No More Blackouts: How PG&E is using machine learning to strengthen the power grid
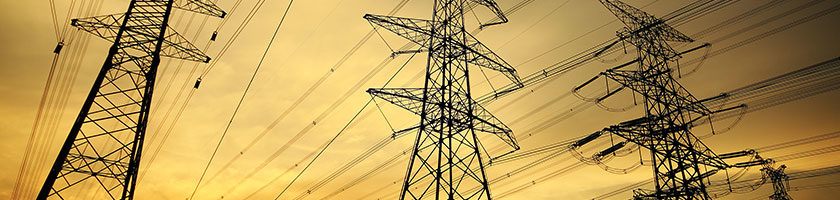
As society’s reliance on constant energy consumption continues to increase, Pacific Gas and Electric is investing in deep learning capabilities to enhance grid reliability and to integrate distributed energy resources at massive scales.
For the past decade, PG&E has been investing heavily in laying the ground work for applications of machine learning. As of 2014, PG&E had installed smart meters across its entire service territory, covering over 9 million properties. These meters generate massive quantities of data, over 2 terabytes per month and 100 billion meter readings per year, which exceed the capabilities of traditional analytics. [1] PG&E has started to develop applications in deep learning, a subset of machine learning that uses neural networks to enable more human-like and independent decision making, in order to pursue two primary process improvement goals: maximum grid reliability and integration of distributed energy resources.
Grid Reliability
In 2003, the importance of grid reliability was demonstrated when a transmission line in Ohio sagged and hit a tree, disrupting the flow of electricity through that line. The alarm system failed and therefore did not notify the utility, and soon after the grid experienced cascading failures as fewer connected generation resources were being pushed beyond their limits in attempting to supply the uninterrupted demand of end-consumers. The result was a multi-day blackout impacting over 50 million people in the Northeast United States and costing $6B. [2] To protect against a similar failure in their territory, PG&E is leveraging machine learning capabilities to optimize both proactive and reactive actions.
PG&E currently has two machine learning tools to address grid reliability. The first tool, “Fault Location, Isolation and Service Restoration” (FLISR), relates to addressing the type of issue that resulted in the 2003 blackout. This tool using algorithms to enable the grid to be self-healing, restoring service automatically following an electric fault through rerouting. This tool has been in use for multiple years and has had an immediate effect. Going forward, PG&E will seek to make this more robust through the installation of smart sensors on the transmission lines to enable faster physical repairs and improved preventative actions (e.g., cutting high risk tree branches). [3]
The second tool, “System Tool for Asset Risk” (STAR), is a dynamic risk scoring model for all of PG&E’s assets, enabling prioritization of asset replacement and maintenance. This predictive maintenance capability will apply to everything from major substations to individual power poles, ultimately lowering costs and further reducing the frequency of outages [3]. Predictive maintenance is an application of machine learning with growing popularity, but in order to learn requires significant data points of example failures. With the industry as a whole experiencing greater baseline reliability, hopefully PG&E will seek to collaborate with other utilities to establish an industry standard in preventative maintenance through shared data.
Distributed energy resource integration and management
Beyond reliability, distributed energy resource (DER) management has become one of the greatest industry challenges and opportunities. Historically, the US electric grid has connected roughly 5,800 utility scale power generation assets with the hundreds of millions of customers consuming energy. [2] Today, PG&E alone has over 300,000 private solar customers generating their own electricity and contributing some energy back to the grid, and over 85,000 electric vehicles (EVs) which represent roughly 20% of the total US EV market. [3] Effectively integrating these resources and ensuring the overall grid is constantly balanced between supply and demand is a challenge which requires the use of machine learning, and is a major area of future opportunity for PG&E.
Deep learning facilitates integration and management of DERs through load forecasting and accessing demand-side flexibility. [4] Energy consumption levels vary throughout the day in patterns influenced by weather, major events, holidays, and other factors which can be built into a forecasting model. Similarly, renewable energy resources have introduced significant variability on the electricity generation side due to variable sun and wind conditions on an hourly and seasonal basis. Connecting utility and private assets which can both charge and discharge (e.g., batteries, EVs) to the grid, paired with deep learning algorithms to optimize when to charge and discharge, can balance the grid and allow for both reduced overall capacity and greater allocation of capacity to renewable resources (see Exhibit 1) [5] Effective application of this type of system is expected by to limit the increase in US generation capacity to 1% relative to a 25% increase in energy consumption by 2050 due to the smoothing of consumption peaks across more of the day. [6]
Exhibit 1: Illustrative impact of storage assets on load profile and centralized capacity needs

PG&E is well on its way to becoming a “utility of the future” fully integrating IoT sensors with machine learning algorithms capable of driving improved grid outcomes based on that data. What remains to be seen is how PG&E will adapt its business model to capture the value provided in its increasing role as grid integrator and maintainer rather than primarily deriving value from its role as power intermediary.
(Word count is 783)
References
[1] Twentyman, Jessica. “PG&E: Where Big Data Meets the Internet of Things.” I-CIO, May 2014, www.i-cio.com
[2] McClelland, James. “Connected Assets: How Machine Learning Will Transform the Utilities Industry.” Digitalist, February 2018, www.digitalistmag.com
[3] PG&E Interview
[4] Mooney, Gavin. “10 Ways Utilities Companies Can Use Artificial Intelligence and Machine Learning.” Digitalist, May 2018, www.digitalistmag.com
[5] St. John, Jeff. “California’s Distributed Energy Grid Plans: The Next Steps.” Greentech Media, July 2018, www.greentechmedia.com
Thumbnail courtesy of Shutterstock
While I see the application of machine learning through FLISR and STAR as a way to improve P&G’s performance through smart sensing and fixing faults (reducing variability) as well as rerouting (thereby adding a buffer of sorts), I am curious if there are predictive applications being used on a commercial scale. Has machine learning been applied to load forecasting? Moreover, are there any inherent challenges in the reliability of predictive models given that the model contains idiosyncratic shock variables such as variability in weather and regulatory changes? I imagine that the accuracy of predictive algorithms will improve with time but curious how they control for idiosyncratic variables in the logit or regression models.
This is a very interesting application of machine learning that truly allows a company to benefit from the intelligence gathered from the large operating data sets. Natural disasters are becoming more prevalent and these systems that PG&E are building will allow them to do a much better job at preventing loss of electricity and help them restore electricity to their customers in a more efficient manner. I’d like to see where they take machine learning next in their company.
PG&E is facing a lot of pressure this year after the ferocious wildfire season in California, some of which may have started at utility facilities. Perhaps a machine learning algorithm that looks at factors that could cause wildfires would be helpful. I imagine they could use that information to make targeted improvements to facilities that reduce the likelihood of a substation sparking a fire, though finding appropriate data to feed the algorithm may be a challenge, and it may simply be too random and hard to predict.