Farmers Business Network: Democratizing agriculture using machine learning
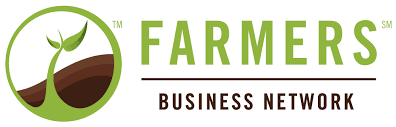
Farmers Business Network (FBN), a HBS grad-founded startup, is disrupting the agriculture sector by crowd-sourcing farmer data. FBN has managed to apply machine learning techniques to aggregated farmer data to generate actionable insights around input use and crop marketing for farmers.
There are more than 2 million farms in the US. Family farms make up 99% of all US farms, and account for over 90% of production[i]. This level of fragmentation stands in stark contrast to the consolidated nature of the upstream and downstream market. On the commodity trading side, a couple of buyers including Cargill and Archer Daniels Midlands dominate the market. Meanwhile, consolidation has resulted in a similarly small number of input suppliers like DuPont and Bayer controlling the input market[ii]. This results in an information asymmetry that leaves farmers squeezed in the middle.
FBN was founded to equalize the playing field by applying machine learning to an increasingly rich set of farmer data to provide farmers with insights on input choices and crop marketing. Over the past years, farm equipment has become adept at collecting data[iii]. For example, tractors collect data on seed, pesticide and fertilizer application, thus improving farmers’ ability to apply exactly the right amount of inputs to maximize yield. But data from one individual farm is of limited value[iv].
The value proposition of FBN is in aggregating data from large groups of geographically diverse farms to generate accurate predictions around input use, agricultural practices, and crop marketing. Farmers willingly share this data with FBN, in exchange for access to the analytics platform[v]. Importantly, this is only possible because farmers trust FBN and see it as an independent company separate from the existing oligopolistic ecosystem of agribusinesses. Over time, the FBN platform has leveraged machine learning to become increasingly accurate in predicting patterns and associations within agricultural data. For example, as the platform receives additional data on how a specific agricultural input like fertilizer or seed performs under certain geographic, weather, and soil conditions, it becomes more and more accurate at providing recommendations to farmers that face those conditions.
Short-term, management has designed a business model to leverage the value of machine learning by focusing on network effects among farmer data. Increasing the number of farms has non-linear benefits in improving their machine learning algorithms. FBN’s short-term strategy is to quickly get to scale to improve their value proposition. They’ve done so by leveraging a low, fixed-cost subscription fee model (at only $600 per year, regardless of the size of the farm) that has seen them enjoy rapid growth[vi]. By the end of 2016, FBN had data on more than 55 million acre-events (crops planted on an acre of land in one crop cycle).
Long-term, the company sees itself as a one-stop shop for input purchasing and crop marketing, thus potentially cutting out the input suppliers and commodity traders. By leveraging machine learning insights from the rich dataset that FBN has access to they can effectively sell inputs (since they know what will work best) and market crops to buyers (since they can provide full traceability and predict supply better than competitors). Still, there is a potential conflict of interest to navigate here. FBN relies on farmer trust to thrive, but if farmers believe FBN is promoting inputs that are more profitable for FBN, this trust will quickly erode. For this reason, it will be critical to focus on transparency in input sales and crop marketing.
Short-term, FBN needs to focused on building out customer trust, developing new products, and simplifying existing ones. Behavior change, in particular among farmers, is not always easy. Farmers that have already joined the platform are early adopters. It is likely that the marginal customer acquisition cost will increase, and ensuring that the platform is easy to use will be critical as less technologically adept farmers join (especially as they seek to expand outside of the US). Long-term, FBN needs to focus on hiring and training skilled data scientists to leverage their competitive advantage and exploring potential large-scale changes in agricultural business models (e.g. offering farmers financing and purchasing commitments based on data-driven insights) that can allow FBN to further disrupt the agriculture industry.
As FBN considers how to further leverage machine learning in its product development, there are several questions to consider:
- How can FBN continue to grow its product offerings using crowd-sourced data while still maintaining farmer trust?
- To what end should the FBN platform be prescriptive (e.g. here is what the algorithm recommends for you) versus simply providing farmers with the tools to generate their own insights?
Word Count: 721
Citations
[i] https://www.ers.usda.gov/data-products/ag-and-food-statistics-charting-the-essentials/farming-and-farm-income/
[ii] https://www.ers.usda.gov/amber-waves/2017/april/mergers-and-competition-in-seed-and-agricultural-chemical-markets/
[iii] Precision Agriculture Technology and Robotics for Good Agricultural Practices. IFAC Proceedings Volumes, Volume 46, Issue 4, 2013, Pages 1-4. Josse De Baerdemaeker
[iv] Liakos, K.; Busato, P.; Moshou, D.; Pearson, S.; Bochtis, D. Machine Learning in Agriculture: A Review. Sensors 2018, 18, 2674.
[v] HBS Case 9-217-025 “Farmers Business Network: Putting Farmers First”, by Prof. Shawn Cole and Tony L. He.
While I agree that building trust and transparency are fundamental building blocks in pursuing network effects through machine learning, I would argue that focusing on expanding farmer education and awareness is more critical to FBN’s growth at this point in time. In such a fragmented market, farmer’s are already yearning for a competitive edge in pricing against the big input sellers. However, to realistically go up against the likes of DowDuPont and Monsanto, FBN needs more critical mass to compete and incentivize manufacturers of generic products to switch to FBN (https://www.forbes.com/sites/amyfeldman/2018/06/19/farming-ag-agriculture-farmers-business-network/#7ba563166312). Therefore, FBN should invest more heavily in promoting their mission. While potentially costly as the post alludes to, this marketing will have a bigger impact on their ability to scale effectively through machine learning given the traction FBN has already gained in the market. Thus, the question centers less around maintaining trust with existing farmer members and more around how to accelerate word of mouth amongst new farmers to gain the necessary clientele population to compete.
With regards to the first question it seems FBN will continue to build farmer trust — and therefore scale their crowd-sourced data further — by maintaining their commitment to their core value of putting farmers first (https://www.fbn.com/about#farmer-experience). Their commitment to this value drives internal company decisions from seed pricing to launching new business lines e.g. crop marketing. Where they may be put in a position to think more deeply about what farmers first really means is when they may choose to diversify their revenue streams in the future. As a growing business they may face choices along the lines of whether to provide data to suppliers and manufacturers further upstream. While this could provide revenue opportunities for them they’d also need to balance their commitment to maintaining farmer trust.
In terms of question two — FBN currently provides farmers with relevant data but they do not provide recommendations to the farmer based on this data. In addition to data transparency, FBN is also committed to providing unbiased and independent information to farmers. FBN will need to weigh the incremental benefit to farmers by providing them prescriptive recommendations against the cost of any perceived bias or loss of independence (based on an interview with an FBN employee).
Thanks for sharing!