The Impact of A.I. and Machine Learning on Financial Data at S&P Global
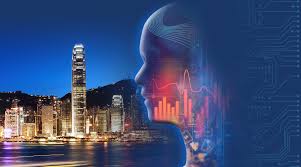
With a massive amount of data now available to financial institutions, S&P Global must determine how to use A.I. and machine learning to meet customers’ evolving demands.
On March 6, 2018, Kensho, became the largest artificial intelligence deal in history [1]. Most people would guess the lucky startup was acquired by a Silicon Valley giant; however, the buyer turned out to be from Wall Street – S&P Global acquired Kensho for $550 million. S&P Global’s acquisition of Kensho exemplifies how A.I. and machine learning are impacting both the internal process improvements and product development strategies in the financial sector.
S&P Global’s strategic shift to focus on artificial intelligence and machine learning
The S&P Global Market Intelligence segment, which includes S&P Capital IQ and SNL, aggregates huge amounts of company financial data. The firm employs thousands of analysts to add 100,000s of new companies to the database every month; to maintain the necessary speed and quality requirements, the company needs to develop more efficient processes using A.I. rather than relying solely on manual analysis [2]. However, automation cannot completely replace human analysis. Instead, as research in the Harvard Business Review demonstrates, if humans and machines work collaboratively the more successful the process improvements in the company will be (See Figure 1) [3].
Furthermore, customers, such as investment banks, are no longer only looking for large amounts of data; rather, they are demanding tools that will provide them insights on data. Institutions recognize that data which integrates machine learning insights, rather than simply data mining, allows for deeper and broader analysis, and thus increases business value [4]. Ultimately, S&P can no longer stick to its historical strategy of providing large amounts of data but rather needs to integrate machine learning into its products and processes to make them more useful and thus create a stickier customer base.
Integrating artificial intelligence is part of S&P Global’s short- and long-term strategy
Recognizing that customer demands are evolving, S&P Global has created an internal team of engineers to develop machine learning capabilities, such as natural language processes, that will complement its existing data products. For example, one quant hedge fund originally signed up for Capital IQ to receive access to PDF files of investor transcripts [5]. Eighteen months later, the quant fund now uses transcripts that integrate machine learning technology to make the transcript interactive. By adding machine learning into its products development roadmap, S&P will attempt to keep up with customer demands.
To accelerate its long-term strategy to have machine learning fully integrated in its data products, S&P has begun to invest in technology through S&P Global Ventures, relationships with venture capital funds, and direct investments, thus explaining the acquisition of Kensho [6]. Kensho will be integrated into the S&P platform, allowing users to ask financial questions in a Google search format and instantly receive answers (See Figure 2) [7].
Additionally, A.I. will augment the data mining process of the S&P platform. For example, S&P has invested in bots that help analysts as they pull data [8]. They are investing heavily to automate as much of the analyst process as possible. While integrating Kensho and the bots will take time, the long-term impact will make the S&P platform more efficient, accurate, and better serving customer needs.
S&P Global must implement additional processes to meet the changes of artificial intelligence in the financial sector
S&P Global must take measures to build trust both internally and with the consumer that the AI technology is accurate. In the 1980s financial institutions attempted to use AI to make financial decisions but these systems almost all failed in comparison to human judgement; while in years since, A.I. has been integrated in certain financial institutions, such as with banks to detect fraud, there has yet to be significant evidence that machine learning can make better financial decisions than humans [9]. However, trust does seem to be slowly returning as a handful of funds are using AI-enabled strategies for trading various asset classes [10].
The only way S&P will garner the necessary confidence is by keeping humans involved in the data collection process. A.I. tools are only as good as the data provided to the algorithms; if humans are not involved in authenticating the data the A.I. tools will lead to false confidence in the results and exaggerate human errors [11].
Ultimately, S&P needs to stick to its original value proposition – quality and trustful data; to continue to do this, the Company must use the assistance of machine learning.
What else should S&P Global consider?
What are some concerns financial organizations should have about implementing machine learning into their analysis?
What specific aspects of financial analysis do you think will benefit most from machine learning? Feel free to draw on personal experiences.
Figure 1: Human-Machine collaboration leads to more successful process improvements
Figure 2: An Example of an answer from Kensho
(Word count: 785)
[1] Antoine Gara. “Wall Street Tech Spree: With Kensho Acquisition S&P Global Makes Largest A.I. Deal In History,” Forbes, March 6, 2018[https://www.forbes.com/sites/antoinegara/2018/03/06/wall-street-tech-spree-with-kensho-acquisition-sp-global-makes-largest-a-i-deal-in-history/#165c780967b8], accessed November 2018.
[2] S&P Global Inc., 2018 Analyst/Investor Day (New York: S&P Global Inc, 2018.
[3] James Wilson and Paul R. Daugherty, “Collaborative Intelligence: Humans and AI Are Joining Forces” Harvard Business Review (July-August 2018).
[4] David He, Michael Guo, Jerry Zhou, and Venessa Guo, Boston Consulting Group, “The Impact of Artificial Intelligence (AI) on the Financial Job Market,” March 2018.
[5] S&P Global Inc., 2018 Analyst/Investor Day.
[6] Ibid.
[7] Antoine Gara. “Wall Street Tech Spree: With Kensho Acquisition S&P Global Makes Largest A.I. Deal In History,” Forbes, March 6, 2018
[8] S&P Global Inc., 2018 Analyst/Investor Day.
[9] Lauren Cohen, Christopher Malloy, and Will Powley, “Aritifical Intelligence and Machine Learning Revolution in Finance: Cogent Labs and the Google Cloud Platform (GCP),” HBS No. 9-218-080 (Boston: Harvard Business School Publishing, 2018).
[10] Ibid.
[11] Michael Schrage, “Is ‘Murder by Machine Learning’ the New ‘Death by PowerPoint’?”” Harvard Business Review (January 2018).
A cool piece on how AI is being used to do financial analysis…so we don’t have to! However, I am curious to know that since the AI algorithm is always contingent upon human operators, and knowing that humans are inherently imperfect, will Kensho go to AI that can identify its own errors and be able to fix them? Particularly errors that a human would not be able to see or foresee.
Great article Danielle! I think you pose interesting questions which I’d like to answer by focusing on investing organizations. Some concerns investing organizations may have about depending on AI for their investment strategies is that once AI becomes mainstream, the strategies generated by different algorithms, and hence different firms, may be similar. This would decrease the returns of any one institution because over the long-run AI would theoretically increase market efficiencies decreasing opportunities for excess returns. A more dire consequence of this dependence on AI is that if all institutions are following similar strategies, it may increase the cyclicality of business cycles as a majority of firms would buy at the same time, leading to an increases in prices, and subsequently sell at the same time, leading to a crash.
I’m not sure if the machine learning is truly going to complement the analysis for the investors seeking alpha (as someone who is coming from the finance/hedge fund background, have to defend it), but I see that some open innovation / technology advances can definitely take over some jobs in finance: trade execution, back office support, risk management analytics, etc. If it’s going to the point of such integration of quant/machine learning, do you think that finance as industry is doomed?
I think machine learning for investors is a really interesting concept. One “concern” or question I have is whether or not we can find ourselves in a precarious situation where certain investors are at massively unfair advantages due to their machine learning capabilities. While investors are not receiving any non-public information about the companies they are investing in, in reality the individual investors are operating with a relative lack of information because of their inability to obtain or process such vast amounts of data. Will machine learning put individual investors at too much of a disadvantage?
I cannot tell you how much mind numbing data sifting one does as a Wall Street analyst! I would love the idea of tools supplied by S&P to make transcripts, financial data and company reports more accessible, data driven, and understandable. I am curious about the application of machine learning tools to investing. That has been a super popular field for a while, but I think investors broadly are just beginning to understand the implications of this. To your point around risks of a completely automated system, I think of something like the 2010 Flash Crash, where a number of automated trading bots malfunctioned and caused a substantial and rapid decline in securities prices, an event which took years to fully understand and disentangle. Great piece!
Great article and I echo Everett! I’m glad to see they’re exploring ways to add more value for insights. Before sending our clients quarterly reports, our performance team would spend weeks cleaning data and ensuring that we were reporting accurately relevant to benchmark. Benchmark data itself for indices was pricey. We’d have to negotiate firm-wide access every time we introduced a new benchmark for an assets performance – and it seemed ripe for disruption given the prices! Given the efficiencies of scale providers like S&P have, it makes sense that they’d take on more of the insight role, instead of every bank individually performing the same analysis over and over again!