Extreme Makeover Home Edition: Wayfair Optimizes Your Home Décor Shopping Experience Through Machine Learning
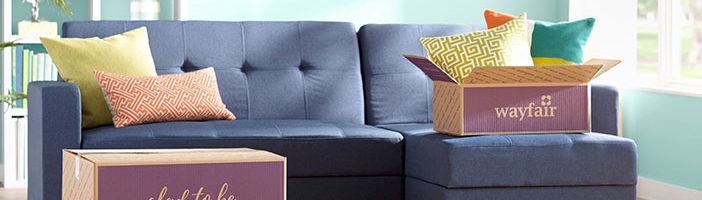
Though many shoppers view Wayfair as “that online furniture store,” Wayfair identifies as a technology company that “reinvents the way people shop for their homes.”[1] In an information-abundant age, customers are king, and with a few keystrokes gain access to the world’s markets. Sellers, then, face fierce competition for clicks, carts, and conversions. At Wayfair, machine learning is central to improving the customer experience and driving sales. Specifically, Wayfair employs machine learning at the top of the sales funnel to optimize product listings, better matching results to customers’ intent and historical preferences, as well as eliminating duplicative listings.
Since customers rarely browse beyond the first page of search results, Wayfair employs machine learning to surface products customers are most likely to select. “If customers can’t find something easily, they are probably not going to buy it,” says software engineer Suyash Sonawane.”[2] The first tool, Wayfair’s Query Intent Engine, breaks down words in a customer’s query to determine their true meaning (for example, whether “glass” refers to a material or a product type), then maps the meaning against known attributes, and lastly directs the Search Engine to show products whose attributes match the query. In addition to product data provided by suppliers, the algorithm uses historical click data to learn what products have met the needs of customers with similar queries.[3]
A second machine learning algorithm, the Product Reranker, builds on the Query Intent Engine. The reranker optimizes results for a specific outcome like “Add to Cart”; it takes items deemed “relevant” by models like the intent engine and ranks them according to their likelihood of delivering the desired metric. [4] Wayfair also deploys the reranker outside of search, including on customized landing pages and remarketing newsletters. As both algorithms receive new data every day, they build on themselves and each other. Looking ahead, Wayfair aims to make better use of product reviews, which often reveal attributes not already provided by the supplier, as inputs to these models.[5]
This diagram outlines how various data inputs inform what product listings are returned to a user. Source: Suyash Sonawane, “How We Use Machine Learning and Natural Language Processing to Empower Search.”
Wayfair also leverages machine learning to improve the search experience by eliminating duplicative listings. Since Wayfair does not own inventory and instead acts as a platform connecting suppliers with customers, it does not control product listings at their inception. Unique suppliers often list identical products at different prices or under different names, creating confusion for customers.[6] In response, Wayfair uses a Duplicates Review Tool to consolidate listings. This algorithm compares product attributes (like weight, manufacturer, and number of parts) to calculate a “similarity metric” for a pair of items. Pairs with a high-enough score are then reviewed by a human and consolidated if the algorithm correctly identified a duplicate.[7] For greater impact, Wayfair hopes to increase the number of attributes fed into the model and deploy it earlier in a product listing’s lifecycle so that duplicates never hit a consumer-facing catalog.[8]
As a data-rich e-commerce destination, Wayfair already leverages machine learning to reduce friction in customers’ shopping experiences. But to further address the issues discussed, Wayfair will need to increase the speed at which it integrates data. With its customer and supplier counts growing daily, Wayfair must deploy algorithms more quickly to prevent data processing from becoming a bottleneck (at the current pace, it takes some algorithms three weeks to cycle through the catalog).[9] In addition, the firm should be wary of asking too many teams to pilot machine learning solutions simultaneously. A common problem among growing technology companies, this can lead to overlapping work and algorithms interfering with one another on site.
A question readers can consider when evaluating machine learning at Wayfair is whether these algorithms should be fiercely protected or, conversely, commercialized. Advertisers already partner with Wayfair to leverage their data for targeted advertising, but would the value of Wayfair’s machine learning tools ever be such that Wayfair stands more to gain from commercializing the predictions than from than protecting them as a competitive advantage? This shift would make clear that Wayfair realizes its identity as a technology company, rather than a digital furniture store.
(784/800)
References
[1] “About Wayfair.” Wayfair. Accessed November 12, 2018. https://www.wayfair.com/about/.
[2] Sonawane, Suyash. “How We Use Machine Learning and Natural Language Processing to Empower Search.” Wayfair Tech Blog. October 15, 2018. Accessed November 11, 2018. https://tech.wayfair.com/2018/10/how-we-use-machine-learning-and-natural-language-processing-to-empower-search/.
[3] Sonawane, “How we Use Machine Learning and Natural Language Processing to Empower Search.”
[4] Kirkwood, Rachel. “Information Retrieval and Machine Learning at Wayfair.” Wayfair Tech Blog. July 6, 2018. Accessed November 11, 2018. https://tech.wayfair.com/2018/07/information-retrieval-and-machine-learning-at-wayfair/.
[5] Sonawane, “How we Use Machine Learning and Natural Language Processing to Empower Search.”
[6] Maunu, Ryan. “The Duplicate Review Tool: Incorporating Visual Search into Merchandising Operations – Wayfair Tech Blog.” Wayfair Tech Blog. May 25, 2018. Accessed November 11, 2018. https://tech.wayfair.com/2018/05/the-duplicate-review-tool-incorporating-visual-search-into-merchandising-operations/.
[7] Manu, “The Duplicate Review Tool.”
[8] Manu, “The Duplicate Review Tool.”
[9] Manu, “The Duplicate Review Tool.”
I am curious how effective the duplicate review tool is at identifying duplicate postings of an item. It seems that this tool is heavily dependent on the data entered by the user and therefore its effectiveness could be limited. One way to overcome this obstacle is require certain data fields to be entered before a user can list an item. This would ensure identification of duplicates and also improve the strength of the product catalog. Additionally, what kind of communication does Wayfair send to a seller before the postings are consolidated? The seller might have a preference on which price is taken for a duplicate listing or have legitimate reason for posting an item twice. Since Wayfair is a marketplace, I think it should be up to the sellers discretion how many times an item is listed.