Don’t Give Up the Ship: A Smarter Approach to Maintenance in the US Navy
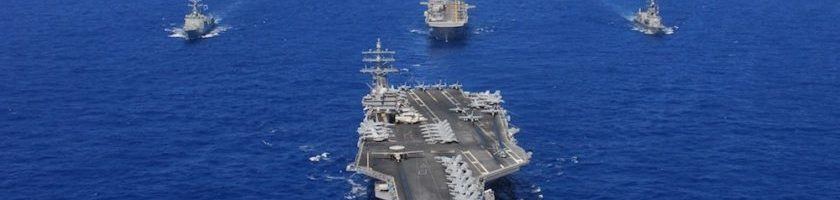
The US Navy's antiquated maintenance system must evolve into a conditions-based system enhanced by AI.
“There has to be a better way.” This was a common phrase among my Sailors on USS Laboon, a guided missile destroyer in the US Navy. Every week the 23 Sailors in my division completed hundreds of hours of maintenance. This maintenance, however, was based on an antiquated system that relied upon time-based maintenance rather than the conditions-based maintenance (CBM) increasingly used in the commercial sector. When combined with internet of things (IOT) sensors and artificial intelligence (AI), CBM has the potential to better predict and avoid machine failure, dramatically reducing equipment downtime and maintenance costs [1].
The Navy’s current maintenance system relies heavily on time-based maintenance. While there are some systems, like propulsion, that use a primitive version of CBM, this is the exception rather than the norm. The current time-based system might require a technician to replace an air conditioner filter every 90-days. This process has two primary issues. First, the filter could fail in less than 90-days, causing damage to the equipment. Second, the filter may not need to be changed after 90-days, in which case the technician has wasted an hour of time that could have been spent elsewhere. This is particularly problematic on ships like the Littoral Combat Ship (LCS) and the Zumwalt Destroyer Class that rely on minimally-manned crews augmented by automated systems.
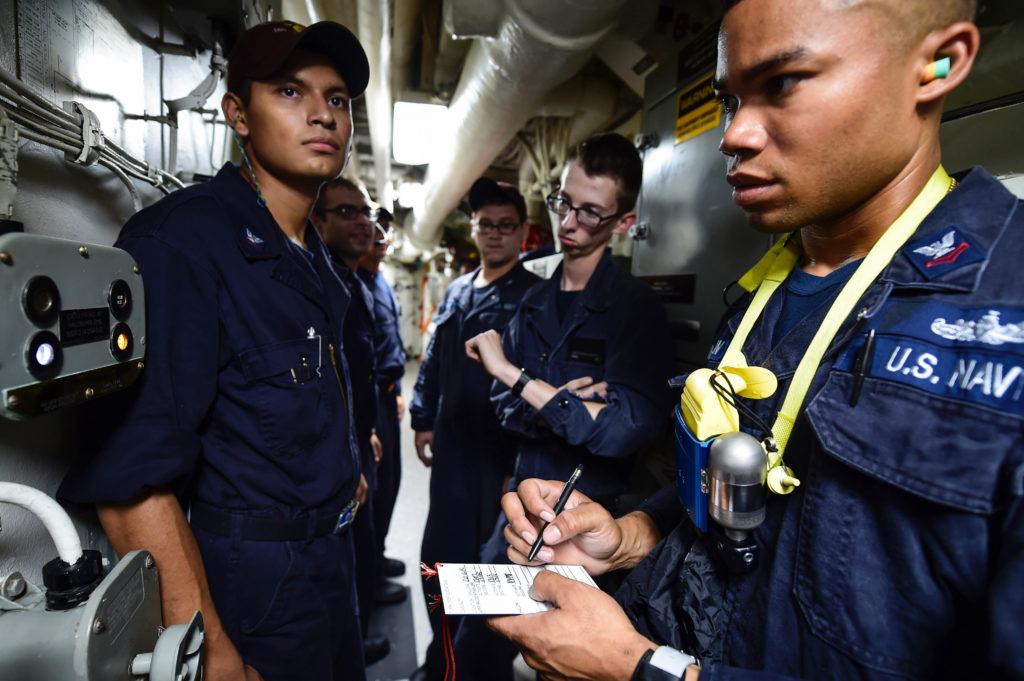
MEDITERRANEAN SEA (Aug. 6, 2016) Gas Turbine Systems Technician (Mechanical) 2nd Class Alvah Johnson, from Baton Rouge, Louisiana, monitors the simulated halon run time during an engineering training team evolution aboard USS Ross (DDG 71). Ross, an Arleigh Burke-class guided-missile destroyer, forward-deployed to Rota, Spain, is conducting naval operations in the U.S. 6th Fleet Area of Operations in support of U.S. national security interests in Europe and Africa. (U.S. Navy photo by Mass Communication Specialist 1st Class Theron J. Godbold/Released)
CBM, on the other hand, is performed after one or more indicators show that equipment is going to fail or that equipment performance is deteriorating. CBM is particularly impactful when combined with IOT and AI applications. For example, a remote sensor could be placed behind the filter to detect flow rate. This sensor could feed the flow rate data into a central repository where it could be aggregated with other sensor data and routed through an AI algorithm. The algorithm could determine a) if the filter needs to be replaced and b) if the combination of the flow rate data and other sensor data from the AC indicates a more significant issue with the equipment as a whole. Over time, as the algorithm digests greater amounts of data it can, through reinforced learning, create more accurate predictions.
The military is not blind to the importance of smarter maintenance. The Department of Defense has invested heavily in Defense Innovation Unit Experimental (DIUx) [2], a DOD-funded venture capital unit, which has made machine learning for predictive maintenance a priority [3]. In June the group awarded $1mm to Uptake, a Chicago-based startup that has partnered with companies including Boeing and Caterpillar [4]. The contract is to pilot a CBM program for the Army’s Bradley Fighting Vehicle. In addition, Sea Systems Command (NAVSEA), the Navy command responsible for engineering and building Navy ships, has begun to incorporate a broader array of equipment sensors that will inform future CBM systems [5].
While these are certainly steps in the right direction, the Navy cannot proceed at the glacial pace that often characterizes change in large bureaucracies. To that end, the Navy should do three things. First, the private sector is successfully utilizing AI-enhanced maintenance programs and the Navy should take advantage of the work that has already been done. Specifically, it must dramatically expand the number of pilot programs with tech organizations like Uptake. Second, it must eliminate (or atleast reduce) the red tape that often prevents private sector organizations from partnering with the military. Finally, the Navy must ensure that lessons learned in the other branches inform its own progress. For example, any successes or failures associated with the Bradley Fighting Vehicle program must influence future Navy projects. Time is too short to repeat failures.
What Comes Next?
As the Navy moves toward an AI-enhanced CBM model there are critical questions that must be answered. Will information security and cyber security concerns outweigh the benefits of this new system? Are there other elements of private-sector maintenance systems that should be incorporated? Will recent military-related protests at Google [6] lead other private sector organizations (which are vital to the future of this model) to abandon their work with the DOD?
(Word Count: 798)
[1] Smartening Up With Artificial Intelligence (AI)- What’s in it for Germany and its Industrial Sector. (2017). Retrieved November 11, 2018, from www.mckinsey.com
[2] Cornillie, Chris. “Rump Embraces Obama’s ‘Venture Capital Firm’ for Pentagon Tech.” Bloomberg Government, 21 Feb. 2018, about.bgov.com.
[3] Defense Innovation Unit Experimental (DIUx) Annual Report. (2017). Retrieved November 11, 2018, from https://diux.mil/download
[4] Greg, A. (2018, June 26). Army to use artificial intelligence to predict which vehicles will break down. Washington Post. Retrieved November 11, 2018, from www.washingtonpost.com
[5] Graffius, R., & Gaffney, R. (2016, Fall). Changing How the Navy Schedules Maintenance. Surface Warfare Magazine. Retrieved November 11, 2018, from https://www.public.navy.mil/surfor/swmag
[6] Shane, Scott, and Daisuke Wakabayashi. “’The Business of War’: Google Employees Protest Work for the Pentagon.” New York Times, 4 Apr. 2018, www.nytimes.com/2018/04/04/technology/google-letter-ceo-pentagon-project.html.
You touched a lot on why the Navy needs to switch to a CBM model and steps they could take to do so, but you didn’t touch as much on why they haven’t already switched. Is it just because of the bureaucracy? Or is it a lack of willing private sector partners (as you hinted at with the Google protests)?
Joseph, I think this is an interesting read. You touch on this a bit at the end, but my bet is that there are many other industries that would benefit from the switch to conditions-based maintenance. Do you have a good sense of how much of CDM is expected to be reporting on data gathered from sensors (e.g. “This air conditioning filter must now be replaced”) versus how much of it is using machine learning/predictive analytics (e.g. “Due to past data we are expecting that this filter will need to be replaced in 8 days”).
Joe, glad to see the Navy is making progress (albeit slow) towards this new CBM model. I completely agree with your concerns regarding bureaucratic inertia but wonder about the costs of this model. My guess is that costs are relatively high as this sensing technology and the associated algorithm probably require significant upfront investments. It probably makes sense, though, for the Navy because of the highly expensive nature of the Navy’s equipment. The Marine Corps could also greatly benefit from this CBM model but I highly doubt it will be cost effective enough any time soon for Marine Corps adoption. Many of the maintenance problems the Corps faces are due to wear and tear of relatively simple, low cost items. The Marine Corps is betting that it can scale additive manufacturing to the point that 3d printing low costs items on the spot is easier and more cost effective than having to ship them to dispersed units across the globe.
We have the same issues in the Army. Although the Army is also trending in this direction, making changes to maintenance schedules requires an enormous muscle movement, and buy-in from industry, and several multi-star commands. It will be interesting to see which service moves fastest on this and how they are able to defeat the entrenched interests.
I love that you drew on first-hand experience to clearly identify both a problem (the inefficiencies of the Navy’s current maintenance process) and a solution (CMB over TBM.) It sounds like the biggest barrier to change is regulation and bureaucracy. Are there other examples where the Navy (or other branches/the DOD) have successfully and effectively worked with private sector vendors, whether or not it relates to AI? If so, are there any learnings or best practices that can be extracted, generalized, and applied? How does the Navy work with 3rd party vendors at large, and in what circumstances? Does the Navy utilize AI elsewhere in its operations?
Hopefully a solution-oriented approach like yours can move this development process along!
Love the personal experience element of this piece. I feel like even a lot of private sector maintenance system is based on a timed framework- at least it was in the company I worked at. I think this is partially attributed to cost (must be very expensive to install these systems you talk about), but another part is labor. I think this case is unique because you are doing navy work as well as maintenance, compared to in other places, there is special labor purely for maintenance. Workers potentially don’t want to change, especially if they have done this routine for a while. It is also much harder to train new people for conditions based maintenance compared to routine. Would love to hear more from you about security concerns with this as I’m not too familiar in this area.