Pfizer and IBM: A Collaboration to Accelerate Drug Discovery?
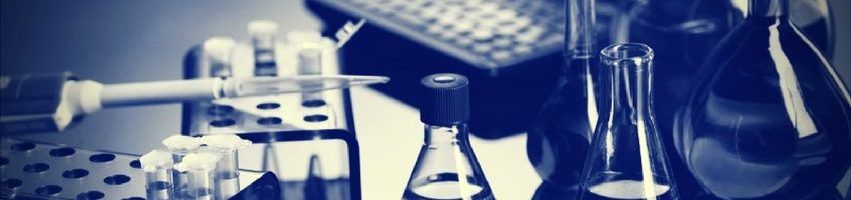
In December 2016, pharmaceutical company Pfizer Inc. announced a partnership with IBM Watson Health to use IBM Watson for Drug Discovery’s machine learning platform to help Pfizer’s research in immuno-oncology. [1] Pfizer sought to use Watson’s machine learning and natural language processing to better identify new drug targets and more efficiently select patients for clinical trials.
Machine learning is important to Pfizer’s product development because of existing inefficiencies in the drug discovery and development process. In order for a pharmaceutical drug to be approved by the FDA, it has to go sequentially through the following processes: target validation and lead selection, pre-clinical testing, phase I, phase II, phase III, and a final submission process. On average, for every 24 drug candidates that enter the discovery pipeline, only one will end up becoming FDA approved. [2] The time to bring a new drug to market takes about 14 years and costs over $1.5 billion on average, with about 40% of the costs coming during initial lead selection. [3] It is therefore crucial for industry leaders to explore more efficient methods of approaching this development process, and machine learning has emerged as a potential solution. Other close competitors in the pharmaceutical industry have already acquired, merged with, or partnered with AI firms in an effort to gain an edge on drug discovery. For instance, Roche partnered with GNS Healthcare using machine learning to convert cancer patient data into models that can identify new targets for cancer therapy. Novartis has also partnered with IBM Watson Health targeting breast cancer drugs. [4]
Watson for Drug Discovery serves as a cloud-based platform with 25 million Medline abstracts, over one million full-text medical journal articles, and four million patents. This amount of data far exceeds what any one research scientist can read in a year, which is closer to 200 to 300 articles. [4] In the short term, Pfizer is hoping that Watson for Drug Discovery will be able to help identify relationships among genes, drugs, and diseases that may not be apparent to researchers working in silos. The machine learning component may be able to sift through massive knowledge databases and reveal correlations between certain drug characteristics and disease states that may provide potential for further preclinical and clinical studies. [5] In the medium term, Pfizer will have to test the effectiveness of Watson for Drug Discovery to better quantify the sensitivity and specificity of applying this technology to pharmaceutical development. Pfizer will likely look at how many successful drug candidates Watson for Drug Discovery is actually able to produce and weigh this benefit against the costs. However, Pfizer will not be able to rely solely on its new machine learning platform to solve its pipeline issues; to achieve maximum capacity, Pfizer will need to integrate Watson into its existing structure and continue to explore acquisitions to de-risk its development portfolio.
To better leverage its partnership with Watson for Drug Discovery, Pfizer will need to be cognizant of any biases the algorithms may contain. Machine learning techniques for process improvement are only as good as the data used to create them. [6] If Pfizer wants to focus on immuno-oncology drugs, it will need to make sure that the research articles in the Watson for Drug Discovery database sufficiently covers immuno-oncology. It will also need to beware of over-representation so that it does not over-index on more widely researched genes, drugs, and diseases. Furthermore, correlation does not imply causation, so Pfizer will still need to perform sufficient preclinical tests on each candidate. In the medium term, Pfizer can allow quantitative data it collects on the sensitivity and specificity of the machine learning algorithm to guide its investments in future drug pipelines. It can also look into using machine learning to guide a Bayesian approach to clinical trials. Bayesian adaptive design trials may lower the number of patients needed to complete a trial by loading each arm of the trial that appears more promising with more patients. [7] Lastly, rather than looking at existing relationships among drugs, diseases, and genes in literature, Pfizer can also use machine learning techniques to study features of compounds that may make them more suitable to be drug candidates; for instance, certain chemical structures are intrinsically more unstable or toxic in human bodies, and these should be screened out at an earlier stage. [8]
In the context of Pfizer, some questions remain. How much of their R&D budget should they invest in machine learning to help with internal development of drugs, especially when the majority of their successful drugs on the market come through acquisitions? Furthermore, how much can they rely on machine learning techniques when no such techniques have proven financially or clinically successful so far and there continues to exist a black box around machine learning rationale?
(Word Count: 797)
Works Cited
- “IBM and Pfizer to Accelerate Immuno-Oncology Research with Watson for Drug Discovery.” Pfizer, December 1 2016, pfizer.com/news/press-release/press-release-detail/ibm_and_pfizer_to_accelerate_immuno_oncology_research_with_watson_for_drug_discovery, accessed November 2018.
- Kola I, Landis J. Can the pharmaceutical industry reduce attrition rates?. Nat Rev Drug Discov. 2004;3(8):711-5.
- Maitland ML, Hudoba C, Snider KL, Ratain MJ. Analysis of the yield of phase II combination therapy trials in medical oncology. Clin Cancer Res. 2010;16(21):5296-302.
- Sennaar, Kumba. “AI in Pharma and Biomedicine – Analysis of the Top 5 Global Drug Companies.” TechEmergence, October 10 2018, techemergence.com/ai-in-pharma-and-biomedicine/, accessed November 2018.
- Panteleev J, Gao H, Jia L. Recent applications of machine learning in medicinal chemistry. Bioorg Med Chem Lett. 2018;28(17):2807-2815.
- Zhong F, Xing J, Li X, et al. Artificial intelligence in drug design. Sci China Life Sci. 2018;61(10):1191-1204.
- Zhang L, Zhang H, Ai H, et al. Applications of Machine Learning Methods in Drug Toxicity Prediction. Curr Top Med Chem. 2018;18(12):987-997.
- Lo YC, Rensi SE, Torng W, Altman RB. Machine learning in chemoinformatics and drug discovery. Drug Discov Today. 2018;23(8):1538-1546.