Roche: Machine Learning Brings a Big Pharma Business Model Under Siege
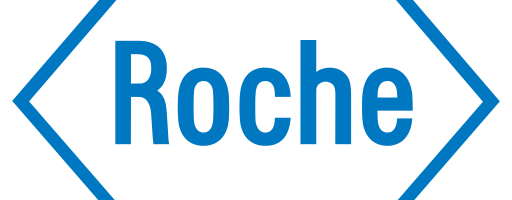
Roche, the Swiss pharmaceuticals giant, is caught in a race against time. After making a big bet on breakthrough drugs and personalized medicine, the company has spent much of the last 18 months thinking ahead to the challenges that machine learning and artificial intelligence pose to a traditional, integrated pharmaceutical business model.
The traditional business model in pharma hinges on blockbuster drug development, large-scale clinical trials and a robust market access and marketing engine. But the drug-hunting approach of old—with a cycle time of a decade and a price tag of $2.7 billion and growing—is unsustainable for Roche and its fellow pharmaceutical giants. [1] For Roche in particular, a biologics “patent cliff” looms over its portfolio of cancer drugs, which generated $21 billion in sales last year but will face competition from cheaper biosimilar copies by the end of this year. [2]
Roche’s traditional competitive advantages across each stage of the chain—1) Discovery & Development, 2) Clinical Testing, and 3) Go-to-Market — are being threatened by upstarts across technology and health care armed with new sources of data and machine learning techniques.
- Machine Learning in Drug Discovery: Advances in technology and the introduction of new types of gene and cell therapies have given rise to more and better-funded early-stage biotechs. These biotechs are taking advantage of i) declining cost of genomics, ii) huge volumes of new patient data, and iii) applications of machine learning techniques to computational biology. Machine learning startups such as BenevolentAI claim to be upending and outpace traditional R&D models in pharma with target validation success rates that are 4 times as high. [3]
- Digital Biomarkers in Clinical Testing: As the number of high-potential drug targets grows, the bottleneck for pharmaceutical companies will increasingly become clinical trials. Today, trials are expensive affairs with big logistical challenges (30% of Phase 3 trials are terminated due to enrollment difficulties). [4] Yet companies like Roche will face competition from technology giants that are better positioned to collect digital biomarkers from large samples through virtual, “siteless” trials (e.g., via Apple Watch or Alphabet’s Project Baseline) and apply machine learning techniques to improve clinical trial design and efficacy.
- Go-to-Market and Revenue Models: With physicians becoming more engaged in designing custom therapeutic interventions that move beyond pharmacology to include behavioral and digital approaches, the results from the clinical trial won’t have the last word—pharmaceutical companies will be challenged on outcomes and prices by providers, insurance companies, and governments. Providers and payers will look to apply machine learning techniques to a wealth of patient data when determining whether and how to prescribe and pay for drugs.
In the last 18 months, Roche has responded to these challenges to its business model through i) a strategy of partnerships with drug discovery companies, ii) acquisitions of genomic profiling companies that will help it personalize cancer treatments, and iii) a focus on clinical decision support tools that combine in vitro diagnostics with other patient data to give Roche leverage with providers. These tactics—all fundamentally bets on the value of machine learning techniques—are designed to address the emerging threats to Roche’s business model. In turn:
- Drug Discovery: To strengthen its target pipeline and better position itself against new drug discovery startups that use machine learning, Roche entered a strategic partnership with GNS Healthcare, a company that uses ML techniques to help identify new drug candidates. [5]
- Acceleration of Clinical Trials: In July 2018, Roche acquired Foundation Medicine, a genomic testing and profiling company that will help Roche a) better identify which patients are suited for clinical trials for its drug candidates, and b) expand testing to more potential candidates for treatment through the development of liquid biopsies. [6] This comes on top of its February acquisition of Flatiron Health, an oncology data startup that could give Roche sufficient real-world evidence to have to forgo control groups entirely—a development that would help Roche defend itself against technology companies seeking to introduce “virtual” clinical trials. [7]
- Go-to-Market and Revenue Models: With payers applying increasing pricing pressure and providers recommending more personalized treatments, Roche needs to protect is value proposition. To do so, Roche entered a long-term partnership with GE Healthcare to build clinical decision support tools for physicians that use machine learning and *combine* genomics, biomarker, and monitoring data—getting Roche directly in front of the physician when it comes time for them to recommend treatments for patients. [8]
Yet partnerships are not a panacea. To succeed, Roche needs to rethink the role of a pharma giant in the digital age. When ML startups can develop assets faster, tech giants can run bigger clinical trials more precisely, and providers and patients can customize holistic treatments, Roche’s capabilities all fall under competitive threat. Instead of betting that it will allocate capital across drug discovery, testing, and distribution more efficiently than its competitors, Roche will need to reposition itself and focus on the segment of the value chain that it can best defend.
What *is* the best, most defensible territory for old guard pharma in an age of AI drug discovery startups, patients empowered with digital health solutions, and a global health care system straining to pay for expensive, breakthrough drugs?
(799 Words)
[1] Joseph A. DiMasi, Henry G. Grabowski, and Ronald W.Hansen, Innovation in the pharmaceutical industry: New estimates of R&D costs. Journal of Health Economics, May 2016.
[2] John Miller and Ben Hirschler, Roche steps up efficiency drive to take sting out of biosimilars. Reuters, September 13, 2018.
[3] Biotechnology Report: Beyond Borders. Ernst & Young, 2017.
[4] Patients Recruitment Forecast in Clinical Trials. Cognizant, August 2015.
[5] GNS Healthcare Announces Collaboration to Power Cancer Drug Development with REFS™ Causal Machine Learning and Simulation AI Platform. GNS Healthcare Press Release, June 2017.
[6] Roche and Foundation Medicine reach definitive merger agreement to accelerate broad availability of comprehensive genomic profiling in oncology. Foundation Medicine Press Release, June 2018.
[7] Lydia Ramsey, Why Roche Acquired Foundation Medicine and Flatiron Health. Business Insider, August 2018.
[8] Roche and GE enter partnership to develop integrated digital diagnostics platform to improve oncology and critical care treatment. Roche Press Release, January 2018.
Thank you for this interesting article! Raises a very relevant question around future of pharma companies in light of the digital technology and advances made to reduce the time and $ investment in the product development. Particularly, raises questions around how barriers to entry are changing in the pharma industry. Roche has been a leader for several decades, but how will that change if startups and other competitors can also emulate the same machine learning strategy that Roche is currently deploying. Will be interesting to understand what the competitive difference will be then, if machine learning costs are substantially lower than current tools/systems for clinical trials?
First, the purported price tag of $2.7 billion for drug development is based on research that is not sound. With that number, they factored in the opportunity cost of investing funds into R&D, essentially using it to double their figure. Additionally, the study was funded by pharmaceutical companies, creating clear conflicts of interest. However, that aside, much can be done to improve drug development and Roche’s efforts are a step in the right direction. I think machine learning will be particularly useful in drug discovery, more so than clinical testing and go to market strategy but is an interesting concept to explore regardless.
Definitely an interesting combination of acquisitions in Flatiron and Foundation which will allow them to support more efficient clinical trials using segmented patient populations. By segmenting patient populations based on key genomic and phenotypic biomarkers, Roche can target those individuals who would benefit from a particular therapy, from those that wouldn’t. A classic example of patient segmentation based on a biomarker is for Herceptin (Genentech), which is used to treat breast cancer. Before treatment, patients are tested for the overexpression of HER2 in a breast cancer biopsy. Patients that don’t demonstrate overexpression of HER2 will not benefit from treatment, and treatment would only expose them to potential side effects. By utilizing key biomarker endpoints, Roche can only help themselves run leaner, more cost effective trials, and increase their chances for approval by only treating those patients where a response is anticipated.
Very interesting topic! One way I see the incumbents in the industry attempting to maintain their competitive position and improve the efficiency and effectiveness of their R&D spend is to supplement their internal R&D with structured external R&D programs. For instance, Johnson & Johnson has a program called JLabs that was established to develop an incubation model for J&J’s biopharmaceutical business [1]. Others in the industry have adopted this approach as well. “The goal of these efforts is to access the vast pools of innovation outside the walls of the in-house R&D organization and tap into the entrepreneurial risk-taking culture of biotech to secure a future pipeline, while avoiding the well-appreciated and suffocating burden of larger bureaucracies on creative research.[2]” This approach allows large pharmaceuticals companies to rely on small, agile partners for innovation and they can focus their efforts on commercialization of promising drugs that result. I believe they can leverage this approach with their ability to manufacturer large quantities of product in a highly regulated industry and their network of physicians to sustain their position in the value chain. They’ll need to continue to adapt, as Roche is doing, to ensure they maintain their role.
Source:
[1] “About Us.” JNJ Innovation, 19 Oct. 2018, http://www.jnjinnovation.com/about-us#par-44.
[2] Booth, Bruce. “Positive Impact Of External Sourcing On Pharma R&D Productivity.” Forbes, Forbes Magazine, 16 Dec. 2016, http://www.forbes.com/sites/brucebooth/2016/12/16/positive-impact-of-external-sourcing-on-pharma-rd-productivity/#122d8a613863.
This is a fascinating application of machine learning in the healthcare industry. I agree – there could be a variety of applications surrounding the entire process. I’d be curious to see how it could be applied to high value drugs with a long development cycle to further minimize costs. It seems to create value down the entire value chain from the R&D state all the way until drug development.