Making the Leap: Can Machine Learning Take Root in Animal Healthcare?
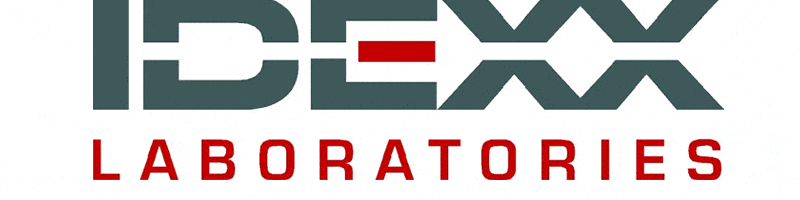
Can IDEXX successfully bring machine learning diagnostics to market in the animal healthcare space?
Companion animals are firmly established as full-fledged members of today’s modern family. As a result, veterinary and related services spending has grown tremendously (9.0% CAGR from 1980-2017 for veterinary-related spending relative to 5.6% for broader personal consumption) and the standard of care available to our four-legged friends has made tremendous improvements in beginning to narrow the gap between human and animal medicine.[1] Artificial intelligence research and associated product developments have become mainstays in human healthcare.[2] Now, IDEXX Laboratories is making the first move in the animal healthcare field to deploy machine learning with its new in-house urinalysis machine, SediVue Dx. The potential for future product development incorporating machine learning is enormous; however, the jury is out as to whether veterinarians and pet owners will embrace the big data revolution.
Research and development is a cornerstone of IDEXX’s strategy – the firm spends over 80% of the identifiable R&D dollars in the animal healthcare diagnostics industry.[3] IDEXX boasts an expansive network of reference labs, owns one of the leading practice management software packages, and develops in-house diagnostic machines that are fully integrated into the workflow of its client clinics.[4] With its launch of SediVue Dx in early 2016, IDEXX gambled that the industry is ready to embrace machine learning from a clinical and economic standpoint. Sedivue Dx is a machine that analyzes urine specimens using IDEXX’s proprietary “Neural Network 3.0” technology.[5] By utilizing machine learning software, SediVue Dx evaluates multiple images of a urine specimen for abnormalities such as the presence of blood cells, epithelial cells, bacteria, casts, and crystals. Comparing each image against a database of millions of other specimens that have already been analyzed and categorized, SediVue Dx returns images from the original specimen that are annotated with text and graphics that identify specific abnormalities.[6]
Last year, I led a pilot at the largest private owner of animal hospitals in North America to determine whether adopting the SediVue Dx into our clinics would add value clinically and financially. Our pilot illustrated both the promise of the technology and the limitations currently causing initial adoption to be somewhat muted. First, the machines and associated consumables are dramatically more expensive than sending the specimens to IDEXX’s reference lab for review or evaluating specimen in-house using a centrifuge and microscope. Additionally, the image outputted by the SediVue Dx requires the veterinarian to interpret the results to determine a course of action and many clinicians required refresher training given that the last time they had analyzed a urine specimen was back in veterinary school. The product itself continues to advance as its algorithms improve the accuracy with which it can identify abnormalities in the specimens, but versions 1.0 and 2.0 of the Neural Network technology were plagued by errors.
IDEXX’s commitment to deploying machine learning in animal healthcare should be heralded, but it must take dramatic action in the short and medium term to regain momentum to ensure that incremental product developments and new product launches incorporating the technology are well received. In the short run, IDEXX must continue to refine its Neural Network technology to ensure that SediVue Dx continues to improve its clinical accuracy and offer even more training to veterinarians and clinicians about how to evaluate the annotated output images. From an economic standpoint, much more work needs to be done to prove that shifting urinalysis volume from the reference lab to SediVue Dx in-house will lead to incremental treatments that will justify the higher relative costs.
Over the medium term, IDEXX has a tremendous opportunity to take its learnings from its initial machine learning launch and apply the technology to its existing portfolio of in-house diagnostics. IDEXX has tremendous amounts of clinical data from its reference labs and should investigate using machine learning to identify potential gaps in the industry’s current product.
As the company looks to the future, key questions remain. Do its investments in machine learning make financial sense given its position as one of the two dominant players in an industry that is essentially a duopoly? Can it recoup its significant R&D expenditures related to SediVue Dx and future machine learning products by charging higher prices to animal hospitals who may be warry of passing on these prices to pet?
(Word Count 800)
[1] Credit Suisse, “IDEXX Laboratories: The 2018 Maine Event,” August 17, 2008, via Thomson Reuters/Investext, accessed November 2018, 5.
[2] Yu, K., Beam, A. and Kohane, I. (2018). Artificial intelligence in healthcare. Nature Biomedical Engineering, 2(10), pp. 719-731.
[3] “IDEXX Growth Strategy” (PDF file), downloaded from IDEXX website, https://idexxcom-live-b02da1e51e754c9cb292133b-9c56c33.aldryn-media.com/filer_public/bf/b7/bfb75df1-2586-4f92-a017-100353d4492d/20180815-idexx-investor-day.pdf, accessed November 13, 2018, 17.
[4] IDEXX Laboratories, 2017 Annual Report, p. 6, https://idexxcom-live-b02da1e51e754c9cb292133b-9c56c33.aldryn-media.com/filer_public/03/ae/03ae8315-1723-4639-9a3c-e9d3606f941e/2017-10k.pdf, accessed November 2018
[5] “IDEXX Advances Power of SediVue Dx with Latest Software Update,” press release, February 5, 2018, on IDEXX website, https://www.idexx.com/en/about-idexx/news/idexx-advances-power-sedivue-dx-latest-software-update/, accessed November 2018, 1.
[6] IDEXX Laboratories, “SediVue Dx Urine Sediment Analyzer,” https://www.idexx.com/en/veterinary/analyzers/sedivue-dx-analyzer/, accessed November 2018.
Interesting read! One question I have is what is the overall value proposition for this platform? Is the purpose to become a more accurate or sensitive diagnostic tool than the current standard, or is it to ultimately be a cheaper alternative once economies of scale kick in? I agree that machine learning definitely has a place in animal healthcare, but I wonder if this is the best application. You mention both cost reduction and improved treatments as possible positive effects, but without a particular goal in mind for either the research seems hard to justify.
Good question! So the benefits of in-house diagnostics are that results can be determined while the patient is still at the clinic and thus the veterinarian can make a diagnosis and start a treatment plan on the same visit, which is incredibly valuable from a clinical and financial perspective given that follow-up appointment attendance is way lower than in human healthcare. SediVue Dx also offers an additional benefit in that in-house diagnostics have much lower rates of inconclusive tests than if the specimen is sent to a reference lab – urine samples can decay in transit causing a number of reference lab results to be invalidated.
Great article! This is a great application of machine learning especially for those that do have four legged friends. It’s interesting to think about the differences in bio-engineering for humans vs animals. The process seems to be similar with high capital expenditures early on the in the development of the product, however, it seems that the market for animals will be smaller which makes it harder for animal sciences companies to recoup their initial investments. Using machine learning may be able to help improve this situation by allowing simulations to help speed up the process. Interested in following the progress of this company as they continue on their mission!
I thought this was really interesting. When we think of healthcare, we tend to think only of humans, but technological advancements in that space should certainly have benefits for animals as well. I definitely want to learn more about this and see what other applications of machine learning the industry discovers. I’d imagine another of our megatrends, additive manufacturing, is also disrupting pet healthcare just like human healthcare, so it would be interesting to hear about that as well.