Machine learning is not limited to Tech firms: Conventional Oil & Gas Industry Has Adopt The Advancement To Extract More Profit

Generally, machine learning is perceived for computer technology-driven firms. This article examines the use of machine learning in an old world industry such as oil and gas.
Machine learning, a technical term people use to describe a program that teaches itself to develop. Generally, the term is used in computer science, data science, and computer engineering. The first thing that an expert think about machine learning is the amount of data. The more data there is, the more applications of machine learning there are [1]. As a result, tech firm is always on top of the list of machine learning application. Then, what kind of industry, other than tech, that can generate such amount of data?
Oil and gas industry has been operating for more than a century. Chevron was established in 1876 in California. Its current business value chain ranges from exploration to distribution to gasoline consumers. Each operation generates a significant amount of data. For example, exploration requires geological maps, oil refining processes generate machine operation reports, selling gasoline tells us who are the consumers, and others. In upstream business, the complexity of data has evolved because of endeavor to save cost, improve safety, and reduce development time. In this article, we will examine machine learning within exploration and production.
Under the surface: How do geologists use machine learning?
Where do geologists find an oil reservoir? Everything starts underneath the ground. First, geologists conduct seismic scans, use a variety of complex tools to collect data, create geological models, and analyze the results to tell where to invest millions of dollars to do wildcat drilling, an exploratory phase of finding petroleum reservoir. Combining knowledge and experience, geologists decide which area has a potential of oil, gas, or both. Searching through data to find similar geological structure can help geologist making a better decision. [2]. With the new data generated every day and the complexity of seismic analysis, searching millions of charts from the company database might take days or weeks.

Chevron implements AutoML, a cloud-based machine learning analyzing tool from Google, to look through millions of documents, charts, tables, and figure of internal knowledge warehouse that otherwise would require days or week to find. Sometimes, considering rework is easier than searching. [3]
In the future, I would like to see if Chevron can use machine learning to tell which area is worth exploring. For example, machine learning may compare new geological data with existing proven reservoirs and tell us where to drill, even calculate necessary investments and returns.
On a processing plant: What can we do with information from thousands of equipment?
In an oil processing plant, thousands of sensors constantly detect and provide information about the machine operating conditions. When the machine breaks down, operators know the operational parameters such as flow rate, pressure, temperature, etc. What would be a better way to do so?
“In the past, operators can tell how the machine performs. In the future, the machine will tell us how it’s performing” Said CIO of Chevron [4]. Chevron is currently operating on a global scale with more than 5,000 heat exchangers, an equipment that uses to transfer heat energy from one liquid to another. Originally, maintenance would be done periodically by a preventive maintenance program. Each heat exchanger has its own period to be cleaned, repaired, and changed.
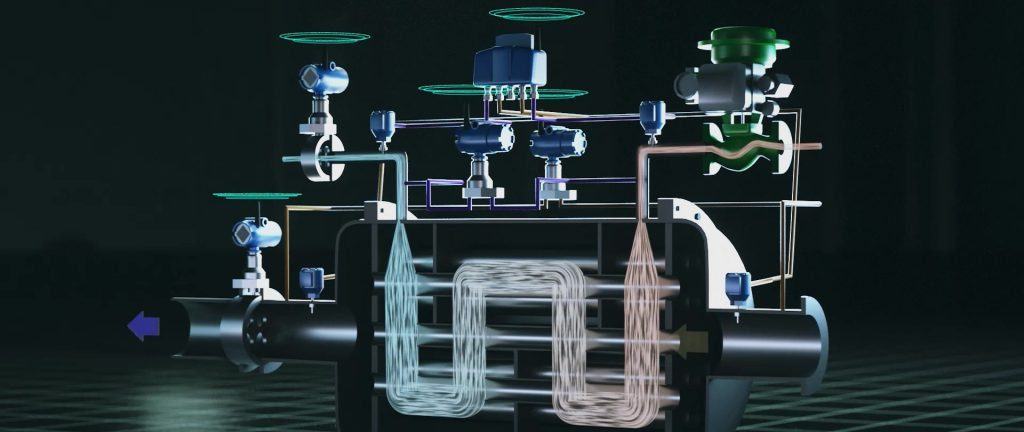
From machine learning, operators can build predictive models that make heat exchangers says something when it is about to need maintenance.
Not only in the heat exchanger, but in the more complicated machine such as distillation column, gas turbine, compressors, and others. In the future, Chevron plan to incorporate machine learning in more equipment. Chevron can reduce the cost of over maintenance by conduct maintenance only as needed.
However, one of the questions that I like to challenge is the potential of out of control loop. In a big integrated system, how can engineers make sure that the system will never go corrupted if the system logic itself beyond human’s intelligence to prove? Can we really trust the machine learning to operate our multi-million or billions of dollars asset?
Innovation Dilemma in oil and gas: Nobody wants to be the first mover in proving technology. Why?
Although technology is here to stay, unproven tracks of incorporating technology into this multi-million investments post a question “can the solution be safe and reliable enough?”
The industry has faced several major accidents, causing loss of life and money. The size of the consequence is usually multi-million. For example, BP Deepwater Horizon cost to 65 billion US dollars because of failure in interpreting data of equipment testing [5][6][7]. The technology has been used for many years, and yet can fail. This may cause major technology review within the industry.
Adding new technology, particularly no long-term track record, would cause reluctant in the oil and gas industry. What is the right tradeoff between unproven and proven one? How to start using innovation and still maintain or improve safety? Such a question needed to be answered first, before deciding which ranking to target.
[Word Count: 798]
[1] Mike Yeomans, What Every Manager Should Know About Machine Learning, July 2015, Harvard Business Review
[2] Leon Farrant, The role of a Petroleum Geologist Explained, June 2018, DARE Energy’s Article https://www.dare-energy.com/news/the-role-of-a-petroleum-geologist-explained/37697/
[3] Lavent Besik, A closer look at our newest Google Cloud AI Capabilities for developers, August 2018, https://cloud.google.com/blog/products/ai-machine-learning/closer-look-our-newest-google-cloud-ai-capabilities-developers
[4] Sara Castellous, Chevron Launching Predictive Maintenance to Oil Fields, Refineries, September 2018, The Wall Street Journal. https://blogs.wsj.com/cio/2018/09/05/chevron-launching-predictive-maintenance-to-oil-fields-refineries/
[5] Deepwater Horizon Accident Investigation Report, September 2018 https://www.bp.com/content/dam/bp/pdf/sustainability/issue-reports/Deepwater_Horizon_Accident_Investigation_Report.pdf
[6] David Hammer, Oil Spill Commission: BP’s final steps, misinterpreted pressure test sealed well’s fatal fate, November 2018, Nola.com https://www.nola.com/news/gulf-oil-spill/index.ssf/2010/11/oil_spill_commission_bps_final.html
[7] Ron Bousso, BP Deepwater Horizon costs balloon to $65 billion, January 2018, Reuters
I completely agree that machine learning will revolutionize the energy sector. The article describes that machine learning will help operator managers to predict when heat exchangers need maintenance. In a higher-level scale, machine learning, through the information gathered by thousands of sensors, will allow the operators of the electricity system to make better decisions on how to best allocate energy resources [1].
I strongly believe that big energy companies are willing to prove new technologies, despite having risk of losing money. In the one hand, new methods could allow companies to have a competitive advantage such as the reduction of costs of a project for both companies and clients. In addition, energy companies do large capital investments and then the test of new technologies (R&D) is part of their business model. To illustrate, during 2017 Chevron spent $864 million in R&D [2]. However, injuries or deaths of employees are risks that companies will avoid at any price. In general, both the ethical dilemma of exposing workers to hazards and the possible reputation damage, have led companies to put safety as a central pillar.
[1] Franklin Wolfe, “How artificial intelligence will revolutionize the energy industry.” Harvard Business Review Digital Articles (August 28, 2017).
[2] Reuters, “Chevron Corp”, https://www.reuters.com/finance/stocks/income-statement/CVX?stmtType=INC&perType=ANN, accessed on November 2018.
I agreed that machine learning has huge potential to revolutionize oil & gas industry. Due to the vast amount of data generated by company operation in this industry, there are many beneficial applications of machine learning to reduce the reliance on expensive human resource and potential human error associated with it. In addition to underground oil reservoir exploration and preventive maintenance program mentioned in this essay, another potential application area is in refinery operation – increasing the number of variables that can be managed in the operation by 10 times [1]
However, in transitioning from human-centered operation to a fully-automated machine learning, it is very imperative to do so very carefully. One option could be to trial the automated system under human purview, so that intervention can be made in case of system failure.
[1] Robert Brelsford, “Repsol launches Big Data, AI project at Tarragona refinery”. Oil & Gas Journal, June 6, 2018, https://www.ogj.com/articles/2018/06/repsol-launches-big-data-ai-project-at-tarragona-refinery.html, accessed on November 2018