Teladoc about Machine Learning
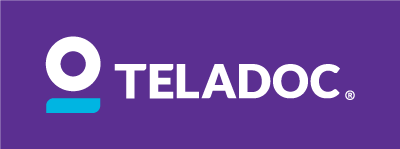
Telehealth has recently emerged as a convenient alternative to the traditional in-person health care appointment. Teladoc, the oldest and largest telehealth company, provides 24/7 access to physicians worldwide via audio or video consultations. Patients use the Teladoc website or mobile app platform to complete a medical questionnaire, speak directly with a board-certified physician, and obtain prescriptions [1]. Machine learning has brought a paradigm shift to the telehealth care process, powered by the availability of health care data and rapid advancement of algorithm technologies. Telehealth companies have turned to machine learning for assistance in three major processes: diagnosing patients, improving treatment decisions, and solving logistical challenges [2]. By integrating synergies between machine learning and telehealth, Teladoc can remain competitive in delivering the most efficient, highest quality care to patients.
Teladoc currently models a traditional appointment by connecting patients with a single physician or therapist. However, diagnostic errors remain inevitable when relying on the knowledge of one health care professional. Studies suggest that physician diagnoses are incorrect 10-15% of the time [3]. Machine learning has significant potential in diagnostic support; AI can use sophisticated algorithms to “learn” from a large database of medical data, and compare patient symptoms to that database to suggest possible diagnoses. AI engines in the form of “chatbots” can now remotely lead patients through a more comprehensive set of questions compared to those asked by physicians, to increase the probability of reaching the correct diagnosis [2].
AI algorithms can also recommend treatments through training from a database of existing treatments and success rates. Physicians face the great challenge of remaining up-to-date with new medical literature to deliver the most current interventions. One study estimated that it would take a physician 29 hours per day to review all up-to-date primary care literature, an impossible task [4]. AI can assist physicians by providing relevant medical information from journals and textbooks to inform patient diagnosis and treatment [5]. InfiniteMD, a company marketed as a “second opinion” for patients treated by a specialist, uses an AI engine to support the consultation process. The company states that “28% of our cases result in a change or correction in diagnosis and 72% of our cases result in a revised treatment plan,” providing evidence for a future role for AI-assisted diagnosis and treatment [6].
Machine learning platforms also have the potential to solve logistical challenges that Teladoc will inevitably face as the company scales. Teladoc treats general health conditions, and has recently expanded to include a limited set of specialty services including therapy, dermatology, sexual health and tobacco cessation [1]. One major competitor to Teladoc, Lemonaid Health, collects patient information through a chatbot and matches patients with a physician best suited to address their needs [2]. As Teladoc expands to offer more specialty services, the company must consider the challenge of information asymmetry—often, patients are unaware which specialty can best treat their symptoms, and rely on primary care physicians to make this decision. The company could incorporate algorithms to rapidly connect patients with physicians who historically have the best outcomes for the patients’ symptoms.
As telehealth companies trend toward AI assistance in diagnosis, treatment, and logistics, Teladoc must take steps in the short term to optimize its care process in order to remain competitive on quality and efficiency. Teladoc responded to these challenges through acquisition of specialty consultation platform Best Doctors in the summer of 2017. The acquisition included Oncology Insight, an IBM Watson-powered second opinion service for oncology experts. As Teladoc continues to incorporate machine learning platforms to guide physician decision-making, the company should prioritize quality control through careful selection of datasets and partnerships. Continued physician involvement in the integration of cognitive computing services will remain crucial at this stage, as AI-assistance remains far from perfect. With a strong foundation in medical quality, the company can then focus long term on the expansion of machine learning to appropriate specialties, and even work towards reducing physician involvement to minimize human error. By focusing on both short- and long-term applications of machine learning, Teladoc can continue to deliver on its mission to provide health care with “greater convenience, outcomes and value” [1].
Question
Teladoc was founded to help patients overcome barriers to accessing care by connecting patients with physicians. However, the rapid advancement of algorithms in health care has brought to light new approaches to addressing the access issue. AI-assisted symptom checkers have emerged that aim to reduce physician involvement in the care process, representing a shift toward democratization of health care delivery. Can Teladoc still provide value by connecting patients with physicians through telecommunications if machine learning outcomes reliably exceed physician outcomes?
(Word Count: 773)
References
[1] The quality care you need with the convenience you want. Teladoc. <https://www.teladoc.com/> Accessed 11/10/18.
[2] Artificial intelligence in telemedicine and telehealth – four current applications. TechEmergence. <https://www.techemergence.com/artificial-intelligence-in-telemedicine-and-telehealth/>. Accessed 11/10/18.
[3] Graber, Mark. The incidence of diagnostic error in medicine. BMJ Qual Saf 2013;22:ii21-ii27
[4] Alper, Brian. How much effort is needed to keep up with the literature relevant for primary care. J Med Libr Assoc. 2004 Oct; 92(4): 429–437.
[5] Jiang F, Jiang Y, Zhi H, et al. Artificial intelligence in healthcare: past, present and future. Stroke and Vascular Neurology 2017;2.
[6] Consult a Top Specialist. InfiniteMD. <https://infinitemd.com/patients/>. Accessed 11/10/18.
Very interesting to understand the advancements going on in healthcare. I think the question raised is the right one. My perspective, is given the emotional nature behind personal or family illness, I’d suspect in the short to medium term individuals will still want a physician to be involved to opine on the computer generated response. That said, if technology is able to evolve to very high machine generated accuracy, I could see an environment where demand for physicians declines. Teladoc, however, will still be positioned strongly to capitalize on this change in market dynamics given the wide public acceptance and trust of Teladoc’s history.
I too agree with the question being asked, though I would stress that for the foreseeable future, this ought to be treated mainly as another resource (albeit an important one) provided to physicians to enable them to make more accurate decisions, i.e. a diagnosis. The reason why I am skeptical to replace doctors with Teladoc, even despite the possibility that Teladoc may be more accurate, is two-fold. First, physicians will always be at least as accurate as Teladoc because they will use the AI to help them make a diagnosis (the overriding assumption here is that since decision-making is still in the hands of the physician, if the physician decides not to concur with the AI, it is because the AI made the wrong diagnosis). Second, physicians do more than just diagnose and treat. They also help provide guidance on daily management of a condition and more importantly, can provide a patient comfort via human-to-human interaction. Therefore, while I think a trend towards more diagnoses from AI is beneficial, the counterweight should be in conjunction with a physician to provide more complete care to a patient beyond just a diagnosis.
In my opinion, connecting patients with physicians is an important step in diagnosis of a problem. Machine learning can only go so far. In medical science, it’s not just diagnosis of the problem that could be the end of a user journey – it’s just the start. The human judgement that a physician brings to the table can never be replaced by a machine. Moreover, thinking about village areas in developing countries, services like Teladoc can be very useful where physician availability is almost zero and people are still relying on their age-old methods of treatment.
On the commercial side, an app with just machine learning algorithm ( similar to Teladoc, but not involving any human interaction) could be programmed to suggest the most expensive medicine on the market when there might be cheaper options available – which an average user might not be aware of.
I agree that machine learning powered apps/technology should assist the doctors or physicians but should never replace them.