Machine Learning at YouTube: Removing Abusive Content
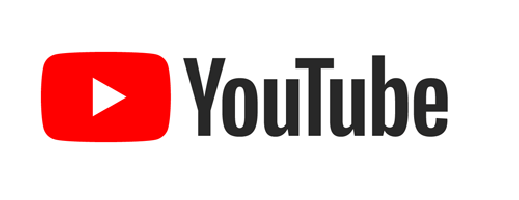
YouTube has become the world’s foremost video-sharing website. Recently, it has faced criticism for the proliferation of abusive content on its platform. How can YouTube use machine learning to identify and remove such content to create a safer environment for its users?
In the first half of 2018, nearly 18 million videos were removed from YouTube, the world’s foremost video-sharing website[1]. Of those 18 million videos, 79% were identified by automated systems created by the company to identify content that violates YouTube’s Community Guidelines[2]. Susan Wojcicki, CEO of YouTube, acknowledged in an official statement that while YouTube’s “open platform has been a force for creativity, learning, and access to information”, it has also left the company vulnerable to “bad actors exploiting our openness to mislead, manipulate, harass or even harm” YouTube’s community[3].
Combating the proliferation of abusive content is critical to YouTube’s business model. In a recent New York Times article, parents protested the site, claiming that “their children have been shown videos with well-known characters in violent or lewd situations and other clips with disturbing imagery, sometimes set to nursery rhymes”[4]. Adult users have also expressed frustration. As news of YouTube’s popularity with white supremacy groups and other organizations prone to using hate speech has emerged, the average user is left to question their ability to engage with the platform in a safe way. Only 4% of respondents contacted by Business Insider Intelligence’s 2017 Digital Trust survey felt that YouTube was the safest social media platform to participate in, ranking it last amongst the various platforms, and 44% behind the leader, LinkedIn, and 15% behind its lowest competitor, Twitter[5]. Even advertisers are unhappy, frequently severing relationships with YouTube upon learning that their advertisements are displayed next to inappropriate content. In recent years, YouTube and other technology companies have also garnered the attention of national governments. Global leaders have expressed concern about the platform’s ability to monitor its content effectively, particularly as it relates to national security, and UK Prime Minister Theresa May even accused YouTube and other technology companies of providing “safe spaces” for extremist groups and terrorist organizations.
In the short term, YouTube is taking action to purge their platform of problematic content and restore users’ trust. The company introduced YouTube Kids, which offers curated content and parental control settings to protect younger users. Additionally, videos are increasingly “age-gated”, granting access to only those users that are signed into Google accounts that indicate they are over a certain age. Perhaps the initiative with the most promise, however, is the introduction of machine learning. YouTube teaches machines to make decisions (whether or not to remove content) using training data (past examples of flagged content). Once the negative content is identified by the machines, human reviewers assess whether the video does in fact violate YouTube’s guidelines, and provide a recommendation as to whether the content should be removed from the site. Each video flagged, and subsequent human decision, then serve as data points for the machines, allowing the machines to better identify problematic content going forward.
Machine learning at YouTube has had significant initial success. The speed at which videos are identified and removed has increased dramatically – 77% of all videos removed from April to June 2018 due to machine learning were removed before they received a single view[6]. Susan Wojcicki estimates that machine learning is helping human reviewers remove nearly five times as many videos than they were previously able[7]. Certainly with continued human supervision and an increasing amount of data, YouTube will be able to further hone the effectiveness of the program. Unfortunately, unlike other companies like StichFix or Uber that have introduced machine learning to manage process improvement and/or product development with little notice or resistance from consumers, some users are actively trying to beat YouTube’s algorithms. When the use of slur words created red flags for YouTube’s algorithm, users began using “basketball Americans” to refer to African-Americans, “population replacement” to characterize white genocide conspiracies, and some even resorted to spelling words using numbers to avoid being caught[8]. We know that “algorithms draw their power from being able to compare new cases to a large database of similar cases from the past”, but for YouTube, past examples will only remain relevant until users learn not to repeat them[9].
Perhaps YouTube could adopt more rigorous screening processes prior to upload. This would eliminate users’ ability to livestream, but if YouTube waits to screen videos once they are live, are they destined to be one step behind their bad actor users? Alternatively, YouTube could consider creating signals for its algorithms that are not inherent to the actual videos being reviewed (e.g. has the user uploaded abusive content before?). In doing so, the company must strike the right balance of human / technical interaction to avoid the impact of human biases. For example, predictive policing has the potential to exacerbate human biases, might the use of machine learning at YouTube to identify extremist groups have similar unintended consequences?
(795 words)
[1] Google, “Transparency Report,”https://transparencyreport.google.com/youtube-policy/overview?content_by_flag=period:Y2018Q2;exclude_automated:&lu=content_by_flag, accessed November 2018.
[2] Ibid.
[3] Susan Wojcicki, “Expanding our Work Against Abuse of our Platform,” Broadcast Yourself (blog), YouTube, December 4, 2017, https://youtube.googleblog.com/2017/12/expanding-our-work-against-abuse-of-our.html, accessed November 2018.
[4] Sapna Maheshwair, “On YouTube Kids, Startling Videos Slip Past Filters”, The New York Times, November 4, 2017. https://www.nytimes.com/2017/11/04/business/media/youtube-kids-paw-patrol.html, accessed November 2018.
[5] Dylan Mortensen, “Marketers Prefer Social to YouTube for Digital Video Campaigns,” Business Insider Intelligence, August 12, 2016. https://intelligence.businessinsider.com/post/marketers-prefer-social-to-youtube-for-digital-video-campaigns-2016-8, accessed November 2018.
[6] Google, “Transparency Report,”https://transparencyreport.google.com/youtube-policy/overview?content_by_flag=period:Y2018Q2;exclude_automated:&lu=content_by_flag, accessed November 2018.
[7] Susan Wojcicki, “Expanding our Work Against Abuse of our Platform,” Broadcast Yourself (blog), YouTube, December 4, 2017, https://youtube.googleblog.com/2017/12/expanding-our-work-against-abuse-of-our.html, accessed November 2018.
[8] Yoree Koh, “Hate Speech on Live ‘Super Chats’ Tests YouTube,” The Wall Street Journal, November 2, 2018. https://www.wsj.com/articles/hate-speech-on-live-super-chats-tests-youtube-1541205849?mod=searchresults&page=1&pos=2, accessed November 2018.
[9] Mike Yeomans, “What Every Manager Should Know About Machine Learning”, Harvard Business Review Digital Articles, July 7, 2015, pp. 2–6.
Great article that accurately reflects the struggle we faced at YouTube. With over 400 hours of content uploaded every minute, it’s virtually impossible for humans to manually review and remove videos that don’t meet community guidelines. That’s where machines have to step in and offer a helping hand – sifting through videos pinpointing ones that should be removed and escalating ones that need further review by a human. A great example of machines and humans working together.
As with the scale of videos uploaded, livestreams offers an additional challenge. In my view, it would be possible to apply a similar approach to on-demand videos on live videos with the caveat that the live videos may need to have a time lag. This time lag would allow the video to be constantly screened by YouTube’s systems to ensure community guidelines are being met. There are further protections that could be put in place, such as earning the trust of the platform before having the ability to livestream. This could be in the form of a ‘clean history’ or a sizable subscriber base that indicates some level of credibility.
Finally, we must not forget the power of the community in helping police platforms. With the right culture and systems in place, users on YouTube can be empowered to contribute to actively policing the platform and flagging videos for review when needed. On top of supporting with policing, it would also feed the ML algorithms very helpful training data to help finetune it’s video-spotting capabilities.
Very interesting take on how YouTube is grappling with the limitations of machine learning as a tool to filter inappropriate content. I am impressed by the success of machine learning in removing this content before it hits viewers’ eyes. However, not mentioned is the implications of this for freedom of speech. While YouTube can and should be accountable for policing harmful content, if its algorithm results in the over-blocking of content, including content incorrectly construed as offensive (assuming this happens) it could compromise the openness of the platform. I’m curious to see how YouTube is able to refine its algorithm to capture bad content and code it to pick up on the workarounds described, while not over censoring uploads.