Irrational Exuberance: Machine Learning at the Federal Reserve
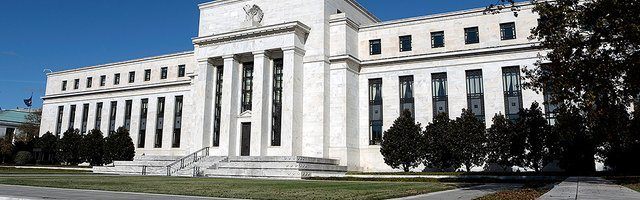
As the Federal Reserve enters its second century, will innovations in machine learning and artificial intelligence put our central bankers out of a job?
Machine learning (ML) and artificial intelligence (AI) will meaningfully improve the way the Federal Reserve (Fed) designs interest rate policy and manages financial stability risks in the United States. These two key responsibilities are entirely dependent on the quality of the Fed’s data, modeling, and surveillance of the economy and capital markets. With ever increasing amounts of data produced by firms and the economy at large, ML will significantly expand the Fed’s ability to understand and forecast market conditions [1].
Setting Interest Rates and Financial Stability
Machine learning can solve two main difficulties with business cycle analysis. First, the Federal Open Markets Committee (FOMC) relies heavily on lagging economic indicators to make its interest rate decisions. For instance, in the US, the unemployment rate is surveyed and computed only once a month [2]. During a financial crisis, relying on the monthly unemployment rate will leave the Fed a step behind. Deep learning, however, can provide more accurate and faster “nowcasts” of key economic indicators given vast quantities of consumer and financial data available today [3].
Second, the Fed’s models are completely reliant on economic theory to understand the economy, requiring theoretical cause-and-effect links. As evident in the financial crisis, prevailing macroeconomic models did not appropriately model the effect of the financial sector for prediction purposes [4]. Neural networks can uncover patterns in economic data “without the constraints of theory,” giving policymakers even more insight into where the economy is trending. [5]
Furthermore, ML can improve the Fed’s oversight over the stability of the financial system, a responsibility known as macroprudential supervision [6]. First, ML can provide better “early warning” signs of potential bank failure by identifying crucial correlations between credit or deposit data and bank weakness [6]. Second, ML can hep Fed regulators understand if banks are gaming capital regulations, given substantial grey area in bank capital standards and vast amounts of data used in capital stress testing. [7]
Machine Learning at the Fed
The Fed itself is approaching ML through a decentralized approach, allowing the 12 regional Fed banks to independently pursue disparate strands of ML research. Today, the Fed is already using automated ML “heat maps” in its annual bank capital assessments (CCAR) to uncover financial stability risks [8] as well as for back-testing and validating banks’ capital loss models [9]. The Fed also uses natural language processing tools at large financial institutions to examine emails and search for potential signals of control failures or misbehavior [10].
Researchers at the Federal Reserve Bank of Kansas City have developed neural network models which can more accurately forecast unemployment than any other currently existing models [11]. And today, three regional Federal Reserve banks publish their own online ML-based nowcasts of GDP or inflation. Although the FOMC does not yet refer to nowcasts in its interest rate announcements, it seems likely that in the medium term, these ML driven forecasts will become an important datapoint for the Fed’s monetary policy, especially as more data is collected.
However, there is no unified ML or AI strategy at the Fed currently. I would recommend that over the short term, the Fed: 1) Pursue a more centralized approach to developing its ML strategy. The potential benefits of ML in accuracy and speed necessitate a more coherent approach. The Board of Governors should convene a task force to identify the ways the Fed could best implement ML in its monetary policymaking, instead of relying on bottoms-up innovation from regional Fed banks. 2) Collaborate with other central banks to develop ML policymaking best practices. Global central banks are all facing similar ML issues, and financial stability and monetary policy is inseparably linked across markets. The Fed should work with other leading central banks to harmonize approaches to ML and share best practices.
In the medium-term, the Fed will need to develop the necessary private consumer and financial data streams necessary to create meaningful ML models. ML requires copious amounts of data, and the Fed may not currently have access to crucial relevant datasets, including credit card, web search, and financial market data. The Fed should work with Congress, other regulators, and the private sector to find privacy-protected ways to obtain these data to power its ML models.
Finally, there still remain crucial questions for the Fed’s use of ML in its fundamental responsibilities. For interest rate setting, given the limited historical span of market data and the even smaller number of recessions, to what extent can the Fed produce meaningfully predictive economic models? In its role as a regulator, are there other ways that the Fed can deploy ML to provide stronger macroprudential supervision of financial markets?
(Word Count: 771)
[1] Kliesen, Kevin L., and Michael W. McCracken. “Tracking the U.S. Economy with Nowcasts.” St. Louis Fed. November 21, 2017. https://www.stlouisfed.org/publications/regional-economist/april-2016/tracking-the-us-economy-with-nowcasts.
[2] Kuczynski, Michael. “Financial Times Unemployment Rate Is a Lagging Indicator.” Financial Times. August 8, 2013. https://www.ft.com/content/ae5e3b40-ff89-11e2-a244-00144feab7de.
[3] Financial Stability Board. “Artificial intelligence and machine learning in financial services.” November 2017, available at: http://www.fsb.org/2017/11/artificialintelligence-and-machine-learning-in-financialservice/.
[4] Stiglitz, Joseph E. “Where modern macroeconomics went wrong.” Oxford Review of Economic Policy 34, no. 1-2 (2018): 70-106.
[5] Wall, Larry D. “Some Financial Regulatory Implications of Artificial Intelligence.” Journal of Economics and Business (2018).
[6] Wall, 2018.
[7] Ibid.
[8] Arner, Douglas W., Jànos Barberis, and Ross P. Buckey. “FinTech, RegTech, and the reconceptualization of financial regulation.” Nw. J. Int’l L. & Bus. 37 (2016): 371.
[9] Quarles, Randy. “2018 Financial Markets Conference – Keynote: A Conversation on Machine Learning in Financial Regulation.” Federal Reserve Bank of Atlanta. https://www.frbatlanta.org/news/conferences-and-events/conferences/2018/0506-financial-markets-conference/transcripts/keynotes/quarles-conversation-machine-learning.aspx.
[10] Quarles, 2018.
[11] Cook, Thomas R., and Aaron Smalter Hall. Macroeconomic Indicator Forecasting with Deep Neural Networks. No. RWP 17-11. 2017.
Very interesting and well presented summary! I agree wholeheartedly with your point that the Fed should focus on creating the relevant data streams to enable a ML process. This is the main shortfall I see in this potential ML approach – after all, the modern US economy has only existed for ~100 years, and most of the data streams we would have access to today (e.g. consumer credit card purchases) have an even shorter history. So, I wonder if their is enough “training data” to allow ML any confidence interval on predicting what will happen next. However, the Fed should absolutely start collecting as much data as possible today, if only to give more viability to ML in the future.
Jonathan,
Super interesting piece! I have two questions after reading your post:
1) if the Fed does start to rely on an algorithm to set monetary policy, will the market (especially quant hedge funds) back-engineer the algorithm and try to take advantage of it?
2) I am afraid what the ML model would fail to incorporate is the element of fear and other investor behaviors. For example, in 2008, the financial crisis was not really caused by connectedness of banks or if some financial institutions won’t have enough capital to absorb the counter-party loss posted by Lehman’s failure. Instead, the entire financial system was brought down by contagion of fear and run on money market funds within days. So I am not sure if ML model based on data will be able to incorporate the behavior elements of the irrational market.
Excellent article. Interesting and relevant topic, and very well written.
The points where I do not necessarily agree are:
1) Is a more centralized approach really better than the current system where different models are being tested and compared? Similarly to other technologies (or technological applications), in their nascent phase it is often valuable to have a a cone of divergent solutions preceding a convergent cone. My impression is that we are not mature enough to enter the convergent phase.
2) Assuning that it is a supervised learning algorithm, isn’t this approach going to repeat mistakes of the past? For instance, given a training set (i.e. the historical data) that was meaningfully impacted by Quantitative Easing and other hotly-debated monetary practices, is the model going to repeat those measures without questioning if they made sense in the first place? In other words, if there is still no consensus about the economic theory, should there be a model to keep propagating the past?
Extremely well written and thought-provoking piece! Really enjoyed reading it.
Some thoughts,
The author mentioned “Deep learning, however, can provide more accurate and faster “nowcasts” of key economic indicators given vast quantities of consumer and financial data available today” – my question is how would deep learning necessarily be able to pick out the relevant data that is leading indicators versus lagging ones? The ML relies on data as well as algorithms so not sure the Fed will have the right input in terms of numbers or parameters to look at.
Also, the capital market doesn’t have that long of a history and I would if the data size is enough for the ML requirement to predict different cycles. It can clearly do better than human but how would a Fed’s ML compete with a D.E Shaw or Citadel?
When I think about the Feds and its decisions, I also think there’s a lot of macro-economics conditions and policy-making influences so I do wonder how much help ML can provide.
Nevertheless, a great piece and I enjoyed reading it!
Well written article about the potential of machine learning to revolutionize central banking. I agree that ML should become a standard part of the Fed’s process of evaluating present and predicting future economic conditions. Moreover, greater predictability of the Fed’s decisions, which could be the result of the implementation of a standardized ML model, would only be good for economic stability. However, I believe we are very far from deferring the responsibility of raising or lower interest rates to a ML tool. A ML tool can help inform such decisions, but a decision with such widespread societal implications will most likely continue to be decided by people, albeit people better informed by ML prediction tools.
“In its role as a regulator, are there other ways that the Fed can deploy ML to provide stronger macroprudential supervision of financial markets?”
I found this quote particularly interesting and it got me thinking that the Fed should actually go one step further than establishing a national task force. They should work with the European Central Bank and others from the very onset. This can help (1) make sure banks are collecting global data from global organizations and (2) establish meaningful oversight of companies that operate an increasingly globalized world. I suspect there may be some pushback from multinationals but there’s a role for government and international organizations to step in and ensure that banks get access to data in a privacy-compliant way.
Really interesting, well written article – thanks! Before reading this, I hadn’t thought of using ML to fill in the gaps between the GDP/inflation/unemployment estimates that are spaced out. I wonder whether ML can really make policy recommendations. My understanding is that ML algorithms work by guessing the relationship between a set of many input variables and a single output variable by repeatedly guessing and receiving feedback about the quality of the guesses. It can take a lot of iterations before the algorithm “learns” to guess well, it needs a lot of data. I wonder whether 50 years of monthly economic statistics (measured with error) would be enough. There might also be a problem where the algorithm would never recommend policies that hadn’t previously been tried.
Thanks for a compelling read. I think more generally ML has been applied to a few financial contexts but the Fed is a new one I haven’t really explored, but obviously should, I only question a few of the fundamental tenant of the Fed’s role and whether it is really in a place to inherit outputs from a system that is mostly using inputs of lagging versus leading indicator. Most people have already recognized that economic models are rarely if often ever accurate and especially in light of the financial crisis, not as relevant as many would like. Do you really think ML can do much better? Even with neural networks? As a “black swan” event, a crisis doesn’t exactly seem like a predictive result. Also, can this model be applied globally to other central banks? I’m not sure it can be calibrated to understand cultural economic differences. Economies fundamentally rely on consumer behavior which can be so different across cultures and regions. Regulatory differences, reactional differences to things like increased rates or decreased supply and taxation can lead to differences in reactions to monetary and fiscal policy, I’m not convinced this could be used practically across the board.