“News”, “News”, “Fake News”: Can Machine Learning Help Identify Fake News on Facebook?
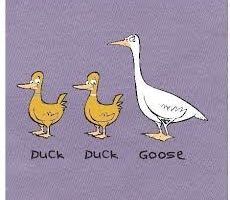
Among those wishing for a do-over on the 2016 presidential election, Facebook is somewhere high on the list. Can machine learning help them avoid repeating their mistakes?
After widespread criticism that “fake news” disseminated through the social media site may have directly affected the outcome of the election, Facebook has invested heavily in its capability to identify and interrupt the spread of misinformation.
But despite an obvious effort to address the problem of fake news, many challenges remain. As of February, Facebook had around 7,500 of its own content moderators (up 3,000 since May 2017) [1], and it has also outsourced the task to fact-checking media companies like Philippines-based startup Rappler. Facebook and Rappler employees alike have reported being under-resourced to effectively evaluate the large and growing volume of news articles—fake or otherwise—and some Rappler employees have even reported death and rape threats in response to their work [2].
To keep up with the growing volume of fake news without hiring fact-checkers at increasing rates, Facebook has sought to narrow the funnel of articles fact-checkers must review by experimenting with a number of AI and machine-learning approaches.
Facebook’s approach
This year, Facebook acquired Bloomsbury AI, a London-based artificial intelligence startup specializing in “trawling” documents for patterns and relationships—a signal that it views AI and machine learning as a critical component in its fake news arsenal [3].
In the near term, however, the scope of machine-learning’s impact on the challenge of fake news has been and will continue to be limited by factors such as the immaturity of natural-language processing technologies and the breadth of contextual data required to evaluate credibility.
The language problem is exacerbated by the widespread use of irony—a universal sore thumb for machine learning technology—and even “adversarial” writing, whereby fake news authors actively obscure the intended message of their articles to avoid detection by algorithm. In the style guide for Nazi publication The Daily Stormer, founder Andrew Anglin writes, “The unindoctrinated should not be able to tell if we are joking or not” [4].
On the credibility side, a 2018 Georgia Tech Research Institute study concluded that “While modeling text content of articles is sufficient to identify bias, it is not capable of determining credibility.” Unlike bias, which was reliably estimated by the presence of certain key words, credibility assessment was “highly tailored to the specific conspiracy theories found in the training set” [5].
Given these limitations, it is not surprising that Facebook has continued to utilize human eyes by designing a platform that feeds suspicious stories—many of which were flagged by algorithms—to a dashboard viewed by human fact-checkers at third-party accredited publications and internally [6]. Also unsurprising is that it tends to hire content reviewers not for their technical skills, but for their language expertise [7].
Based on the evaluations by fact-checkers, Facebook will display stories flagged as false lower on people’s newsfeeds, rather than removing them altogether [8].
Going forward
With advances in natural language processing, it is likely that machine learning technologies can assume more of the burden of identifying fake news, but Facebook should take several more active steps to meet the challenge of a growing and evolving fake news universe.
Through initiatives like the Facebook Journalism Project and the News Integrity Initiative, Facebook has already begun collaboration with news organizations, technology companies, and academic institutions aimed at helping individuals make more informed decisions about news consumption and funding research, projects, and products related to news veracity [9]. While this is valuable work, Facebook should aim to explicitly address the challenge of identifying credibility through article content by leading a coordinated effort to consolidate credible news data as training data for machine learning algorithms. Whereas previously, articles could be flagged for their similarity to established conspiracies, this data set might allow articles to be flagged for their deviation from established facts.
Outside of machine learning, Facebook has supplemented its team of trained fact-checkers by facilitating user’s ability to flag posts as suspicious. A limitation to this approach is the “echo-chamber” effect, whereby users are more frequently shown posts with messages with which they are likely to agree, and thus less likely to report. A solution could be to provide alternative newsfeed views through which users can read content that has not already been filtered to their preferences. While these user flags are not a substitute for professional fact-checking, like machine learning, they could help narrow the funnel of articles that fact-checkers must evaluate.
Open question
With so many advances in AI and machine learning still to come, it is difficult to predict how Facebook and other content hosts will fare in the battle against fake news. A concerning trend is the use of machine learning to create fake news content. How might Facebook and machine learning address this phenomenon?
Word Count: 793
1 Alexis C Madrigal, “Inside Facebook’s Fast-Growing Content-Moderation Effort,” The Atlantic, February 7, 2018, https://www.theatlantic.com/technology/archive/2018/02/what-facebook-told-insiders-about-how-it-moderates-posts/552632, accessed November 2018.
2 Alexandra Stevenson, “Soldiers in Facebook’s War on Fake News Are Feeling Overrun,” New York Times, October 9, 2018, https://www.nytimes.com/2018/10/09/business/facebook-philippines-rappler-fake-news.html, accessed November 2018.
3 Margi Murphy, “Facebook buys British artificial intelligence company Bloomsbury,” The Telegraph, July 2, 2018, https://www.telegraph.co.uk/technology/2018/07/02/facebook-buys-british-artificial-intelligence-company-bloomsbury/, accessed November 2018.
4 Andrew Marantz, “Inside the Daily Stormer’s Style Guide,” The New Yorker, January 15, 2018, https://www.newyorker.com/magazine/2018/01/15/inside-the-daily-stormers-style-guide, accessed November 2018.
5 James Fairbanks, Natalie Fitch, Nathan Knauf, and Erica Briscoe. 2018. Credibility
Assessment in the News: Do we need to read?. In Proceedings of
WSDM workshop on Misinformation and Misbehavior Mining on the Web
(MIS2). ACM, New York, NY, USA, 8 pages. https://doi.org/10.475/123_4
6 Madrigal
7 Ibid.
8 Mike Ananny, “Checking in with the Facebook fact-checking partnership,” Columbia Journalism Review, April 4, 2018, https://www.cjr.org/tow_center/facebook-fact-checking-partnerships.php, accessed November 2018.
9 Adam Mosseri, “Working to Stop Misinformation and False News,” Facebook for Media, April 7, 2017, https://www.cjr.org/tow_center/facebook-fact-checking-partnerships.php, accessed November 2018.
Great article, thanks for sharing. I think you alluded to how Facebook might address the potential concern of machine learning being used to manufacture, instead of detect, fake news. One of the advantages Facebook has in its war against fake news is its massive and massively diverse user base. Machine learning can be used to trawl through posts and detect content patterns indicative of fairness, and after that filter has been applied, Facebook can then solicit its users to evaluate flagged content to provide a level of nuance that their machine learning algorithms have not yet been able to achieve. Alternatively, Facebook could take the reverse approach and have users flag content to then be reviewed by its machine learning algorithm in an effort to speed the learning curve/accuracy of its fake news detection tool.
Very interesting to learn about the challenges that Facebook is facing as it tries to combat the proliferation of fake news. I was shocked to learn that so much of this process is done by humans! It seems like it would be very challenging for a machine to draw an accurate conclusion on the authenticity of an article. Perhaps one way it could be done is to try and create a depository of reputable publications, news sources and research institutions. Humans can validate the existence and accuracy of the platform and then once confirmed, can be added to the database. Over time, I would assume that most news-worthy events would be covered by these sources and the facts of a given article could be compared to this reputable database. Creating a dynamic database that would update in real-time may be challenging but with partner support could be possible.
Thanks for the in-depth analysis. You raised a lot of great points, especially how “fake news” culprits could conversely use AI and machine learning to actually create their content. That’s a very interesting point, and it makes me think this battle against fake news is just getting. This could be a major issue for the rest of our lifetimes.
One thing that really caught me off-guard was that Facebook chooses not to *remove* fake stories that have been identified by machine learning and fact-checkers as inaccurate. I understand that “penalizing” false posts by displaying them lower on newsfeeds will significantly limit it’s reach, but this makes me question Facebook’s true sincerity. How can they credibly launch the Journalism Project and News Integrity Initiative if they aren’t willing to remove fake content from their platform?
Thank you for laying out so thoughtfully such an important problem facing Facebook and our society in general.
The most intriguing part of your essay arrives near the end during your discussion of the “echo chamber” effect. This effect is in fact created intentionally by Facebook’s algorithm — Facebook engineers have designed the news feed algorithm with the specific intent of giving you more of what they think you want to see. Bad actors who wish to spread fake news exploit this algorithm by creating polarizing content that those at the far ends of the distribution are more likely to read, share, and react to, which in turn makes those same people more likely to receive similar content.
As a result, it seems to me that Facebook is in some ways battling itself on this issue. Constantly supplying new training data so that Facebook engineers can refine their fake news-detecting algorithms will be a continuous uphill fight. The simplest solution would be for Facebook to use a separate news feed algorithm for sharing news articles versus the one used for curating friends’ statuses and photos, an algorithm that selects news articles from a set of pre-approved and accredited news sources (not according to “likeliness to read”). That would mark a return to the old-fashioned days when the set of available news sources was much narrower in scope. But alas, I doubt Facebook would do something so drastic that could impact their growth and profitability.
You highlighted the challenge of picking the goose from amongst the ducks. Gauging bias and credibility is very challenging – not just for machines, but for humans too. You mentioned the difficulties machines have cutting through creative uses of language to side-step the algorithms, but in my view the gap will get narrower and it’ll become more and more difficult to bypass algorithms as they improve over time.
To address your question, it becomes a war of capabilities. Who has the better engineers – the ones building fake news engines or the ones that are trying to weed out fake news. I would argue it’s the latter — the good guys should win this battle.
Great report, thanks for sharing! As social media becomes an ever more important news source for the average voter, operationalizing an effective and trustworthy content review system is critical for the health of a democracy. I agree with your recommendations on how to practically implement a scalable solution: by blending machine learning with user flagging. Although I believe that at the end of the day, a judgment call will always need to be made on what to do with content.
To Melcolm’s comment on whether to remove or simply de-prioritize fake news, I wonder if we have to ask a larger operations question: is Facebook’s platform approaching the status of journalism / free press? If so, the protections afforded via freedom of expression within the US might jeopardize Facebook’s ability to exercise their own discretion on reviewing content. Rather than risking an expensive judicial backlash on Facebook’s judgment calls, I wonder if it would be better for Facebook to explicitly partner with the government and other free press institutions to explore how to best tackle the challenge of judging what to do with posts deemed “fake news”? This partnership could help Facebook make what I believe to be the most difficult operations decisions they have: what to do with fake news.
Great article! Thank you for sharing — I wish Facebook had started developing these new detective tactics at some point between 2012-2015……
I love the creativeness of some of your recommendations, however, I am having a difficult time trusting that we can put our faith back in the judgment of the user. While I can imagine a the scenario in which you proposed where users can read/see content that has not already been filtered for their preferences, I can also see a world where the consumer begins to become frustrated with the new user experience. Their perception may be that Facebook’s personalization algorithm is no longer optimizing their experience, leading them to make irrational decisions when flagging an article
as suspicious, or even just ignoring the flagging process altogether.
Do you truly believe that Facebook will be able to attack the scope of this project by the next presidential election? I have my concerns that Facebook won’t be able to quickly scale the process of “feeding” the machine learning tools enough contextual data to actually asses the nuances and credibility of an individual article.
Interesting topic!
Great essay to address the interesting topic.
I am curious about the credibility part. You mentioned that Credibility assessment was “highly tailored to the specific conspiracy theories found in the training set”. what specific theories are they? how to tailor it? I believe the credibility is a great challenge in making machine learning go on a commercial way on fake news judgement.