GM and Machine Learning Augmented Design
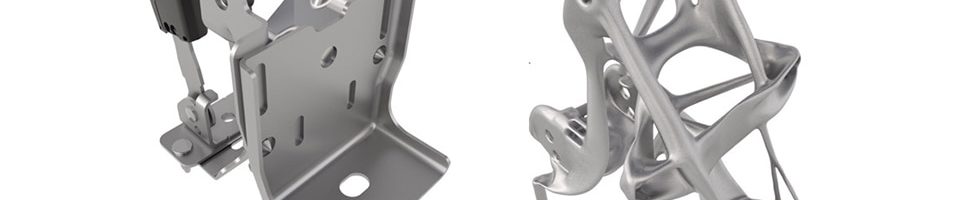
AI-driven product development may help GM find an edge in an industry that has increasingly trended towards conformity in the face of uncertainty. Can GM use machine learning to transform from a company hedging its risk by dabbling in everything new – to a company that thrives on compressed, cost effective design cycles with measurable results?
AI-driven product development may help GM find an edge in an industry that has increasingly trended towards conformity in the face of uncertainty. Can GM use machine learning to transform from a company hedging its risk by dabbling in everything new – to a company that thrives on compressed, cost effective design cycles with measurable results?
GM suffers from pressures that face the entire automotive industry. The effective price growth of the average vehicle has remained flat since 1998.[7] Despite this, regulatory pressure, consumer demands, and a fractured technology landscape have increased cost pressure and thinned margins for GM.[7] Less cash is available for R&D in an industry that increasingly demands innovation to define its future.
Overall, the automotive industry currently faces its greatest period of uncertainty. Several macro trends are seated to permanently disrupt the marketplace. Consumer tastes could substantially shift to electric vehicles – disincentivizing OEMs (original equipment manufacturers) from investing in improving traditional drivetrain tech, while overall vehicle ownership could shift from individuals to rideshare.[8]
In face of these uncertainties automakers have trended towards platform-based innovation to economize – fielding core vehicle platforms with infinite numbers of small variations in appearance to serve different global markets.[7] In short, real performance-driven innovation is scarce, margin to fund it is scarcer, and risk of investment higher than ever.
Machine learning can provide a needed edge in an increasingly risky, innovation starved product development environment. We have all encountered natural speech powered AI assistants, data analysis, and process automating AI, but increasingly machine learning is trending towards design process “amplification.”[6]
Machine learning amplified design can help reduce the cost of new product design, compress design cycles, and provide a creative edge that will help GM break free from platform-driven innovation by reducing R&D risk.[5]
How does it work? One new application integrates machine learning into computer aided design (CAD). This process has been coined generative design. The application GM is experimenting with: Autodesk’s Dreamcatcher, procedurally generates dozens of plausible designs of a given part based on a series of inputs such as required weight, strength, size, material, cost, or other performance requirements.[4] These plausible designs can be sent directly to machining to generate test parts. Real performance data of the parts can be fed back into Dreamcatcher to optimize the program’s understanding of the performance implications of its design choices. What would normally take a designer several days to create a single design, can now be done in hours with multiple viable alternatives. The more parts that are designed and analyzed with dreamcatcher, the larger its knowledge base of performance outcomes – allowing it to cross-leverage knowledge from multiple different classes of parts.[1][4]
Currently, GM has only experimented with simple, non-drivetrain related applications. GM recently completed a pilot project to redesign a seatbelt bracket. The original part consisted of eight separate pieces. The dreamcatcher program generated 150 alternatives that were all a single piece, one of which proved to be 40% lighter and 20% stronger than the original eight-component design.[1][2][3]
Sample design iterations [9]
GM is currently using this technology to drive two design initiatives: lightweighting, and part consolidation. These two initiatives serve a strategy of improving fuel efficiency (lightweighting) and supply chain simplification (parts consolidation). In the near term, GM will use Dreamcather to drive these two strategies across all vehicles as part of a multiyear partnership.[5][3][1][2]
Unfortunately, the barrier to entry in lightweighting and parts consolidation in non-drivetrain componentry is low. To sustain its edge, GM has to shift into using machine learning to power generative design and rapid prototyping in higher value components. Naturally, drivetrain components have more performance variables and integrating performance results back into dreamcatcher as part of supervised learning is more difficult. Undertaking this task would however allow GM to develop a real asset – a bank of performance data attached to drivetrain-specific variables that is very difficult for other companies to replicate. Early targets could include relatively simple components such as valve covers and engine blocks while the long term could shift to moving parts such as crankshafts, pistons, and transmission componentry.
Given the proliferation of in-vehicle data generation, GM should focus on integrating live performance monitoring of higher-value componentry into its generative design process. Sensors could understand how engine componentry is performing under various situations. Data could also be generated as part of procedure during regular maintenance at dealerships – understanding how design choices on habitually maintained parts impact long-term reliability.
Overall, further investment in machine learning amplified design will help give GM the cost advantage needed to sustain real incremental innovation in a highly uncertain environment. It will do this not only by compressing the design lifecycle, but by de-risking experimentation in higher value componentry.
Finally, I question whether the insights produced by machine learning augmented design will be limited by traditional manufacturing techniques. Will the prototypes suggested by Dreamcatcher be too complex for mass production? (See photo above).[9]
Words: (809)
Sources:
[1] “Project Dreamcatcher | Autodesk Research”. 2018. Autodeskresearch.Com. https://autodeskresearch.com/projects/dreamcatcher.
[2] “Advanced Software Design Technology Leads GM To Lighter, More Efficient Vehicles”. 2018. Generalmotors.Green. https://www.generalmotors.green/product/public/us/en/GMGreen/home.detail.html/content/Pages/news/us/en/gm_green/2018/0518-more-efficient-vehicles.html.
[3] 2018. Gmsustainability.Com. https://www.gmsustainability.com/_pdf/downloads/GM_2017_SR.pdf.
[4]”Driving A Lighter, More Efficient Future Of Automotive Part Design”. 2018. Autodesk.Com. https://www.autodesk.com/customer-stories/general-motors-generative-design.
[5] Kvernvik, Marthe, and Marthe Kvernvik. 2018. “General Motors Applies Autodesk Generative Design Software To Develop Future Vehicles”. TCT Magazine. https://www.tctmagazine.com/3d-software-news/gm-teams-up-autodesk-generative-design-vehicle/.
[6] “How Humans And AI Are Working Together In 1,500 Companies”. 2018. Harvard Business Review. https://hbr.org/2018/07/collaborative-intelligence-humans-and-ai-are-joining-forces.
[7] 2018. Mckinsey.Com. https://www.mckinsey.com/~/media/mckinsey/dotcom/client_service/%20Automotive%20and%20Assembly/PDFs/McK_The_road_to_2020_and_beyond.%20ashx.
[8]”Where To Profit As Tech Transforms Mobility”. 2018. Https://Www.Bcg.Com. https://www.bcg.com/en-us/publications/2018/profit-tech-transforms-mobility.aspx.
[9]”Generative Design: The Road To Production”. 2018. Engineering.Com. https://www.engineering.com/DesignSoftware/DesignSoftwareArticles/ArticleID/16973/Generative-Design-The-Road-to-Production.aspx.
Great read, really liked the process of generative design, and how it allows the designer to generate multiple alternatives in a short duration, significantly improving the development cycle-time. What particularly interested me was the live-performance-monitoring recommendation. Installation of a variety of sensors on critical moving parts can help us analyse a host of outputs ranging from temperature, vibration, stress, speed etc. to send the signal back to the CAD model and update the generative design optimally. I recently read about the impact that real-time data monitoring through Internet-of-things technology via millions of sensors have created in the mining industry in terms of improved productivity. I believe that the same principle can be applied here in the field of ML-augmented design as well. The use of sensors, as correctly identified here, can be extended to enable predictive maintenance as well, thereby extending technical-life of componentry significantly.
Fascinating to read about the Dreamcatcher. Where I find this to be particularly interesting is if combined with 3-D printing to produce both test parts, and new single-piece parts which have so far not been feasible to manufacture. By opening up the design options to multiple other manufacturing options, there is scope to fundamentally alter the parts cost of a car, but more importantly to completely redesign previously unquestioned styles and facets of cars.
The question you posed regarding the viability of machine learning augmented design in relation to traditional manufacturing techniques is interesting. As mentioned in your paper, with smaller non drivetrain components prototyping via machine learning has been proven to increase efficiency and reduce costs. However, I feel that with larger more complex components creating the array of suggested prototypes for testing may pose challenging with traditional manufacturing techniques. The answer may rest with additive manufacturing or 3D printing. Prototype parts could be produced with 3D printers and tested. The final, selected part could then be mass produced. In fact, 3D printing companies like Stratasys have emerged to specifically “prototype, test, and produce all manners of tools, jigs, fixtures, and street-ready parts with unprecedented speed and efficiency” for the automotive industry [1]. While complex drivetrain printing technology could still be on the horizon, the technology is developing rapidly and could be a practical solution in the near future.
[1] “Automotive 3D Printing Solutions | Stratasys.” 3D Printing Solutions by Stratasys. Accessed November 15, 2018. http://www.stratasys.com/automotive.