The future in cement manufacturing is here: Transforming Cementos Molins’ production process through the application of AI
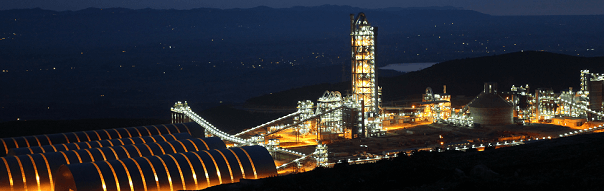
2017 was a landmark year for Cementos Molins, a leader in the European cement manufacturing industry, not only because it sold a record 6 million tonnes of cement, generating an EBITDA of $218 million [1] but also because it revolutionized its highly traditional operations through the application of artificial intelligence.
The cement production process
Cement manufacturing is a process consisted of two major steps with generally similar cycle times: the Mill, which grinds hard clinker to fine powder, and the Kiln, where this powder is heated at 1500⁰C to decarbonize the material [2].
The performance of the process –measured through metrics such as energy consumption, CO2 emissions, output quality, etc.– is depended on thousands of variables (e.g. humidity, vibration, external and internal temperature) which are continuously monitored. To optimize the plant’s performance, staff manually adjusts ~50-100 of these variables over regular time intervals (every ~10 minutes).
The relationship between these variables and the process’s performance, however, is highly complex and employees can only depend on their experience, rather than a predefined playbook, in order to deliver their job. Expectedly, this optimization process is very prone to human error, causing frequent blocking/starving, downtime, high energy consumption and thus large costs to the company.
To respond to these challenges, a growing number of plant manufacturers are applying artificial intelligence in order to both eliminate human error and maximize the variables’ adjustment frequency (from ~10 minutes to seconds). In the case of Cementos Molins, the management decided to develop a real-time self-repairing process, with the objective of maximizing production rate while keeping energy consumption to the minimum and product quality to the maximum.
Appling Artificial Intelligence in a cement plant
Given the company’s lack of experience, the management decided to partner, in the short term, with an external startup specialized in the real-time improvement of industrial processes through the application of Artificial Intelligence, Optimitive. Optimitive’s role in this stage was dual:
- To develop and apply the optimization algorithm to Cementos Molins’ factory in Barcelona, which resulted to the increase of the production rate by 3.8%, the reduction of the energy consumption by 5.5% and the improvement of the output’s quality by 3.1% in the first 6 months [3].
- To train a team of Cementos Molins’ management and factory staff on the development philosophy, application and use of the software. This would enable Cementos Molins’ to oversee the process and, in the longer term, expand the application of the algorithm to other factories.
Going forward, over the medium term, Cementos Molins’ plans to develop an internal team consisted of the trained employees as well as newly hired data scientists. This team will allow the company to continuously improve the algorithm, roll it out to other plants and train local staff whilst avoiding the high consulting fees of external partners.
Looking ahead – How to further leverage Artificial Intelligence
Cementos Molins has done a great start, entering the new digital era of cement manufacturing way ahead of competition. However, this is just the start of the journey. There are many more actions that the company could implement to enhance its operations and its operating margins, with two major ones being:
- Investing in a cloud service: This would allow information sharing between different factories; the algorithms applied to different plants would be able to learn from each other and further improve their performance [4].
- Adding a self-adapting functionality: The cement industry is highly correlated to the local economic environment whilst demand for different types of cement also fluctuates seasonally [2]. Thus, adding a self-adapting functionality would be highly beneficial for the company since it could customize its manufacturing process and the type of cement it produces in response to demand fluctuations [5].
Challenges to address
Great changes are often coupled with great challenges. In my opinion, in the case of Cementos Molins the two most important points that the organization should address are:
Data homogeneity: Since the company’s ability to store data is finite, eventually the vast majority of the data stored will have been generated by the algorithm. Thus, the variability of the dataset will be limited and there will be no room for the algorithm to discover new combinations. This, in turn, means that the algorithm will not be able to further improve its performance, even though this may be feasible in theory. How could the company avoid this situation?
Ensure employee’ experience gaining: Employees must oversee the algorithm’s operation and occasionally to take control. However, since they will not actively participate in the process for the majority of the time, the experience they will gain will be limited and thus they may not be properly equipped to take control. How can the organization ensure that, in parallel to running the algorithm, the frontline of the factory will develop?
(798 words)
References:
[1] Cementos Molins. Annual Report 2017. (2017). Available at: https://www.cemolins.es/uploads/media/B1-Listas/043-Informaci%C3%B3n-financiera_Memorias-anuales/Memorias_Cementos_Molins_ENG/CM2017(Financiera)_ENG_LR.pdf (Accessed: 10 November 2018).
[2] Globalcement.com. CEMENT 101 – An introduction to the World’s most important building material. (2011). Available at: http://www.globalcement.com/magazine/articles/490-cement-101-an-introduction-to-the-worlds-most-important-building-material (Accessed: 9 November 2018).
[3] Optimitive. Optimitive saves 5.5% energy and improves productivity and quality in Cementos Molins Industrial. (2017). Available at: http://www.optimitive.com/en/optimitive-news/233-optimitive-saves-5-5-energy-and-improves-productivity-and-quality-in-cementos-molins-industrial (Accessed: 10 November 2018).
[4] Brynjolfsson and A. McAfee. What’s driving the machine learning explosion? Harvard Business Review Digital Articles (July 18, 2017).
[5] J. Wilson, A. Alter, and S. Sachdev. Business processes are learning to hack themselves. Harvard Business Review Digital Articles (June 27, 2016).
Picture Source:
Industryabout.com. (2017) Sinoma to build US$500m cement plant in Uganda. Available at: https://www.industryabout.com/industrial-news/783-news-cement/40423-sinoma-to-build-us-500m-cement-plant-in-uganda (Accessed: 13 November 2018).
They say that all industries are becoming technology industries, and it makes sense that cement manufacturing would be no exception to this trend. Automation generally enables companies to employ fewer workers — or at least to shift human labor to different kinds of roles. Here we see a shift toward employing data scientists to monitor production algorithms. Automation is a well established, unavoidable trend, but its effects can be painful for highly experienced workers whose expertise is suddenly no longer valued. I would be interested to know whether this labor shift is apparent in Cementos Molin’s operations and how its employees feel are reacting to this production automation.
With the learnings that Cementos gains would it be possible to further eliminate the need for human interaction with the process, thereby reducing human error? I’m no expert in cement, but I do wonder if through the use of scanners and technological advancements in machinery if the company could get even more learnings to better advance the algorithm. Also to address the homogeneity perhaps the company can have an innovation lab that is conducting tests to come up with new alternatives?
Machine learning can not only improve production process, but can also assist production and inventory planning. Building up such capability requires gathering data from the demand side. For example, what is demand in each plant correlated to? Any other factors (such as age of local buildings, population growth) that could affect order quantity? Machine learning could use these data to predict the demand for the next quarter and hence improve cash flow generation of the business.
I saw a similar example of partnership in the chemical industry, where machine learning algorithm helped to control process parameters.
However, for me the open question here is the motivation of startup in work with the manufacturer. The manufacturer takes control over the results of the project and the potential for scaling is not really high. For instance, development of predictive maintenance model is often a separate project for every piece of equipment working in particular circumstances.