Using Machine Learning to Make Better Decisions in the Wind Power Industry
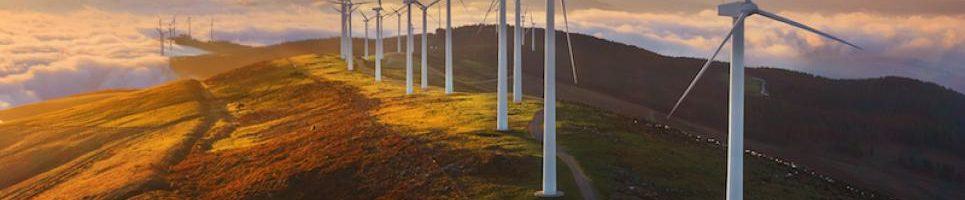
Can machine learning offer an opportunity to reduce the uncertainty in evaluating wind power projects? What is the future of artificial intelligence in the energy industry?
Evaluating wind energy project developments requires an accurate prediction of the energy output of the candidate projects. The most accurate forecasting requires the use of cutting edge technology in climate science and statistical analysis to look into a predicting a highly chaotic weather system with hundreds of variables. The consequence of getting such a prediction wrong is a sub-optimal investment; lower revenues, lower energy output to the grid, and potential reputation damage to an industry that is already viewed skeptically by many investors and other stakeholders.
Predicting weather, and its relationship to renewable energy production is a problem ripe for the application of machine learning. Machine learning seeks to find patterns of predictability in data sets, the problem of climate and energy production already has a huge and chaotic data set (long term climate data, and wind site energy production, acquired by multiple sources at many locations).
Vaisala are a company that seek to turn environmental observations into effective decision making, including wind project analysis. An example of the type of application of machine learning is a wind energy validation study capturing 30 operational wind farms and 143 years of energy production to determine the error between the actual energy output versus the predicted energy output. Applying this model to form an accurate prediction of energy output in the future will reduce the confidence interval and allow for better investment decisions in such projects. [1]
Machine learning and predictive models can help understand wind project risk. Often consultants can give an estimate of the energy production uncertainty, this is done by installing meteorological equipment at a site according to industry norms and ‘rules of thumb’ and acquiring small amounts of local data. These existing methods are associated with greater errors and therefore level of project risk [2]. Vaisala’s Numerical Weather Prediction (NWP) uses current weather conditions to simulate future weather, and to provide proxy data for areas where direct observations are not available. These models can be used to predict wind energy production by tapping into many sets of data, the models are based on complex fluid dynamics and use data from long-term wind observations. Even though such techniques have been used for the last two decades it is machine learning that is pushing the boundary of accuracy and higher spatial and temporal resolution. In the next two years there is scope for extreme learning machines to precisely predict wind speed distribution at different heights that will allow for the most optimally designed wind energy generation equipment [3].
Vaisala, and other machine learning enabled organizations, face shared threats. The first is talent acquisition and retention, a recent poll by EY showed 56% of management see a lack of AI talent as one of their greatest barriers [4], and demand for data scientists and AI specialists vastly outstrips supply. This imbalance will drive up the cost of machine learning talent, and therefore only resource rich organizations will be able to compete in this field [5]. Vaisala should seek to attract talent by augmenting their pitch to potential employees; they not only give data scientists an opportunity to work on complex problems, but their goal is to use machine learning to combat climate change caused by burning fossil fuels. While the labor market catches up and more people seek to study data science at university, Vaisala could seek to up-skill its current employee base and use its in house expertise to train other employees with machine learning skills.
Another issue is bias in machine learning, bias in the data, or the algorithm, will likely be propagated and amplified by artificial intelligence. Vaisala will have to ensure that they implement an appropriate level of risk management to mitigate the effects of bias on their energy forecasting model.
In the next ten years renewable energy will continue to take up a greater proportion of the energy mix, the inherent variability in the energy output from these projects will put additional pressure on those that buy, sell and distribute electricity. A model that can perfectly predict the variability of wind energy production will be helpful in managing the fluctuations in supply and demand. The future is the use of machine learning and artificial intelligence to manage the power grid, such a system would be capable of predicting demand, renewable supply and determining what “flexible” energy sources (e.g. natural gas, large scale storage, hydro) are needed to meet the demand. The value addition through such a system would be decreased waste, maximization of renewable usage and a resultant decrease in costs and CO2 production [6].
How can organizations, private, public and government, collaborate to use machine learning on the worlds largest problems, such as climate change? What are the issues that relate to an artificial intelligence that requires data from competing electricity suppliers and users?
(Word Count 799)
[1] “Balancing Innovation & Stability.” Vaisala, 2017. www.vaisala.com/energy accessed November 2018
[2] Hendrickson, Matthew. “Avoiding Surprises at the Investment Table.” Windpower Engineering & Development, 2015. via www.vaisala.com/energy accessed November 2018
[3] Shamshirband, Shahaboddin; Mohammadi, Kasra; Tong, Chong Wen; Petkovic, Dalibor; Porcu, Emilio; et al. “Application of Extreme Learning Machine for Estimation of Wind Speed Distribution,.” Climate Dynamics; Heidelberg Vol. 46, no. Iss. 5-6, March 2016.
[4] Duffy, Nigel; Mazzei, Chris. “Innovation Matters: Insights on the Latest Disruptive Technologies.” Ernst & Young, https://www.ey.com/gl/en/issues/business-environment/ey-innovation-matters-putting-artificial-intelligence-to-work. accessed November 2018
[5] Daly, Ciarán. “How You Can Bridge The AI Skills Gap in 2018.” AI Business, January 2018. https://aibusiness.com/bridging-ai-skills-gap-2018-long-read/ accessed November 2018
[6] Jones, Jackie. “Balancing Act: How Can We Deal with Variability?” Renewable Energy World, November 2011. https://www.renewableenergyworld.com/articles/print/volume-14/issue-6/solar-energy/balancing-act.html accessed November 2018
Very interesting article! I have worked with this sector in Brazil and I would like to add two potential challenges I foresee for such technology in my country:
1) Incentives to invest innovation: In Brazil, the free electricity market is very small. Most of the electricity is sold in the regulated market and, therefore, are priced under strict rules. Many generators and distributors do not invest in innovation because they are not rewarded by the regulator agencies for that. Actually, there is a risk that an investment in innovation is considered discretionary and the company might be penalized if it plans to transfer the cost of this investment to electricity tariffs. I see more potential of AI investments in private generators, such as Brennand, because they have more resources and incentives to make the change.
2) Information sharing: As you mentioned, the AI system requires a huge volume of data to deliver accurate predictions. As far as I know, wind generation companies protect their predictive models because they are their source of competitive advantage. They do not want other companies to install wind farms close to their areas. Therefore, I believe that data gathering might a challenge and must be treated using an impartial agency/ regulator who can make the best use of the data.
Very informative. Looks like data scientist is going to be a very employable skill for the foreseeable future while we transition from an analog world to a digital one. It’s especially interesting to me, though, how data science is here being used to bring more analog resources online. Based on what you implied, it doesn’t seem very far off before we’ll be using machine-learning trained models to 3D print customized wind turbines for each specific hill on a ridge. That might seem crazy now, but 100 years ago we thought fossil fuels were inexhaustible, so who knows what’s possible! It makes me wonder what the barriers to entry are for a field like this. Maybe we need a Schlumberger for the wind and solar industry.
Great read, Andrew! I remember building a “wind turbine” out of household materials through an outreach program at work–design is no joke. A couple of thoughts on your questions:
1. Climate change is an interesting intersection of first-principles models and statistics. Even though scientists understand the underlying physics of weather, the outcomes are quite sensitive to initial conditions in models, simulations, etc. Data science is immensely helpful in refining current weather models, but I think the one weakness is the difficulty of including long-term macro trends, such as global warming and El Nino/La Nina. For other projects in the public sector, machine learning could supplement years of similar fundamental research to provide an extra layer of refinement.
2. I’m actually quite confident in the integrity of data from electricity suppliers. Given that electricity consumption is critical for utility pricing, I would expect many stakeholders to be keeping a close eye. If there were any cheating or wrongdoing in the consumption data, investors would be calling up their lawyers within the hour!