The Power of Patient Data: How Flatiron Is Combining Big Data and Machine Learning to Revolutionize Cancer Care

With their machine learning-enabled OncoEMR platform, Flatiron is giving oncologists the power of big data at the point of patient care.
From the early 1990s, when the Institute of Medicine pioneered the introduction of electronic medical records (EMR), until 2012 when Zach Weinberg and Nat Turner founded Flatiron Health, the fundamental principles underlying these systems remained relatively constant [1] [2]. They were viewed primarily as a way to improve accessibility to and continuity of information for medical professionals by ensuring that a patient’s complete medical history was collected and stored in one place. For the industry standard EMR systems like EpicCare, this required significant flexibility to accommodate the full universe of medical specialties and sites of care [3]. To achieve this level of flexibility, most systems sacrificed the ability to aggregate and structure data in an easily searchable format. Flatiron set out to address this problem with OncoEMR, an EMR system designed specifically for oncology care [2].
The company’s biggest challenge was finding a way to convert the unstructured data – low-resolution PDF lab reports, audio files, and digital copies of hand-written notes – that was typical of traditional EMR systems into structured data. Their solution relied on a variety of approaches, including matching algorithms to identify critical values in lab reports and natural-language processing to transcribe and then simplify the information contained in audio files. To optimize these processes, Flatiron used a hybrid human-machine learning model. Data gathered using the automated collection process was compared against data gathered by hand from a team of 50 nurses. This hand-collected data represented a training set that could be compared against the software-generated data to identify discrepancies, from which the algorithm could learn to improve its accuracy [4].
The resulting EMR system allowed oncology practices to easily transition from time-intensive and unwieldy legacy EMR providers to an oncology-specific platform optimized for efficiency. OncoEMR has been extremely successful to date, amassing 2.1 million active patient records [2]. Without the product attributes made possible through machine learning, it is unlikely that achieving this scale would have been attainable.
When anonymized and aggregated, the data generated by OncoEMR offers incredible potential, particularly when combined with machine learning. Not surprisingly, many of the growth opportunities that Flatiron is pursuing in the short term mimic the initial commercial applications of IBM Watson in healthcare [5]. OncoEMR data is being used to match patients with clinical trials in partnership with the National Institutes of Health (NIH) and to support the product’s own utilization management capabilities (helping physicians decide which combination of drugs to use when) [6]. In this latter area, Flatiron has a clear advantage over IBM: while Watson relies predominantly on published literature to inform utilization management decisions, Flatiron can combine published literature with real-world outcomes data from OncoEMR.
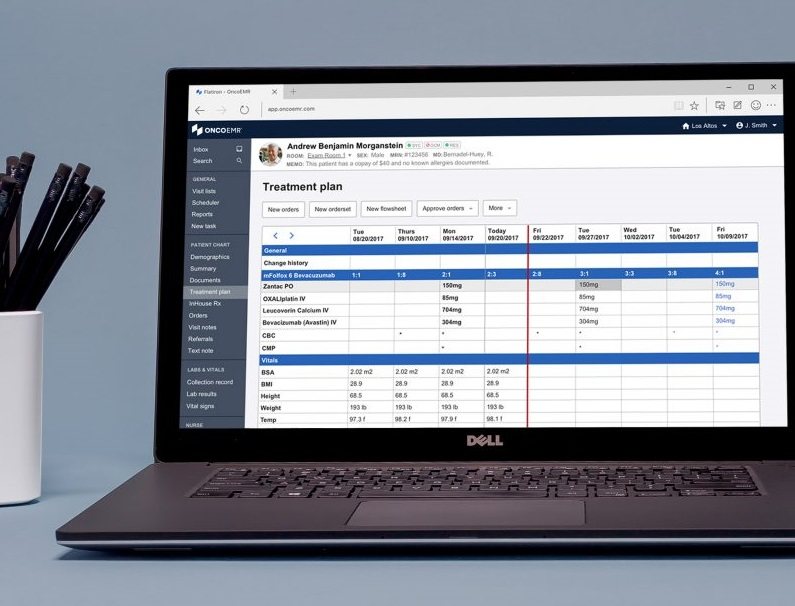
Source: Flatiron Health
In addition to the physicians and patients who help generate this data, Flatiron offers data packages to pharmaceutical companies and academic researchers [7]. This has proven to be a boon for oncology research, particularly retrospective outcomes studies. In only the last 3 months, Flatiron data has enabled 29 research abstracts, manuscripts, and published studies [8]. Roche, one of the world’s largest pharmaceutical companies, is so bullish on the prospects for this platform that they acquired Flatiron earlier this year for $1.9 billion [9]. In the medium to long term, Roche believes that this data could be used to replace the control arms of Phase III clinical trials, thus aiding in the development of new therapies [10] [11].
Still, these applications represent only a fraction of the possibilities for this technology. Instead of relying on humans to mine through the OncoEMR-generated data to produce publications, one could imagine a future in which machine learning enables Flatiron to automatically generate publication-grade research. Take, for example, triple negative breast cancer (TNBC) – a rare form of breast cancer that presents in the absence of HER2, estrogen receptor (ER), or progesterone receptor (PR) mutations and does not respond to the targeted therapies currently on the market. Some research exists to suggest that TNBC is actually a cluster of yet-uncategorized breast cancer subtypes [12]. Using patient-level data from OncoEMR, AI applications may be able to identify combinations of mutations or other laboratory findings that correlate with poor treatment outcomes in these patients. Findings like these would provide critical insight into the etiology of poorly understood cancers like TNBC. By identifying genetic targets, machine learning-enabled technology may even aid in the discovery of new drug therapies.
Of course, these opportunities are not without their challenges. What are the consequences of one company controlling a technology that has such incredible public health potential? And when it comes to drug development, how should the pharmaceutical industry weigh the trade-offs of using more cost-effective retrospective data from this platform against more reliable (but more costly) data generated from clinical trials?
(765 words)
References
[1] Gartee R, “Chapter 1: History and Evolution of Electronic Health Records,” Electronic Health Records: Understanding and Using Computerized Medical Records (3rd Edition), [https://www.pearsonhighered.com/content/dam/region-na/us/higher-ed/en/custom-product/gartee-electronic-health-records-3e/pdf/gartee3e-ch1.pdf], Accessed November 2018
[2] “About Us,” Flatiron Health, [https://flatiron.com/about-us/], Accessed November 2018
[3] “Survey of physicians shows EHR system market share by vendor,” American College of Physicians, May 18, 2015, [https://www.acponline.org/acp-newsroom/survey-of-physicians-shows-ehr-system-market-share-by-vendor], Accessed November 2018
[4] Helft M, “Can Big Data cure cancer?” Fortune, July 24, 2014, [http://fortune.com/2014/07/24/can-big-data-cure-cancer/], Accessed November 2018
[5] “Clinical trial recruitment with AI,” IBM Watson Health, [https://www.ibm.com/watson-health/learn/clinical-trial-recruitment], Accessed November 2018
[6] “How AI could shape the landscape for oncology,” Pharmaceutical Technology, July 24, 2018, [https://www.pharmaceutical-technology.com/comment/artificial-intelligence-oncology/], Accessed November 2018
[7] “About Us: Technology,” Flatiron Health, [https://flatiron.com/about-us/], Accessed November 2018
[8] “Publications,” Flatiron Health, [https://flatiron.com/publications/], Accessed November 2018
[9] “Roche completes acquisition of Flatiron Health,” Roche Media Release, April 6, 2018, [https://www.roche.com/media/releases/med-cor-2018-04-06.htm], Accessed November 2018
[10] Fry E and Mukherjee S, “Tech’s Next Big Wave: Big Data Meets Biology,” Fortune, March 19, 2018, [http://fortune.com/2018/03/19/big-data-digital-health-tech/], Accessed November 2018
[11] Johnston M, “The Transformation of Healthcare with AI and Machine Learning,” InformationWeek, October 16, 2018, [https://www.informationweek.com/big-data/ai-machine-learning/the-transformation-of-healthcare-with-ai-and-machine-learning/], Accessed November 2018
[12] Hubalek M, et al, “Biological Subtypes of Triple-Negative Breast Cancer,” Breast Care; 12:8-14, February 2017, [https://doi.org/10.1159/000455820], Accessed November 2018
Extremely interesting piece on a fascinating area of healthcare! To your question ‘What are the consequences of one company controlling a technology that has such incredible public health potential?’, it’s my view that the negative consequences largely outweigh the positives. By limiting the potential of Flatiron’s technology to the sole preserves of Roche, the scale of impact is drastically reduced. The main benefit is that Flatiron’s leadership are no longer wasting its time and effort on fundraising and can now focus on delivering on its long-term strategy without the hindrance of financial concerns. Roche will need to demonstrate that Flatiron is being given the autonomy it deserves, to innovate in the best-interest of their patients rather than purely to please investors.
Thank you for sharing!
Thanks for sharing this very interesting post! I’m impressed by the amount of academic publications being generated from OncoEMR data and view this practice as key to its long-term success. Oncology is one of the most evidence-based fields in medicine, so proving the benefit of OncoEMR with rigorous data will be key to increasing physician buy-in. My only concern is that the acquisition by Roche might make doctors trust the platform less. Still, if the OncoEMR continues to be produce promising scientific data, trust should be less of an issue. Overall, I’m excited to follow this technology moving forward and am optimistic it will benefit patients.
Thank you for this piece. The potential impact of machine learning within the healthcare space is inspiring. The price tag that Roche paid for Flatiron certainly corroborates that they too are believers in this technology. I found Flatiron’s hybrid human-machine learning model particularly interesting as it illustrates the role that humans still need to play in the product development and calibration of machine learning algorithms. In the debate of human vs. machine, I am a firm believer that both is the best solution. One question that comes to mind upon reading about the effectiveness of OncoEMR revolves around timing – why isn’t this technology being rolled out more broadly? In regards to the question about the consequences of having a single firm control this type of technology, I would point out that we are still in the very early stages of product innovation so I would expect competing firms to rise up and challenge Flatiron as their offering matures.