Can data buy you value in the capital-intensive oil and gas industry?
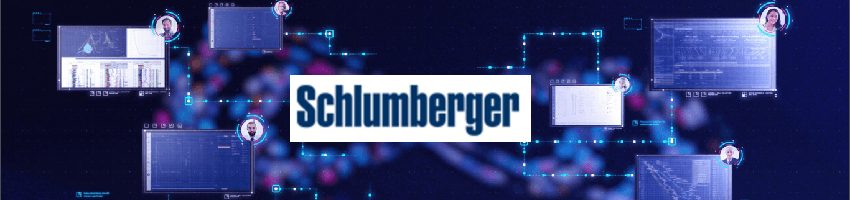
“Data is just like crude. It’s valuable, but if unrefined it cannot really be used.” [1]
The oil and gas exploration and production (E&P) industry has been known to meet market challenges with innovation and technology. With its cyclical nature, global impact and dependence on the geopolitical landscape, it must constantly keep abreast with latest innovation to continue creating value. Recent years of depressed oil prices and industry uncertainty have forced oil and gas companies into strict capex budgeting, causing mass-layoffs and impacting their reserve replacement ratios, RRR [2] (Less than 100% means that it is producing more than it is replacing, with the risk of running out of oil in the long term). This demands for better use of available information with lesser investment in acquiring new data, more collaboration between different sources and leveraging the power of machines to derive deeper insights, driving down costs while maximizing value.
Schlumberger, a 90-year old company and the world’s largest oilfield services company, has been helping its customers by designing and building software applications for the petro-technical and engineering communities across the industry. Every day, Schlumberger records several terabytes of data from operating in 80 countries across the full spectrum of E&P technology. More than 4,500 domain scientists such as geologists, geophysicists and production engineers work on these. Data is getting progressively complex, widespread and large, making it increasingly difficult to process and analyze using traditional data processing or management tools. Can emerging areas related to machine learning, artificial intelligence and cognitive engineering have the potential of adapting and automating human intelligence, to analyse and efficiently process large data sets within practical turn-around times? “To decide, you need to think about the problem to be solved and the available data, and ask questions about feasibility, intuition, and expectations.” [3].
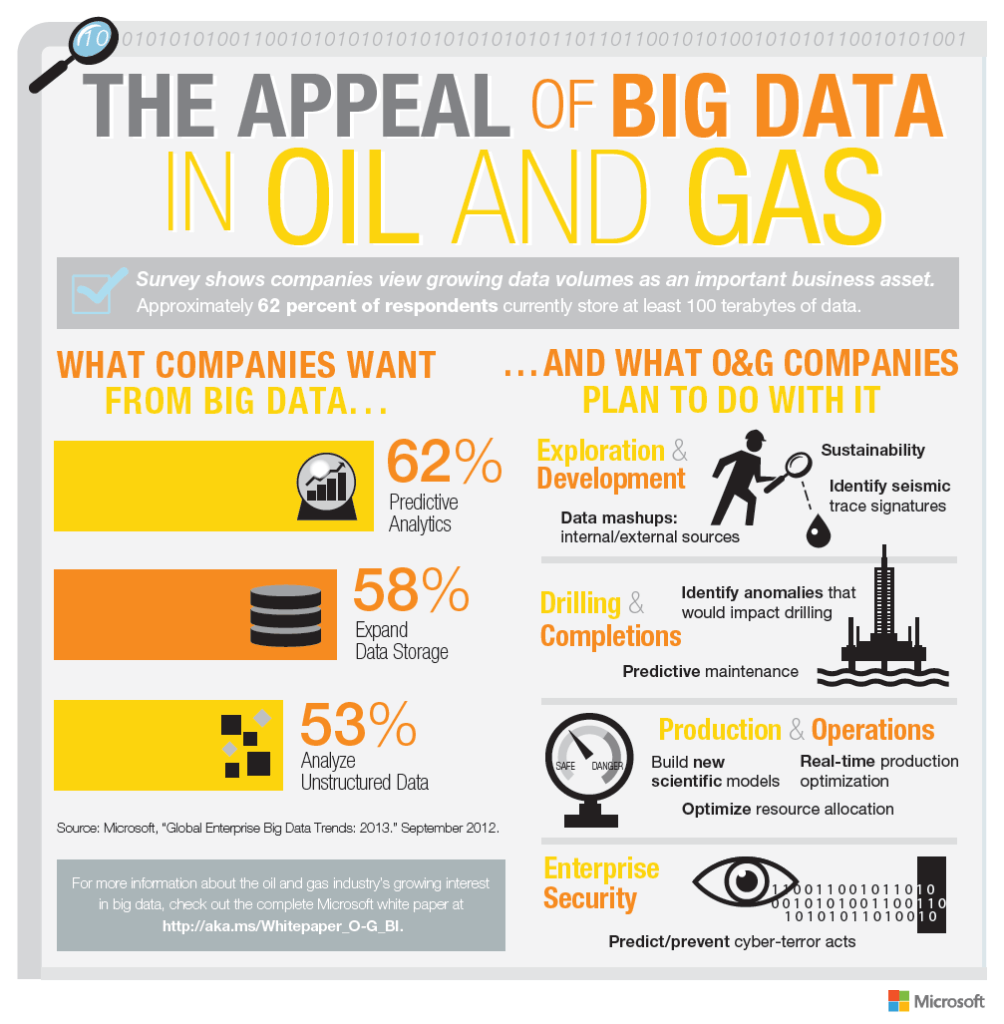
Birth of DELFI: The challenges of integrating such large volumes of data from around the world, and the knowledge of Schlumberger’s domain experts to build a system that would map commonalities in the data-sets and identify patterns that produce replicable workflows quickly, led to the birth of DELFI – the cognitive E&P environment. Launched in September 2017, the DELFI environment leverages digital technologies including security, analytics and machine learning, high performance computing (HPC) and Internet of Things (IoT) to improve operational efficiency and to deliver optimized production at the lowest cost per barrel. [4]
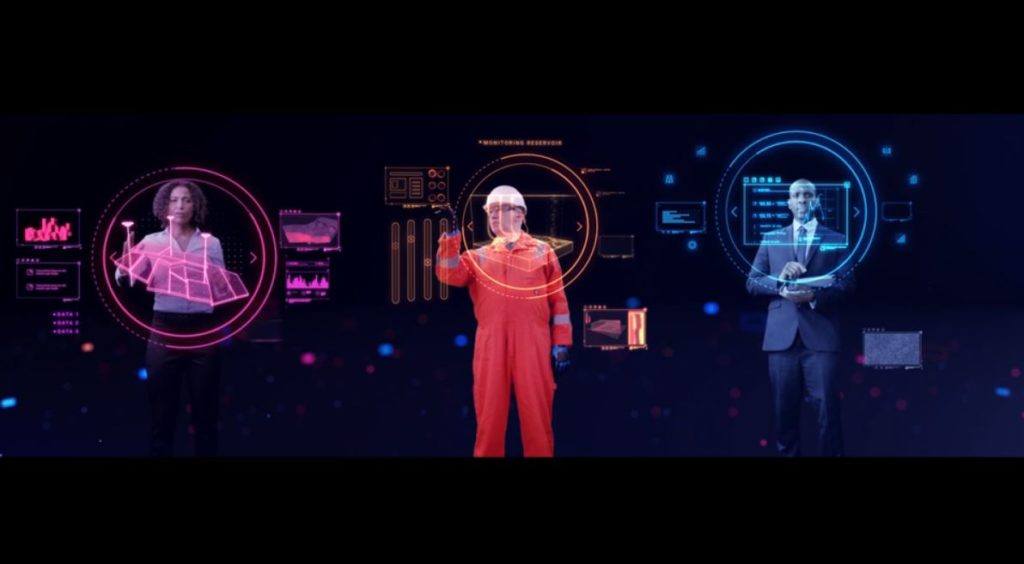
DELFI aims to strengthen the integration of the geophysics, geology, reservoir engineering, drilling and production domains, and to increase collaboration by allowing Schlumberger’s customers and software partners the ability to add their own intellectual property and workflows. “With the launch of the DELFI environment, we deployed an E&P Data Lake on the Google Cloud Platform comprising more than 1,000 3D seismic surveys, 5 million wells, 1 million well logs and 400 million production records from around the world, demonstrating a step change in scalability and performance,” Ashok Belani, executive VP of technology, said in the release.[5] Schlumberger recognizes the value of having a rich database to maximize the value of its machine learning capabilities. Machine-learning relies on adequate and accurate input. It is crucial to understand that “data deluge is important not only because it makes existing algorithms more effective but also because it encourages, supports, and accelerates the development of better algorithms.” [6]
Role of human intelligence in machine-learning: A good data analytics system enables discovery and recognition of meaningful patterns in the data using a combination of mathematics and statistics, descriptive techniques and machine learning, driving decisions and actions. However, one should not ignore the value of human intelligence in building such a robust machine system. Schlumberger engaged both its domain experts and user base to understand the areas with most potential, to identify what data was needed and in what form, for the machine to best utilize it. Several ‘proof of concept’ tests were run to identify potential technical, logistical or practical issues early on. “The best results we got were when we were able to connect the people who understood the technology with people who understood the science and domain and then focused them on an outcome,” [7] said Rennick, president, software integrated solutions, at Schlumberger.
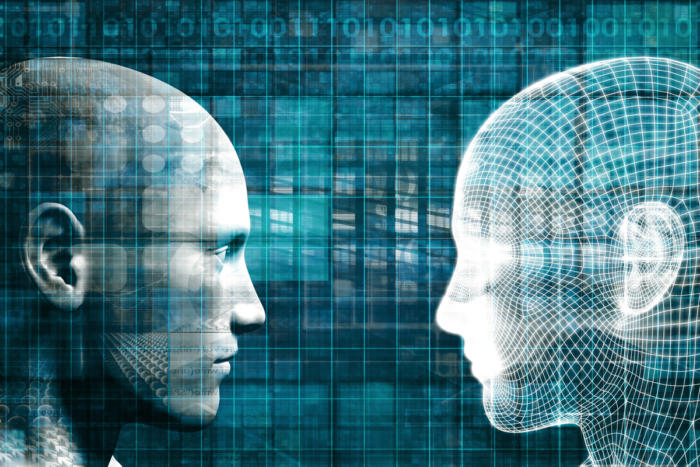
Conclusion: “In steps collaboration, integration and optimization- the unofficial buzzwords of an industry clawing its way back from a profit-gobbling downturn – and another digital solution is born.” [8] DELFI is in its early days but shows tremendous potential in terms of bringing together an industry which often works in silos across its many segments and geographies, grappling with ever-increasing volumes of data. DELFI will help reduce cost, turnaround times and increase efficiency and accuracy. Creating large and secure cloud-based data lakes and allowing machine-learning and artificial intelligence to sift through these large volumes to identify patterns and come up with solutions will vastly reduce labor cost, allowing valuable human labor to shift from mundane and repetitive tasks to more intellectually stimulating value-added problems.
(799 words)
[1] Michael Palmer, “Ana Marketing Maestros”, Data is the New Oil (blog), November 03, 2006, https://ana.blogs.com/maestros/2006/11/data_is_the_new.html
[2] Robert Perkins, “Analysis: Oil majors see reserves slip for fourth straight year”, S&P Global Platts, April 18, 2018, https://www.spglobal.com/platts/en/market-insights/latest-news/oil/041818-analysis-oil-majors-see-reserves-slip-for-fourth-straight-year
[3] Anastassia Fedyk, “How to tell if machine learning can solve your business problem,” Harvard Business Review Digital Articles, November 25, 2016, https://hbr.org/2016/11/how-to-tell-if-machine-learning-can-solve-your-business-problem
[4] “Schlumberger Announces DELFI Cognitive E&P Environment”, press release, September 13, 2017, on Schlumberger website, https://www.slb.com/news/press_releases/2017/2017_0913_delfi_pr.aspx
[5] “Schlumberger Introduces DELFI Cognitive E&P Environment”, DrillPlan, September 13, 2017, Hart Energy,https://www.epmag.com/schlumberger-introduces-delfi-cognitive-ep-environment-drillplan-1657351#p=full
[6] Erik Brynjolfsson and Andrew McAfe, “What’s driving the machine learning explosion?, Harvard Business Review Digital Articles, July 01, 2017, https://hbr.org/2017/07/whats-driving-the-machine-learning-explosion
[7] Dave Perkon, “Schlumberger Finds Efficiency in Data”, on Schlumberger website, November 01, 2017, https://www.slb.com/resources/publications/industry_articles/software/201711-control-schlumberger-finds-efficiency-in-data.aspx
[8] Velda Addison, “Schlumberger talks Harvesting Collaboration with Digital Technology”, Unconventional Oil and Gas Center, October 2017, https://www.slb.com/~/media/Files/software/industry_articles/201710-ep-harvesting-collab-digital-tech.pdf
With the amount of data Schlumberger takes in every day, the man-hours alone seem daunting. AI augmentation appears to be an ideal way to create useful streams of information from the data lakes. These algorithms will become more accurate and useful as humans analyze the predictions and trends.
The comment that stood out to me in your post was “machine-learning relies on adequate and accurate input”. I agree with this statement and it has made me skeptical about some of the practices that Schlumberger is emplying for its data lake. Collaboration across Schlumberger customers and partners is a great way to get a large data set, but I wonder if data coming from mutliple sources might inherently have some bias. It is possible that some customers might have over representation in the data set which may add bias towards a specific practice or geography. Collaboration across customers can also be difficult because there are sometimes additional context or factors that may be confidential and are not included in the shared data causing an impact on the validity of the model.