Navigating Drug Discovery using AI
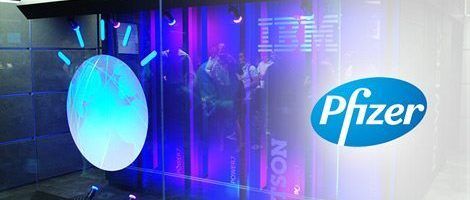
After the boom of Blockbuster(drugs with revenues exceeding $1B/yr) approvals in the 90’s, there has been a slowdown in the development of new drug entities (NDE). With drugs already in the market for easy to identify targets, research in academia and pharmaceuticals is now focused on more complex diseases. Conventional drug discovery has historically been a slow and expensive process. A study from 2014 found that the cost of drug discovery has gone up from $400M in 1980’s to $2.6B in 2014[1]. This coupled with long timelines in discovery and development (10+ years spent on average) has led to a drastic slowdown in the total number of NDE’s approved by the US FDA.


Between 5-6 years are spent in the discovery phase which includes target identification, screening and lead optimization followed by lab studies. Thousands of target sites and molecules go through time-consuming rounds of screening before an NDE is approved for launch by the FDA. Due to pricing pressure from payors and the need for customization for precision medicine, using Machine learning(ML) can be utilized to improve on-time and under-budget delivery of drug development projects.
Utilizing AI to unleash Drug Discovery at Pfizer
To this end many pharmaceutical companies such as Roche, Novartis and Eli Lilly are focusing on ML algorithms to reduce cost and time to market. Pfizer is at the forefront of this charge in the pharmaceutical space through its internal analytics teams and its collaborations with technology companies to help identify targets, identify new molecules and screen them virtually to identify and improve effectiveness and safety.
Pfizer has been using ML and big data to help identify candidates for its clinical trials. With more than 50% of clinical trials not finding enough patients to provide meaningful results [3]. Through its internal analytics team, Pfizer is focusing on using ML on publicly available data around hospital admissions, prevalence of diagnoses and demographics to help increase recruitment in clinical trials. Through its efforts Pfizer has been able to identify previously undiagnosed patients, especially with rare diseases, increase enrollment into trials for its drugs can improve the allocation of funds spent in patient recruitment [4].
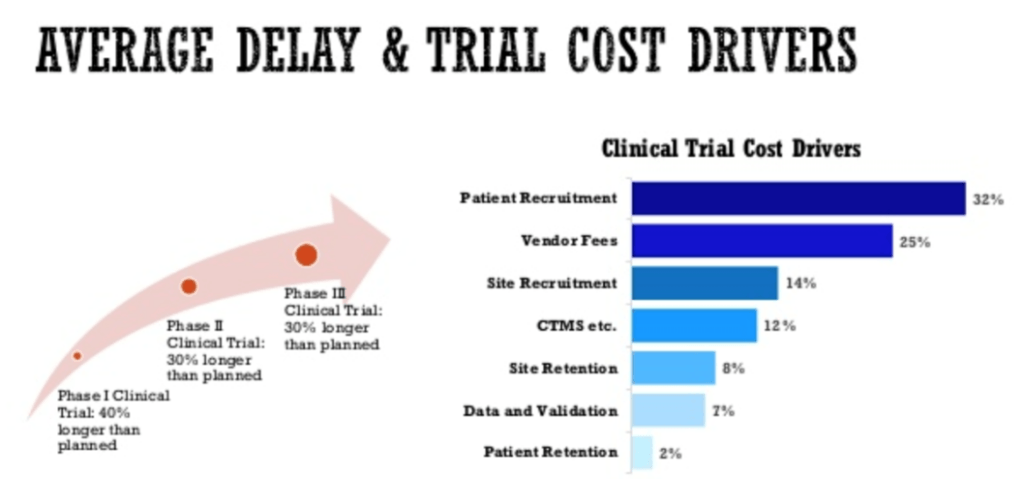
To take a leadership position in the medium and long term, Pfizer has announced partnerships with IBM Watson and Atomwise. In its recent announcement with IBM Watson, Bob Abraham, SVP and Head of Oncology said that “no single human being has the expansive knowledge to allow one to unravel the complexity of the interplay between immune system and cancer”.[5] The average scientist in pharmaceutical innovation reads arounds 200 research articles a year, compared to the 25 million articles and 4 million patient’s data ingested in Watson. Through this expansive data set, and the ability to draw orthogonal linkages between previously siloed data in disparate sources, Watson is helping Pfizer with hypothesis formulation and target identification while reducing timelines from 18 months down to mere weeks and at a fraction of the cost.
Pfizer and Atomwise are collaborating on computational molecular modelling to help design molecules to act upon target proteins and to predict the pharmaceutical properties of molecules before having to do lab trials[6]. Through screening millions of molecules through this simulation model, which predicts the properties and stability of the molecules, Pfizer can cheaply and quickly identify lead molecules to investigate further in a lab setting.
What’s Next?
As newer algorithms are developed there are a few areas in which Pfizer could collaborate with other players to maintain its leading position in the AI-based research space. One of the more exciting aspects of AI is being used by companies like Benevolent.Ai in testing previously developed molecules which failed to show effectiveness through screening models to identify potential uses and actions on other disease targets.[7] If successful this use case will enhance the ability to translate previous research into meaningful drugs which can help improve patient’s lives, provide a low input revenue stream for Pfizer and help keep costs low for payors.
Further as more integrated algorithms are developed, the two sides of hypothesis formulation/target identification and molecule design/pharmaceutical properties could become integrated further accelerating the impact of one on the other and enhancing the ability of both models to provide better results over time.
Questions around governance of data however surround this otherwise exciting space. In its efforts to accelerate and improve the drug discovery process there is a need to improve data governance especially around patient data being shared between collaborating parties. Further while Pharmaceuticals have historically been a highly regulated space with the need to find scientific backing around all research. With AI being such a new and unregulated industry, it will require a great deal of working with the regulators around reliability of AI based findings before we can realize AI’s true potential. (word count 798)
Citations
[1] Mullin, Rick. “Tufts Study Finds Big Rise In Cost Of Drug Development.” https://cen.acs.org/articles/92/web/2014/11/Tufts-Study-Finds-Big-Rise.html.
[1] Patwardhan, B., & Vaidya, A. D. (2010, March). Natural products drug discovery: Accelerating the clinical candidate development using reverse pharmacology approaches. Retrieved from https://www.ncbi.nlm.nih.gov/pubmed/21046974
[3] Kolata, Gina. “Lack of Study Volunteers Hobbles Cancer Fight.” The New York Times. August 03, 2009. Accessed November 13, 2018. https://www.nytimes.com/2009/08/03/health/research/03trials.html.
[4] Castellanos, Sara. “Seeking Insights into Rare Diseases, Pfizer Scales AI Analytics Platform.” The Wall Street Journal. May 10, 2018. Accessed November 13, 2018. https://www.wsj.com/cio/2018/05/09/seeking-insights-into-rare-diseases-pfizer-scales-ai-analytics-platform/.
[5] Health, IBM Watson. YouTube. June 12, 2017. Accessed November 13, 2018. https://www.youtube.com/watch?v=_qqIwfnmVrU.
[6] “Atomwise Enters Into an Evaluation Agreement with Pfizer.” Halo Top Creamery Is Now the Best-Selling Pint of Ice Cream in the United States | Business Wire. September 17, 2018. Accessed November 13, 2018. https://www.businesswire.com/news/home/20180917005163/en/Atomwise-Enters-Evaluation-Agreement-Pfizer.
[7] “BenevolentAI®.” BenevolentAI®. Accessed November 13, 2018. https://benevolent.ai/.
This is a very impactful application of machine learning that is becoming increasingly important in the pharma world. You mention the role of regulators in policing the judgement made in AI but I wonder really how much will change moving forward. To me AI seems to be the method to identify potential drug pathways and formulate a hypothesis for the most effective treatment for a specific cancer type or patient population. I would imagine that the drug candidates would still need to go through traditional clinical trials, unless the FDA creates a new fast track status for AI- discovered drugs where past data could serve as reducing what needs to be proven in a clinical trial or where the barrier is lowered for high risk cancers, etc.
Really enjoyed reading your article! The price of bringing a drug to market is always astonishing! The idea that machine learning can not only help with the timing to market, but also help fill trials is very compelling.
To your second question: we saw in the Watson case that diagnosing through machine learning has some risks. The incentives also don’t seem to be aligned with what is best for the patient–in finding patients for a trial, it seems like there may be an incentive to misdiagnose healthy individuals with the disease in order to increase success cases for a drug. I wonder how Pfizer is dealing with this incentive.
Your point on how much more can be processed through ML in pharmaceuticals is a good one! Overall, the upside for using ML in this industry seems to be enormous.