Football and Chess: How Machine Learning Can Improve Playcalling in the NFL
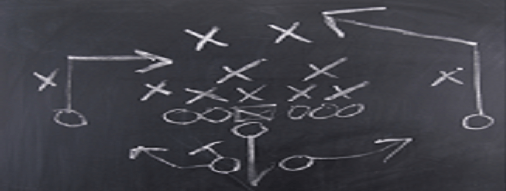
Many fans and analysts liken American football to a game of chess. Each team strives to stay one step ahead of its opponents, and the team with more talented players can lose any game if their gameplan is poor. With machine learning, the NFL can develop tools to help its teams optimize their in-game strategies, and put players in the best position for success.
A Chess Game?
Many fans and analysts liken American football to a game of chess. Each team strives to stay one step ahead of opponents through study of game films, opponent-specific playcalls, and in-game strategy adjustments. Given the comparison to chess, if IBM’s Deep Blue computer was able to outmaneuver a chess grandmaster, there must be an opportunity for machine learning to improve the playcalling process for the National Football League (NFL).
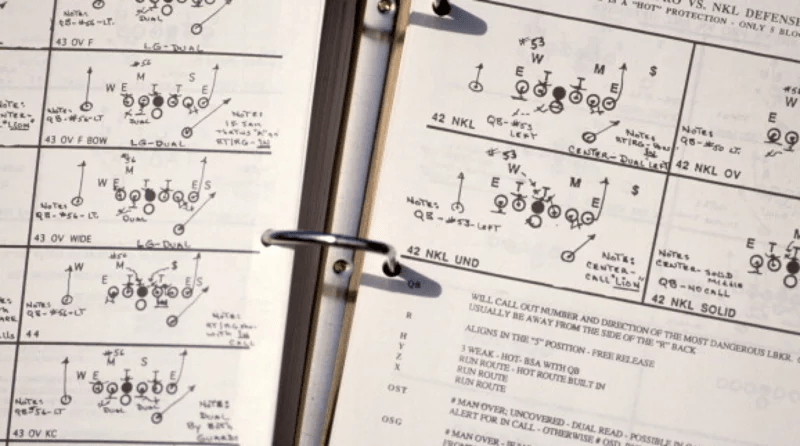
Playcalling Process
Before each football play takes place, coaches have a staggering number of ways to combine the personnel (which players are on the field), with formation (where players line up before the play starts), and concept (assignments for each of the eleven players once the play begins). Each playcall decision is selected to put the team’s players in the best position for success. During games, teams have 40 seconds for coaches to make the playcall decision, relay that information to players on the field, and for players to line up and begin the play. The current playcall process can be significantly improved through machine learning capabilities.
The ideal playcall varies depending on many factors including down, distance, ball location on the field, game time remaining, and the opponent’s playcall. Currently, many coaches refer to a “playsheet,” which categorizes preferred playcalls based on the ideal situations to choose them. Because of the 40 second time constraint, these playsheets contain only a small subset of possible playcalls and are not large enough to address every possible game situation. A machine learning algorithm that can rapidly provide an assortment of recommended playcalls based on the current game situation and the opponent’s expected playcall has the potential to greatly improve team performance.
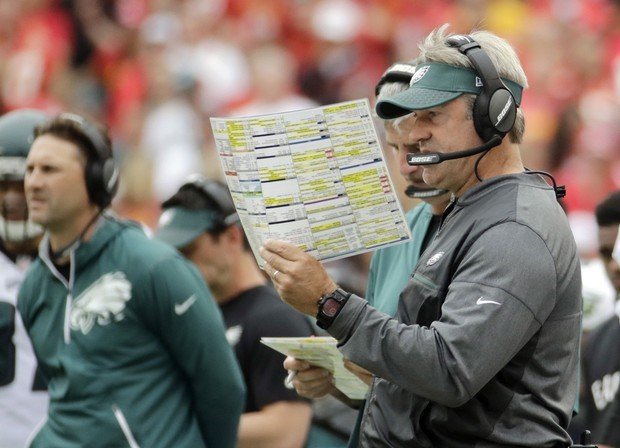
NFL’s Progress
In 2014, the NFL began a program called “Next Gen Stats,” aimed at uncovering deeper insights into on-field action, and improving the fan experience by providing a broader range of advanced statistics.[1] This program is tracking the data necessary to enhance in-game playcalling strategies, along with many other aspects of team operations such as training, fitness, and preparation.[2] Notable developments include adding location-tracking technology into players’ equipment and game balls,[3] a partnership with Amazon Web Services to host and analyze the 3TB of data generated from NFL games each week,[4][5] and a proprietary Next Gen Stats website for fans to learn about the league’s evolving data analysis capabilities.[6]
With respect to the use of machine learning for playcalling, the NFL currently provides each team with just its own data, and not that of its opponents. While many hope to eventually make all teams’ data available league-wide, the final decision lies with the NFL Competition Committee.[7][8] With data on one’s own team, a coach can determine if the team has shown any predictable tendencies or weaknesses that may be exploited by an opponent, but will not have data on opponents to help strategize for upcoming matchups or recommend situational playcalls. In the past, the NFL has made enhancements to the playcalling process by adding speakers to players’ helmets for live communication from coaches,[9] and providing on-field tablets to let teams review plays from earlier in the game.[10]
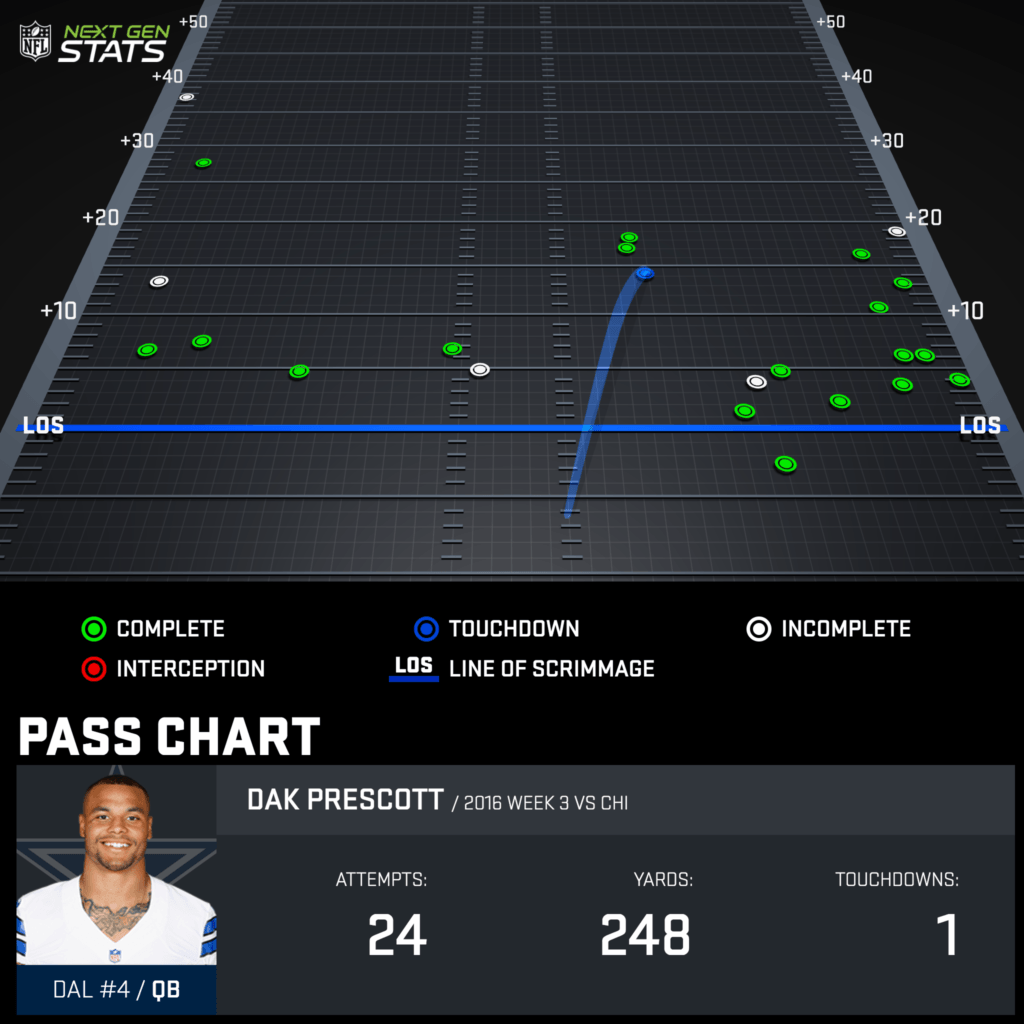
What’s Next
Given the NFL’s history of process improvement around playcalling, adding a machine learning recommendation capability would be a logical next step. This capability will allow teams to make more informed playcall selections and potentially increase the pace of the game, which fans would enjoy. One important factor to consider when utilizing machine learning recommendations is which players are on the field. For example, a certain play may have a very high success rate because it leverages the ability of one of the team’s most talented players; if this player is not on the field when that play is recommended, the likelihood of success may be significantly overestimated. Another consideration is how to weigh the importance of a playcall’s previous success against predictability. A machine learning algorithm will become more confident in a playcall that has been successful in the past, but eventually an opponent will markedly alter their strategy to defend against that playcall. It would be difficult to program a machine learning algorithm to foresee when that opponent’s adjustment will happen in real time and preemptively counter with a less predictable playcall.
Lastly, an important question this topic brings to light is related to the competitive nature of football. In the end, the NFL is a form of entertainment, and its major organizational decisions focus on whether they are improving the game for fans. Does a playcalling algorithm simply add another tool for coaches to use to make the league more competitive, or could automation lead to complacency by reducing the need for preparation and ultimately undercutting the coaching prowess that so many football fans currently admire?
(789 words)
References
[1] Press release: National football league selects AWS as official cloud and machine learning provider for next gen stats. (2017, Nov 29). Dow Jones Institutional News. Retrieved from ProQuest, accessed November 2018.
[2] Hiner, Jason, “How the NFL and Amazon unleashed ‘Next Gen Stats’ to grok football games,” TechRepublic, February 2, 2018, https://www.techrepublic.com/article/how-the-nfl-and-amazon-unleashed-next-gen-stats-to-grok-football-games/, accessed November 2018.
[3] “Zebra Technologies Collaborates with NFL and Wilson Sporting Goods to Deliver Unique Insights during 2017 Football Season,” Business Wire, Sep 07, 2017. Retrieved from ProQuest, accessed November 2018.
[4] Press Release: National Football League Selects AWS as Official Cloud and Machine Learning Provider for Next Gen Stats.”
[5] McKenna-Doyle, Michelle, NFL Chief Information Office, remarks made at re:Invent 2017 Conference, Las Vegas, NV. November 2017, https://www.youtube.com/watch?v=gjDLN3qJudA, accessed November 2018.
[6] https://nextgenstats.nfl.com
[7] Hiner, Jason, “How the NFL and Amazon unleashed ‘Next Gen Stats’ to grok football games,” TechRepublic.
[8] More information on the NFL Competition Committee available at https://operations.nfl.com/football-ops/league-governance/the-nfl-competition-committee/.
[9] “NFL installs new coach-to-defense communications system,” NFL press release, August 12, 2008 (updated July 26, 2012), http://www.nfl.com/news/story/09000d5d809f61c6/article/nfl-installs-new-coachtodefense-communications-system, accessed November 2018.
[10] NFL Football Operations, “SIDELINE OF THE FUTURE,” https://operations.nfl.com/the-game/technology/sideline-of-the-future/, accessed November 2018.
Is it an art or is it a science? This is a question has plagued professional sports since moneyball introduced advanced analytics. Your post raises many potential benefits for developing a better understanding of the scientific impact of different decisions throughout the game. It does make me wonder where we stop though. Does the game actually get better if we introduce machine learning, or, as you suggest, do players and coaches become either more complacent or too targeted in their preparation and decision making? Will we still sit on the edge of our seats watching the “Hail Mary” pass or will the game become so prescribed that it loses its authenticity? Personally, I think this poses great risks for recruiting as the metrics players are recruited on might not align with what it takes to be successful in the big leagues.
Thanks for sharing!
Thanks for the post Derek! If the NFL Competition Committee decides to release all the teams data, do you think that it will even out the competition across the league? It seems like machine learning in play calling has the potential to diminish the effects of skilled players and coaches. I wonder if you have any thoughts about how teams differentiate themselves in a future of automated play-calling. I’m also curious as to how college teams have embraced machine learning and the lag they have relative to the NFL. And lastly, I think the potential for machine learning in play-calling to make the sport safer is very interesting. I think the league has a real opportunity to analyze which plays lead to higher amounts of injuries/concussions that could potentially mitigate the recent negative press the NFL has received around safety issues.
Thanks for the post, Derek. As you suggest, it will be interesting to see how detailed data about specific players will influence our understanding of their strengths and weaknesses in implementing the plays that machine learning will suggest. For example, data about the physical strengths and limitations of players will imply a portfolio of physical strengths and weaknesses that might be more conducive to one play or another. Similarly, data could be used to monitor player vitals on a regular basis, so that these strengths and weaknesses are dynamic — they may change over time, which would thus impact which plays are or are not affected. This might also impact which players are best fit for a specific strategy, given those health profiles.
Very intriguing post Derek. Can we expect to see this next year in intramural football? Jokes aside, I too am hesitant for the league to provide other team’s data to each other. I question where will it stop? I’d love to understand the algorithm’s deployed here a little better, and see how we could improve them. If I took this a step further (given the amount of data being tracked today), I’d love to incorporate as much athlete data into the to formulas as possible. Specifically fitness levels and current playing time to predict current performance levels. Would also like to overlay situational tendencies to further aid in selecting the “best” call.
This was a very thought provoking and interesting post (one would assume you were a college quarterback). You bring up a very plausible application for machine learning not only within the NFL for play calling but more broadly across professional sports. As an avid sports fan however, I am very weary of the implication that this potential technology may have on sports, which you have referred to as a source of entertainment. First, I believe it would be a shame if teams started winning or having an advantage due to a more efficient or innovative algorithm taking away the joy of the game. Second, if this was allowed I am unsure whether or not machine learning would be able to ‘add’ too much to the play calling approach and ultimately producing better results. The reason is that these are humans executing on the plays that were provided them which adds several variables from effort, speed, field conditions, weather, etc. not machines following a standard set of actions similar to chess.
Great article, Derek! I was unaware that the league is providing teams with these massive amounts of game data. I had also never considered how machine learning could be applied in this context. The possible advantages that you outlined are huge. I found your point regarding the teams having each other’s data to be a very critical one. In my opinion, if the league begins to serve each team this information then the competitive dynamics will shift dramatically. Game plans would be tailored directly to statistical outcomes rather than the creative, awe-striking plays we have come to love. I am all for teams gathering intelligence/data on opponents but I think this would be taking it a bit too far. I am also curious – as a former quarterback yourself, how do you think of the differences between machine-learning-enabled play calling versus a player’s intuition to audible to the best possible play?
Thanks for the article, mate. I will also be interested to see how the increasing rollout of machine learning technology affects competition, both in sport and in other fields–it brings to mind comparisons to the introduction of game theory to commercial and strategic decision-making in the 60s and 70s.
I also agree with your point that algorithms are limited in their ability to predict future plays based on historical data, but I suspect that the consequence of that limitation is that the ideal decision-making unit will be a combination of both human and machine intelligence. Given that inputs are forever-changing (e.g. the coach may sense quarterback is simply having an off day) and opponents are responding to a team’s decisions in real-time, I can’t see a world where the judgment fans have come to value so highly is not still being exercised. That being said, it’s almost certainly going to have to be the next generation of coaches who bring this skillset to life–I have a hard time believing that old dogs will be able to learn drastically new tricks.
Awesome post, thanks Derek! It reminds me of “playing the computer” in Madden. It would be interesting to see if the data could be used to create a QBR-esque metric to rank coaches and coordinators based on their play-calling. This would be highly unfavorable by the coaching community but might be entertaining to fans. I am consistently impressed by how great coaching can often beat great talent and would love to see stats behind the media-hyped play-calling geniuses like McVay and Arians.