particular diagnoses). However, I would argue that the application of machine learning to diagnosis in more cognitive fields (like primary care or neurology) are more challenging. In these cases, diagnosis is more of an art than a science — it requires clinical suspicion regarding possible diagnoses and then appropriate follow-up (e.g., laboratory testing and imaging) to explore these possible diagnoses. In my opinion, this is a more difficult issue to solve than the application of machine learning to pathology, radiology, or dermatology.
The primary reason why I bring up this information in the context of this piece is because the author states that Nanfang Hospital wants to use machine learning to solve the issue of long hours for doctors. I would argue that this is not necessarily going to occur with the application of machine learning to the fields of pathology, radiology, or dermatology (which are some of the best lifestyle specialties). In order to leverage machine learning to truly improve physician work-life balance, Nanfang Hospital needs to focus on its applications to diagnosis within the cognitive specialties in addition to specialties like pathology.
I really enjoyed reading your essay. I love how AI is used to tackle life-and-death problems, to improve efficiency in the health industry, and to improve medical doctors’ lives. I believe (and hope) that the hospital’s vision to build hospital of the future using cutting edge technologies including machine learning is achievable. As you mentioned, machine learning is at baby steps phase nowadays. I think as technology and machine learning advance, their applications in health sector will advance as well given that health sector is one of the most critical areas in the world and machine learning applications is highly valuable and meaningful in this area.