Machine-learning in insurance is a sweet deal for customers
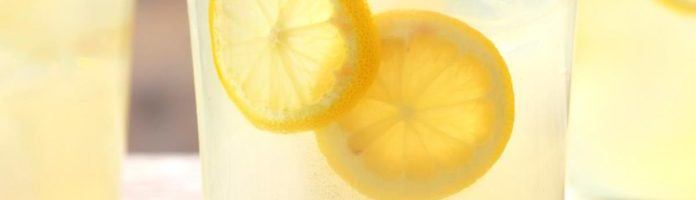
Tired of being given lemons by traditional insurers, customers are turning to Lemonade
Introduction
As we move further into the digital age, our ability to use machine learning, which uses statistical methods to find patterns of predictability in datasets,[1] to gather and analyze complex data is increasingly important. In a recent HBR survey, over half of respondents attributed significant improvements in business processes to machine learning.[2]
Incumbents vs disruptors
Lemonade, a digital insurer, is one such business looking to disrupt the industry by improving business processes and risk quantification. Incumbents typically have unwieldy one-size-fits-all processes and price policies using huge volumes of very basic customer records (e.g. age, ZIP code), known as ‘long’ data.[3] By contrast, Lemonade collects much more and diverse information (‘wide’ data), which is better suited for use in machine learning.[4] The company collects 100+ data-points upfront, which it builds with each interaction to refine customer experience and its view on asset risk.[5]
With the potential to markedly affect revenues and costs, it’s little wonder that Lemonade has joined an industry-wide analytics arms-race. And this is just the beginning.
Short-term implications
Lemonade is able to exploit machine learning to improve efficiencies now across multiple areas:
Opportunity | Description | P&L impact |
Better quantify policy risk | More holistic and yet more granular view of the individual risk. Enable insurers to tailor pricing accordingly [6] | Reduced claim costs |
Simplify claims management | Algorithms dealing with straight-forward claims, and triaging complex cases requiring human intervention [7] | Reduced operating costs |
Personalize underwriting process | Predictive models enable real-time decisions on coverage and pricing 24/7, and automatic data feeds enable customers input less data to get a cover decision [8] | Improved customer conversion rates |
Improve fraud detection | Sophisticated analysis pulls reams of data together to reveal fraud, which up to 25% of Americans purportedly engage in,[9] much faster than humans | Reduced claim costs |
Lemonade continues to grapple with the need to be profitable now, with the need to scale to drive future profitability. Customer growth is a clear priority (1000% growth year-on-year for 1H 2018, but this was on a loss ratio of 116%, which is ~2x a sustainable ratio.[10]
Scale matters from two perspectives: having a diverse and large enough policy book to insulate the business against catastrophe risk events such as storms, but also having the volume to better understand customer needs and more precise view on risk associated with a particular policy.
However, despite mounting losses, vast venture capital dollars are behind the business (Lemonade recently took on $120M from Softbank),[11] and it still feels like a J-Curve that the company is able to emerge from.
Recommendations
- Scale deep and narrow on customer/product: first generate a profitable analytics advantage, before replicating that across adjacencies
- Invest heavily in marketing: Lemonade needs dramatic customer growth to stay ahead of the competition [12]
- Manage investor dynamics: scaling the business by taking on more uncertain risks now to learn more is expensive, but arguably necessary to scale. Management must drive alignment between growth, fundraising and investors
Medium-term
Further opportunities
Over the next few years, additional opportunities to use machine-learning will emerge:
Opportunity | Description | P&L impact |
Adding Internet of Things (IoT) to business model | Using source data rather than proxies to quantify and even reduce risk through device data in the home and telematics (cars) | Reduced claim costs |
Predicting and mitigating churn risk | Dynamic algorithms to predict churn across multiple variables, and use measured incentives to keep them | Improved customer retention |
Much-hyped IoT is gradually giving insurers far more relevant data inputs to price risk through algorithms (e.g. telematics). Last year, Lemonade opened its API to enable integration with IoT players,[13] which will help adoption. It will also allow Lemonade to move beyond insurance to assurance (e.g. home protection – monitoring fire sensors and other devices) that will improve pricing and potentially reduce claims.
Recommendations
- Double-down on IoT: will fundamentally change the nature of risk pricing. Lemonade should consider how to incentivize customer adoption (e.g. through lower premiums, free equipment)
- Focus on the customer: in the long run, machine learning is arguably not a sustainable competitive advantage – it will be acquirable. At that point, Lemonade will need to refocus on the customer and how Lemonade can fit into their lives in order to win business
- Proactive regulatory engagement: significant regulatory risk exists around ‘unfair discrimination’ in pricing, especially if Lemonade could only point to predictability rather than any causality in some pricing factors [14]
Open questions
Regardless of whether Lemonade-like disruptors or more glacial incumbents triumph, customers should reap significant benefits. The outcome may turn on several key issues, including:
- Winning incumbents’ customers – how to establish trust given lack of human contact?
- Breadth of offering – worth developing solutions for car insurance and other adjacencies?
- Vision – is Lemonade a tech company trying to build and sell analytics, or position as a trusted brand for the long-term?
[794 words]
- A. Fedyk. How to tell if machine learning can solve your business problem. Harvard Business Review Digital Articles (November 15, 2016).
- H.J. Wilson, A. Alter, and S. Sachdev. Business processes are learning to hack themselves. Harvard Business Review Digital Articles (June 27, 2016).
- D. Schreiber. Precision Underwriting – Digitization enables precision medicine – why not precision underwriting?, Lemonade 101 Blog, April 3, 2018 https://www.lemonade.com/blog/precision-underwriting/ accessed November 2018.
- M. Yeomans. What every manager should know about machine learning. Harvard Business Review Digital Articles (July 7, 2015).
- D. Schreiber. AI Eats Insurance – Exactly why Artificial Intelligence will transform insurance, and exactly how, Lemonade 101 Blog, Jan 8, 2018 https://www.lemonade.com/blog/ai-eats-insurance/ accessed November 2018.
- Schreiber, Precision Underwriting (above n3), https://www.lemonade.com/blog/precision-underwriting/ accessed November 2018.
- S. Mendelez. Insurers turn to artificial intelligence in war on fraud, Mind and Machine Blog, Fast Company, 26 June 2018. https://www.fastcompany.com/40585373/to-combat-fraud-insurers-turn-to-artificial-intelligence accessed November 2018.
- S. Wininger (co-founder at Lemonade). How do insurance companies use machine learning?, Quora, August 8, 2017 https://www.quora.com/How-do-insurance-companies-use-machine-learning accessed November 2018.
- The Economist, A New York startup shakes up the insurance business, March 9, 2017 https://www.economist.com/finance-and-economics/2017/03/09/a-new-york-startup-shakes-up-the-insurance-business accessed November 2018.
- M. Carbone and A. Jones. Bigger and Redder: A Look at Q1 ’18 for Lemonade, Other InsurTech Carriers, Carrier Management, June 15, 2018, https://www.carriermanagement.com/features/2018/06/15/180464.htm accessed November 2018.
- Intelligent Insurer, Lemonade to slow down growth and tackle loss ratio, June 21, 2018, https://www.intelligentinsurer.com/news/lemonade-to-slow-down-growth-and-tackle-loss-ratio-15644 accessed November 2018.
- Economist, A New York startup shakes up the insurance business (above n9), https://www.economist.com/finance-and-economics/2017/03/09/a-new-york-startup-shakes-up-the-insurance-business accessed November 2018.
- G. Noto. Lemonade Insurance Opens its API Platform to Developers, Bank Innovation, October 11, 2017, https://bankinnovation.net/2017/10/lemonade-insurance-opens-its-api-platform-to-developers/ accessed November 2018.
- M. McKenney. What is Unfair Discrimination in General Insurance Rating? A Regulator’s Perspective, Society of Actuaries, December 2016, https://www.soa.org/News-and-Publications/Newsletters/General-Insurance/2016/december/What-is-Unfair-Discrimination-in-General-Insurance-Rating–A-Regulator-s-Perspective.aspx accessed November 2018.
This is a fantastic summary of how an innovative company is leveraging machine learning in all aspects of its business. I actually worked at Lemonade for 5 months prior to starting work at HBS, and frankly, I could not have done a better job summarizing this company’s approach to AI and the many ways it can be used to reduce fraud, quantify risk and cut administrative costs. The author also does a fantastic job exploring the potential synergies between insurance data and the IoT. I would actually encourage the author to view those synergies from two sides:
1. The insurance company acquires data on the behaviors and risk profile of an individual and their home (Pricing Risk)
2. The insurance company could take steps to change behaviors and mitigate risks- informing and encouraging the use of better alarm, flood or electrical systems (Mitigating Risk)
I do want to raise one potential area of concern. While Lemonade collects a ‘wide’ range of customer data, how much flexibility do they have in terms of pricing their customer? Are they allowed to charge customers more based on any data points they may have collected from social media? Where do privacy concerns come into play? The answer to this question is, of course, that it is highly complex. Insurance is regulated in the United States on a state level, with each state possessing its own rules about what are and are not appropriate criteria for underwriting and pricing. But the author is certainly right that Lemonade is looking to innovate in the space and that the potential payoff is tremendous!
Thanks,
Gabe
Really enjoyed the article over how machine learning and big data have the potential to revolutionize the insurance industry. One important dynamic to consider is the cost of customer acquisition and how to effectively market this product. How will lemonade attain a significant market share in an industry that has traditionally been very relationship focused. Can lemonade market itself as the most efficient insurance provider and thus give consumers more transparency into the process without aggravating privacy concerns? Also, separating causation and correlation seems difficult when dealing with such large data sets. As you mentioned, providing accurate risk estimates based on good data analysis and subsequently better premiums is something all insurance providers will start doing with AI. Will lemonades first mover advantage be sufficient to capture market share?