Discover Weekly: How Spotify is Changing the Way We Consume Music
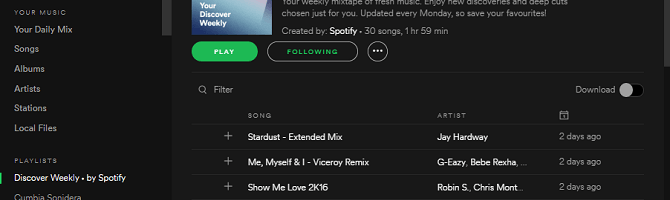
Spotify has invested heavily in machine learning to differentiate its product offering and change the way consumers experience music.
Founded in 2006, Spotify has grown to become the world’s largest music streaming platform. The service commands a ~60% market share and provides 157 million monthly active users (“MAUs”) and 71 million paying subscribers access to a music catalog of over 35 million songs [1].
The company has successfully differentiated itself from other streaming platforms by using machine learning to fuel its superior music discovery and recommendation algorithms. The ever-increasing proportion of music that is recommended to consumers has implications beyond simply improving the user experience: it is helping shift the balance of power in the music industry from traditional record labels to distribution platforms.
How song recommendations are impacting the music industry today
Given the limited content exclusivity (most platforms have licenses for the same song catalogs) and non-existent overlap in subscriber bases, user experience and technology are critical differentiating factors among streaming services. Using machine learning, Spotify has created a virtuous circle in which increased user engagement and scale drive larger data sets, data in turn drives improved recommendations and discovery and, finally, discovery drives differentiation. Today, across both its curated and machine generated playlists such as Discover Weekly and Rap Caviar, Spotify recommended over 30% of overall listening in 2017, up from less than 20% in 2015, providing a highly personalized listening experience [2].
Discover Weekly: How Spotify uses machine learning to recommend music
In order to improve its product capabilities, Spotify has made several strategic acquisitions since 2014. None were more important than the purchase of Echo Nest, a data-analytics startup that revolutionized the way music recommendations were generated by mixing the best strategies used by other services, creating a unique discovery engine that has increased Spotify’s differentiation [3].
Every week, Spotify generates a new playlist for each subscriber called “Discover Weekly”, a personalized list of 30 songs that fit that user’s taste profile. The Discover Weekly engine uses three main types of recommendation models simultaneously [4].
- Collaborative Filtering (“CF”): Models that analyze your behavior and compare it to other users’ behaviors
- Natural Language Processing (“NLP”): Models that scan the internet and analyze text about Spotify’s catalog
- Audio: Models that analyze the raw audio files.
Figure 1: Discover Weekly Data Flow [5]
Collaborative Filtering
Collaborative filtering is a key component of many recommendation engines today (Netflix, Amazon). Rather that recommended products, movies or songs based on similarities between items, collaborative filtering focuses on similarities between users. Leveraging Spotify’s database filled with everything its subscribers have historically listened to, a collaborative filtering algorithm finds similar users based upon their listening history and then suggests songs that only one user has listened to to the other.
Figure 2: Collaborative Filtering Example
Natural Language Processing
In addition to collaborative filtering, Spotify uses NLP to scrape the internet for articles, blogs and metadata about specific artists and songs. Each artist or song is then assigned a dynamic list of top terms that changes daily and is weighted by relevance. The engine then determines whether two pieces of music or artists are similar (much like in collaborative filtering).
Audio File Analysis
Spotify analyzes each individual audio file’s characteristics, including tempo, loudness, key and time signature. This not only improves the quality of recommendations for existing songs, but also enables the discovery of new songs that are less popular and do not show up via CF and NLP.
The Future of Machine Learning at Spotify
In the short term, Spotify is working on using the huge amounts of data generated to benefit the artists on their platform by providing them with very specific insight into their fans’ preferences [6]. Armed with the largest crowd-sourced dataset for music in the world, Spotify will be able to glean unique perspectives into how people consume and interact with music. As live music continues to outpace recorded music in terms of revenue generation, the company should move beyond pure music curation and use its data to help artists connect with their fans live through targeted ticket and merchandise sales.
In the medium term, Spotify is focusing on further developing its audio file analysis capabilities with the goal of further refining its sound recommendations (as opposed to song titles, text, images or artists). This will help lesser known acts increase their visibility and “level the playing field” among artists. As this predictive technology improves, I would like to see Spotify get more involved in the early stages of the music creation process (a role that is largely played by the record labels today).
Key Considerations
Spotify’s recommendation engine has significantly increased the company’s leverage ahead of the upcoming major label deal renewal negotiations slated for 2019. I would like to further explore what the company’s strategy should be ahead of these talks from a game theory perspective. In addition, I think important ethical questions exist around Spotify’s newfound ability to direct consumers to certain catalogs.
(Word Count: 795)
Sources:
- JP Morgan Research, Spotify Initiation of Coverage, April 29, 2018
- Stifel Research, Spotify Initiation of Coverage, April 5, 2018
- Robert Prey, “Nothing personal: algorithmic individuation on music streaming platforms”, Media, Culture & Society, 2018
- Company Presentation, “From Idea to Execution, Spotify’s Discover Weekly”, April 2017
- Galvanize, “Ever Wonder How Spotify Discover Weekly Works? Data Science”, August 22, 2016
- Sage Lazarro, “Spotify’s Head of Deep Learning Reveals How AI Is Changing the Music Industry”, The Observer, May 2015
Thanks for the post! I was wondering how this has changed, if at all, musicians promotion of their music? Is there a way for them to position themselves in a way to increase the amount of times they are recommended? And how does Spotify currently work with musicians? I know initially there was a lot of hesitations for artists to stream their music. Has that changed now that Spotify is in control of the landscape? How can Spotify continue to work on increasing it’s vast music catalog, which is essential in the success of its music recommendation algorithm. I think your point about level playing field is also very interesting. I think one of the challenges Spotify will face will be recommending songs that are played less frequently. I imagine that right now more popular/known songs are highlighted more often to users and, as a result, this innovation has actually fueled less music exploration.
Thanks for the post, BCA! As a proud Spotify user since 2008 and a big fan of the Discover Weekly playlist, I found your article really interesting and I think it raises a number of relevant questions. Data is allowing the Swedish company to grow its core business through new product development (e.g. discover weekly) but it also has potentially to turn Spotify into a vertically integrated music company -by engaging in content creation and ticket sales. With its current market share of 60% and the potential to dominate new music creation and the live show scene, at what point will Spotify become too big/dominant in a discipline -music- where diversity of sources matters profoundly?
Thanks for sharing BCA – it was an interesting read. Definitely seems like machine learning is a very promising field for Spotify given the vast quantity of data they have. I think it would be fascinating to learn more about how spotify clusters individuals into groups based on music tastes as they can often be quite varied across individuals. I do wonder how their peers, such as Apple Music, are competing in this space – is their software proprietary or is prediction of music tastes a phenomena which all streaming companies are doing. I imagine this will be a consideration in the question you raise regarding game theory, and predicting how other players in the market are going to act based on each of Spotfiy’s moves.