Making it Personal: How JetBlue is Using Machine Learning to Personalize the Travel Experience

JetBlue is partnering with a machine learning start-up so they can personalize product offerings to suit each traveler's needs.
In the U.S. alone, over 2 million customers book travel every day [1]. For each of these trips, there are individual motivations, wants, needs, and willingness to pay that determine when, how, and with whom these customers book their travel. Suppliers of travel, such as airlines and hotels, grapple with the difficulties of providing meaningful travel services to satisfy individual preferences, while also reaching the scale that allows for profitability. Machine learning can offer a solution to this dilemma, and JetBlue has taken steps toward incorporating machine learning into their travel product development.
Ever since the U.S. Government deregulated air fares in 1978, airlines have struggled to effectively match the services they provide with customer demand. In 1978, every customer was provided the same level of service (including “ancillary” products such as checked bags, meals, seat assignment, ability to cancel or refund, etc.) regardless if the individual passengers needed or wanted these services. Today, many airlines, including JetBlue, offer several different tiers of service for passengers with different “ancillary” products bundled together, while others such as Spirit employ a fully a-la-carte model. This trend toward individualization is continuing; in 2017, revenue from the sales of ancillary products made up 10.6% of total airline revenues, compared to 4.8% just eight years earlier [2]. In order to continue to offer further individualized product offerings, JetBlue’s management will need to gather real-time insights from ever increasing sources of data on existing and potential customers to offer personalized travel products to match each individual’s preferences and needs.
JetBlue’s management has taken steps to address this changing landscape of airline product offerings in the near term by partnering with FLYR Labs, a technology start-up focusing on enabling real-time dynamic pricing for the aviation industry [3]. FLYR’s technology utilizes machine learning to analyze incoming data streams from a potential customer (demographic information, past purchase behavior, online presence, etc.) and determine the optimal offering of air ticket, ancillary product, hotel, rental car, etc. to offer to that customer [4]. For example, JetBlue’s product bundling today looks like Exhibit 1 [5]:
Exhibit 1

There are four fare products (Blue, Blue Plus, Blue Flex, Mint) that each offer a specific combination of ancillary products for a given price point. In the future, with machine learning enabled algorithms, a search result for air travel may look something like Exhibit 2 [6]:
Exhibit 2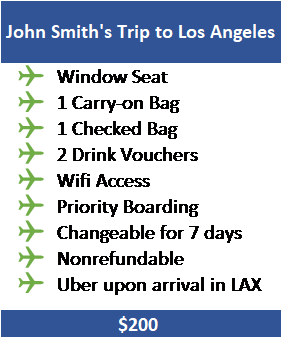
JetBlue can predict what ancillary services will be needed by each individual consumer, and can serve a personalized offering to them.
In the medium term, JetBlue has taken one main step toward the development of continued organizational improvement in machine learning, the creation of JetBlue Technology Ventures (JBTV)[7], a corporate venture capital fund. JBTV is currently the only travel provider corporate venture firm, and this position gives them access to thousands of start-ups that are looking to innovate with new technologies such as machine learning. According to Bonny Simi, President of JetBlue Technology Ventures, the venture fund allows JetBlue to “look around the corner and see what’s coming so we can adapt our business model as a large company to those shifts and also be a first mover in some of the great technologies that are coming forward” [8]. As new companies seek to use machine learning to enhance the aviation industry, JetBlue is ideally positioned to discover and invest in the most promising ventures.
Beyond the current steps that JetBlue’s management has taken to implement machine learning into their product development, I believe that there is opportunity to expand machine learning techniques beyond product development, and into process improvement. One example of a potential process improvement that JetBlue can explore is in the realm of passenger connections in their hub airports. With current technology, it is very difficult to track connecting passengers once they de-plane their first flight and before they board their next flight. Research has shown that machine learning algorithms are more accurate at forecasting passenger connecting times than current benchmarks and tools [9]. JetBlue can apply these techniques to predict which customers on specific arriving flights are most at risk of missing their onward connection, and then intervene to assist that passenger in making their onward flight and avoiding a service disruption.
One open question on the topic of machine learning for product development in the context of JetBlue is what challenges implementation will bring. The path from the current state to the future state described above is unclear, particularly due to the competitive environment in the travel industry. Although machine learning can in theory provide better value to both consumers and travel providers by more efficiently matching service levels to needs, the infrastructure and existing distribution channels can not support this kind of selling. How can JetBlue move forward towards more individualized product offerings without the rest of the ecosystem rejecting their efforts? (797 words)
[1] 2017 Bureau of Transportation Statistics, T-100 Market (All Carriers)
[2] 2018 CarTrawler Yearbook of Ancillary Revenue
[3] Wall Street Journal, “JetBlue Tech Execs Tap Startups To Help Airline Innovate”, July 13th, 2018
[4] www.flyrlabs.com
[5] www.jetblue.com , search results for BOS-LAX itinerary, accessed November 12th, 2018
[6] Created by Author
[7] www.jetblueventures.com
[8] Phocuswire.com interview, “In The Big Chair – Bonny Simi of JetBlue Technology Ventures”, August 22, 2018
[9] Forecasting Airport Transfer Passenger Flow using Real-time Data and Machine Learning, Guo et. al. HBS Working Paper 19-040
Very interesting perspective on how machine learning is disrupting such a critical industry! I especially appreciated the thought starter on how to apply machine learning more in process improvements, e.g. optimizing flight connections. Leveraging more data to identify passengers with late connections earlier for a better real-time reaction seems like a very promising opportunity not only for the airline industry but also for related industries, e.g. rail or general logistics and distribution applications.
The one aspect I would challenge is the statement that existing distribution channels cannot support the selling of individualized service levels such as JetBlue’s offering. With the emergence of more sophisticated web offerings, in the travel industry but also more generally, I could envision a world in which the channel and the personalized offering complement each other: Data collected via different distribution channels could be leveraged to optimize the machine learning algorithm, which then enables a better offering for the customer, making the channel more attractive. Both elements in the supply chain would benefit from each other in the mid- to long-term.
This was a really well-written article on how JetBlue is using machine learning to improve its production and processes. The fact that JetBlue now offers customized offerings of ancillary products is a cool concept that I was completely unaware of. In addition, the improvements to real-time dynamic pricing are not insignificant and I can see more potential progress being made in that realm. In response to your question, the infrastructure and existing distribution channels should be revamped to support these efforts. As long as JetBlue continues to innovate in the realm of travel, it can bring change to the existing infrastructure by working along the chain to push through more innovative offerings based on their machine learning. Since this would bring positive value to add parties involved, I can’t see why this wouldn’t be successful.
Thank you very much for such an interesting topic for HBS students – frequent users of airlines services. From a customer perspective unbundling of services is very convenient, as a customer can select a set of services that specifically represent his/her needs. However, what is the impact of “unbundling” on the airline companies, specifically on their financials? In my understanding, additional services generate high margins. Given that consumers increasingly treat airline services as a commodity, most of them will often go for the lowest possible price which will negatively impact over revenue and profitability of the airline industry. I clearly see how unbundling creates value for the customers, however, I would be curious to know how specifically airline companies navigate unbundling with the help of AI in such a way that creates not only value for a customer but also increase in revenues and profitability for the airlines.
First off – as a pretty frequent flyer, I really enjoyed reading about machine learning + JetBlue; you know your audience ;-).
While I agree that implementation of initiatives/products driven by machine learning will be challenging, due to the competitive environment, I’m not sure I agree with your hypothesis that the root of the challenge is based on inadequate infrastructure and distribution channels. I may be misunderstanding your wording, but to my understanding, airlines can sell an intelligent, individualized ticket-buying experience like you showed in exhibits 1 and 2 using their existing websites, and possibly even on platforms like Kayak or Priceline. What, in your opinion, is particularly challenging about the existing infrastructure/distribution channels? Did you have something else in mind besides what I listed?
I would venture to guess that Jetblue’s (and other airlines’) biggest challenge with rolling out an initiative, product, or process driven by machine learning is the difficulty of gathering (and using) consumer data. For this to be actually impactful, it would require consumers to opt in to their data being shared (or, if it’s already being shared, then it would require social approval by the consumer for the company to use it without being shamed). As a consumer, it makes me uneasy that airlines could use data about me to offer individualized offerings, because I would be skeptical and assume they are using their algorithms to upsell me or overcharge me unnecessarily. It feels more appealing to me to be offered a predetermined set of choices (i.e., 4 different ticket fares with different associated products/services like you show in exhibit 1), but NOT individualized offerings. I would forgo a personalized airline ticket shopping experience to protect my data.
I find the article very interesting since, as someone with experience in the airline sector, I can relate very much to the huge potential that ancillary revenues bring to the companies. These benefits come not only in terms of revenue potential, but also in terms of high profitability, with airlines having been able to transform a cost center into a profit center. Usually the clearest example is the onboard food, area in which airlines stopped spending a pretty considerable portion of their ticket fares in this service, and transformed it into a revenue source, this way saving the associated cost while receiving a profit for it. Having a machine learning based system to untap the potential of these additional profit sources is something very impactful in an industry with low and shrinking margins.
Regarding the last statement about the lack of existing distribution channels and enough infrastructure to support this initiative, on the one hand I do agree that there can be many challenges in the travel agencies/e-travel agencies channel, not mainly related to the infrastructure but to the amount of information that JetBlue is eager to share about its passengers with third parties. On the other, I do not see bigger issues for implementing this system in JetBlue’s own platform, on the contrary, I see a significant opportunity to gradually implement the system, testing the results, and being among the firsts in the industry.