Promise and Peril for Machine Learning at Netflix

As the amount of content, competition, and subscribers in the online media space grows, Netflix is turning to machine learning to provide a more entertaining experience for its customers. While they are investing heavily in their proprietary recommendation engine and enjoying the benefits of increased customer retention, questions are emerging around how far into customer profiling a machine learning algorithm should ideally go.
Business Importance of Machine Learning at Netflix:
Since its founding as a mail-based DVD rental business, Netflix has offered subscribers an extensive array of movies and television shows to choose from. Now with 117 million streaming memberships that watch a combined 140 million hours daily, Netflix has increasingly been branching into content creation, including hit series such as Stranger Things and Orange Is The New Black [1]. With growing scale across more and more diverse customer segments and geographies, the challenge for Netflix of offering highly engaging entertainment to each customer has become increasingly difficult – this is where Netflix has turned to machine learning to ensure that customers are finding the best content on their platform, both in terms of Netflix originals and licensed third-party content.
Nearly 40% of respondents view Netflix as the service with the best original programming
Management Action Plan:
As Hunt and Gomez-Uribe remarked, the content recommendation feature is an incredibly powerful tool in retaining customers. Back in 2013, Netflix saw their recommendations driving over 75% of all content viewing, compared against consumer search [4].
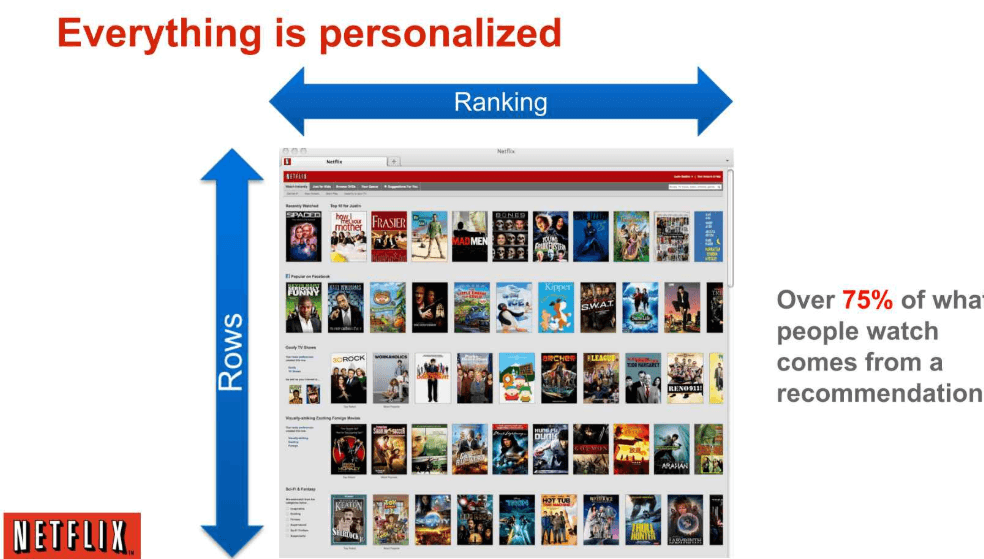
As far as aligning corporate investment with the platform, Netflix in 2014 dedicated a team of 300 employees and spent $150M (around 3% of 2014 revenues) to improve their recommendation engine – this dollar figure has likely continued to grow as the amount of content and subscribers has increased [5].
The newest investment that it seems Netflix is making to create a more customized and tailored platform experience is in connection with the artwork, or the thumbnails that subscribers see when browsing TV show and movie titles [6]. For example, there are nine different artworks for the Stranger Things show that users may see when browsing, depending on whether they have historically shown more interest in comedy, horror, or suspense in their viewing histories. However, this is in particular an area where Netflix should treat with caution, since there are downside risks of profiling and offending customers in a visually obvious way.
Several Recommendations for Netflix:
In 2009, Netflix issued the Netflix Prize challenge to “substantially improve the accuracy of predictions about how much someone is going to enjoy a movie based on their movie preferences,” with a grand prize of $1M [7]. However, it seems that Netflix has not held any similar competitions since 2009. While Netflix’s internal technical talent has grown tremendously over the past decade, the company should continue this legacy of open innovation to tap into the creativity and ingenuity that resides externally.
Additionally, while Netflix does have a large budget and team focused on the applications of machine learning to their product offering, I worry about the relatively narrow view of online activity that Netflix currently has for its customers. Without violating data privacy restrictions, I would encourage Netflix to partner with other non-competitive platforms (e.g., Facebook, Yelp, etc.) to allow their machine learning content recommendation engine to draw from a more complete view of their subscribers when recommending content.
Open questions:
- Positive feedback loops: Any tool trained through machine learning will tend to exhibit a positive feedback loop. For example, videos with which many subscribers engage will become highly recommended to other members, and then the cycle perpetuates. Is this a bias that should try to be adjusted for, or is this actually a beneficial bias that leads to shared viewing experiences within the subscriber community?
- Risk of racial bias: Just as Facebook has shown the ability to diagnose a user’s age, race, and income from their activity, Netflix has enough of a glimpse into their subscriber’s viewing history and other activity to do the same [8]. With this information, Netflix has appeared to target subscribers with content based on race, socioeconomic status, etc. – is this something that should be criticized or encouraged? (730 words)
Sources:
[1] Netflix, “2018 Amended Annual Report”, https://www.netflixinvestor.com/financials/sec-filings/sec-filings-details/default.aspx?FilingId=12516727, February 5, 2018. Accessed November 2018.
[2] “New research shows Netflix’s big bet on the future is working, as it continues to outpace its competition”, https://www.businessinsider.com/netflix-original-tv-programming-analysis-from-morgan-stanley-2018-5, May 8, 2018. Accessed November 2018.
[3] Gomez-Uribe, Carlos A., and Neil Hunt, “The Netflix Recommender System: Algorithms, Business Value, and Innovation”, ACM Transactions on Management Information Systems (TMIS), https://dl.acm.org/citation.cfm?id=2843948, January 2016. Accessed November 2018.
[4] Amatriain, Xavier, “Machine Learning & Recommender Systems at Netflix Scale”, https://www.infoq.com/presentations/machine-learning-netflix. November 2013. Accessed November 2018.
[5] Roettgers, Janko, “Netflix spends $150 million on content recommendations every year, Gigaom. https://gigaom.com/2014/10/09/netflix-spends-150-million-on-content-recommendations-every-year/. October 2014. Accessed November 2018.
[6] Wilson, Mark, “Netflix Is Even Personalizing Its Graphic Design To You Now”, Fast Company. https://www.fastcompany.com/90154608/netflix-is-even-personalizing-its-graphic-design-to-you-now, December 18, 2017. Accessed November 2018.
[7] Netflix, “Netflix Prize”, https://www.netflixprize.com/, Accessed November 2018.
[8] Tiku, Natasha, “Why Netflix Features Black Actors in Promos to Black Users”, Wired. https://www.wired.com/story/why-netflix-features-black-actors-promos-to-black-users/, October 24, 2018. Accessed November 2018.
I think you’ve made a very salient point that in our hyper competitive world of video streaming, it is extremely key for Netflix to leverage ML to create a differentiated recommendation engine. I’m interested to learn how the competitors you mentioned are tackling this problem and if this differs greatly from Netflix’s ML approach. I also found it very interesting that Netflix used to host the “Netflix Prize challenge” but has not done so in recent years. I agree that it is important for Netflix to keep innovating on this front not just in terms of improving their algorithms, but also in terms of including more training data/sources and incorporating more user feedback. To address your second open question on potential biases, I think it is important that Netflix increases the transparency to users around how their data is being used and how the recommendation engine uses that data (e.g. giving users the opportunity to understand some simple logic behind predictions which could also help to build trust between users and the engine). Additionally, Netflix should also give users some sort of option to give feedback to the engine (maybe with a simple button interface) about whether they found the predictions to useful.
Great post! I think that it’s really interesting to look at Netflix because they are on the more mature end of the machine learning spectrum. Rather than just starting to use it, they are moving into the land of how much is too much and how far is too far, questions you raised in your post. I do become concerned when Netflix uses a very limited data set when providing recommendations. I think that when and where possible (understanding privacy laws), it would be valuable for Netflix to understand more about their customer holistically to provide better and more appropriate recommendations. Of course, they do need to be careful because again as you pointed out, it can turn into profiling in a dangerous way.
I echo the privacy concerns mentioned above. I would respond to your comment about the 2009 competition and the need for more crowdsourcing of algorithm development. While open source frameworks is a really good tool for innovation, in the case of Netflix, two researchers were able to uniquely identify some of the users within the dataset that Netflix released: https://money.cnn.com/galleries/2010/technology/1012/gallery.5_data_breaches/index.html . Thus, even with their attempts to make the data non-attributable, it is clear that in the space of machine learning, privacy can be harder to reacher than just merely staying within privacy laws.
Great read! I think Netflix’s algorithm on how to recommend content is probably one of the most advanced out there, since there is so much subjectivity involved in how someone will appreciate something that is basically an art form such as movies and tv shows. This, combined with the enormous catalog that they have on Netflix makes it a very big challenge. You mentioned how it saves them a lot of money by reducing the churn rate. Do you think there are any other benefits that Netflix can extract from this? Maybe like shorter term licensing contracts for content that is not theirs, dropping content that they predict won’t be appreciated by their current an potential subscriber base. Or, using the data to develop original content themselves based on the type of audience they try to target.
Enjoyed reading this, it’s always good to learn more about something like Netflix that I spend more time than I’d like to admit using! As for your questions on positive bias and potential racial bias, I definitely I think it could be a problem. It would be interesting to see how recommendations differed by state or country to see if there are any trends in the recommendations. Also, there is a bit of a “build on your winners” type of strategy going on here that probably does play a role in helping the company differentiate even its own offerings to customers. There is so much new content – both original and licensed – dropping almost daily at Netflix that the recommendation tool helps guide viewership, but it does make me around whether the company pushes shows they invest more heavily in than those that are more low-budget as well. It’s sort of like other social media where one can live in a bit of a silo and the machine learning at Netflix may do nothing to push the boundaries of what an individual consumes.
Stefan — this is a fantastic article, and thank you for posing such thoughtful questions. While I think the positive feedback loop can be really beneficial for Netflix, I also believe that Netflix should work to cut through the biases in creative ways. If Netflix is not offering enough new themes to a viewer, then the viewer is losing out on watching things in a different category that they may love. There is huge value in shared viewing experiences, but I think that viewers want to also have shared experiences around content that they may not have chosen on their own. So, perhaps Netflix could continue this positive feedback loop while also sprinkling in content that is unrelated to what they have been watching, but could prove to introduce the viewer to a new category they may love. In terms of your stated risks of racial bias, I do think that this is something that could be very dangerous. There are ways for Netflix to know and collect this information, while also ensuring that their algorithms aren’t making suggestions solely based on that information. In fact, perhaps they could use that data to actually suggest content that is typically less viewed “within that race, etc.” as a way to continue to introduce new content to different people. Again, this was awesome. Thanks!
Great thoughts! I tend to lean towards more personalization, as I believe it provides the best customer experience and can truly drive customer retention. The use of consumer’s time is a resource, and I think that any way Netflix can cut down on the amount of time searching for content will drive business into the future. My current frustration with Netflix is how long I search before I find something that I would like to watch! I think it would be a good idea for Netflix to start supplementing its own data with other stats from social media sites. If Netflix could target me so effectively that the first recommendation was an immediate hit for my preferences, I would be one happy consumer.
It is very interesting how through machine learning companies can guide our content consumption. As you mentioned, through positive feedback loop, it seems that they are perpetuating our historical viewership preference. While this is great because it ensures that viewers are happy, how do you incorporate new content or exploratory genres? This would essentially guide viewers to areas of interest that are currently not exploited. While there is a risk to such strategy as Netflix could push negative content, it could also provide an area for increased utilization for the platform. The benefit to Netflix would be that it would alleviate the pressure of having to generate new content constantly. Customers would therefore have a lot of new content that they have not previously explored. Great job.
Thanks for the article — this is very interesting, and I learned a lot about Netflix! One question I am left with is whether the AI you point to can help solve the “always on” problem. What I mean is that regular TV still beats OTT solutions like Netflix for some customers because it is easy to turn on and just watch TV. There is the notion that more choice can create unhappiness. I wonder if the algorithms can help this by knowing what I like and then restricting my choices within it, ultimately just “playing” options for me a la a real TV channel.