Can an Artificial Intelligence Deliver Real Results?
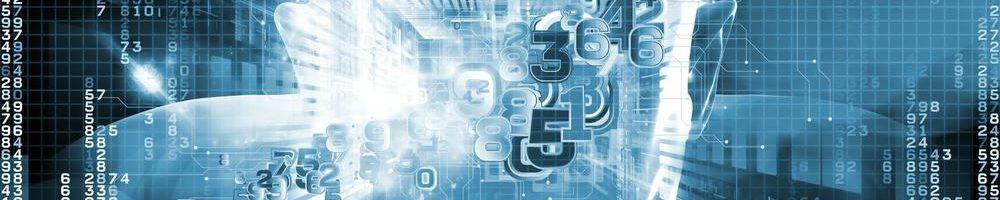
The digitalization of healthcare has added greater complexity and costs, and Memorial Sloan-Kettering Cancer Center believes artificial intelligence may be the solution.
In 2012, Memorial Sloan-Kettering Cancer Center (MSKCC), the world’s oldest and largest private institution devoted to prevention, patient care, research, and education in cancer, announced the beginning of a collaboration with IBM to harness the potential of artificial intelligence (AI) to improve patient outcomes and reduce operational costs in clinics [1]. This ongoing collaboration is in response to the massive digitalization of healthcare information and the increasing complexity of our knowledge of biology and disease treatment. Between 2010 and 2015, patient data increased by 700%, of which 91% was unstructured and thus unorganized in the form of electronic physician notes and lab reports [2]. By 2020, this volume of data is projected to grow to 2,314 Exabytes, adding more costs to the health system and creating bottlenecks for data scientists, clinicians and researchers tasked with interpreting this tsunami of data in real-time to draw meaningful insights [3]. By combining MSKCCs clinical knowledge, patient data, molecular and genomic data, and publicly available medical literature with the computational abilities of IBM Watson, this partnership aims to overcome the challenges of digitalization.
Cancer is one of the leading causes of death in the US with approximately 1.6M new diagnoses in 2016 and projected total costs of nearly $156B by 2020 [4]. Our understanding of the role of circulating biomarkers and the unique genetic signatures of tumors in overall tumor biology has led to new insights that promise improved outcomes [5]. However, these advances take years to reach the clinic. It is the general belief among scientists and the medical community that AI can shorten this time delay, leading to standardized cancer treatments in the US and around the globe.
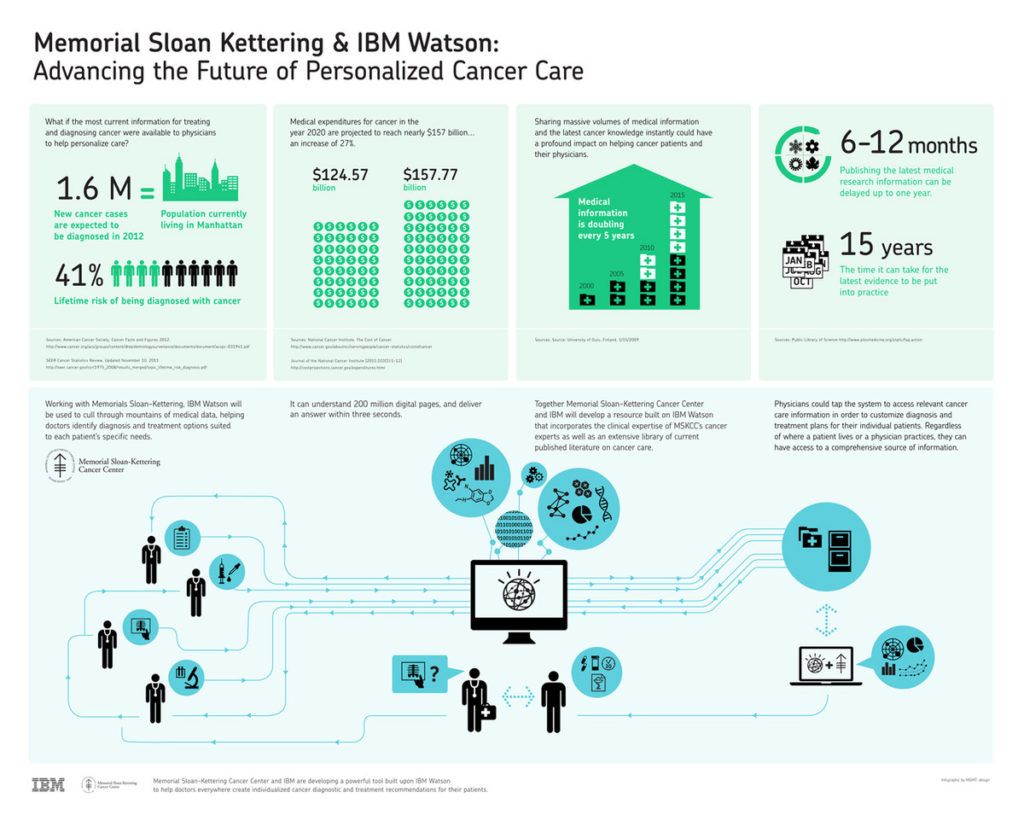
In the short-term, MSKCC is using its doctors and data from the 30,000 cancer patients seen each year to build the machine’s knowledge base [6]. Management intends to use this information base to expedite patient selection for clinical trials, identify research opportunities from their vast trove of data, reduce variability in care, increase compliance with treatment guidelines, and speed the dissemination of best practices. At the 2017 American Society of Clinical Oncology scientific meeting, researchers announced that Watson had reduced the time required to screen patients for clinical trial eligibility by 78%. Moreover, it had achieved concordance rates ranging from 81% to 96% with multidisciplinary tumor boards treating lung, colon, and rectal cancer, suggesting that IBM and MSKCC’s early efforts are starting to pay dividends [7].
In the long-term, MSKCC hopes to create an evidence-based decision support tool that offers clinicians multiple treatment options with various degrees of confidence supported by the medical literature, guidelines, and prior patient outcomes, thus reducing the throughput time between initial patient encounters and treatment. This, in turn, is expected to improve clinic capacity and output, as well as physician utilization. By standardizing and automating routine tasks, enhancing physician capabilities, and allowing clinicians to allocate time more effectively to complex tasks, Accenture believes that AI can deliver $150B in annual savings to our healthcare system by 2026 [8]. To support these long-term efforts, the partnership with IBM has grown to include Quest Diagnostics, the Mayo Clinic, and the Broad Institute – a Harvard and MIT affiliated research institute. MSKCC has also expanded its platform to a hospital system in India and the Jupiter Medical Center in Florida [9].
Research from PricewaterhouseCoopers suggests that AI will increase global GDP to 14% by 2030, adding $6.6T to the global economy through increased productivity [10]. At a CAGR of 40%, the AI market in healthcare is expected to grow from $600M in 2014 to $6.6B in 2021 [8]. However, AI has met multiple challenges, as seen by the failed partnership between MD Anderson and IBM [11]. MD Anderson terminated the partnership due to cost overruns totaling $62M and a failure by management to ensure quality throughout the development process. This failure demonstrates the importance of cautious expectations and investing in product development processes, like the stage-gate method, to ensure quality. Moving forward, hospitals must perform randomized trials to demonstrate that the technology improves outcomes and reduces operational costs. In parallel, management must address patient privacy concerns and invest in systems to protect patient data. Looking into the future, when such machine learning platforms become commoditized, the primary asset will likely be the data provided to the machine’s knowledge base. Given that patients and hospitals are the providers of this data, questions such as who in the end owns the technology will need to be addressed. Additionally, how much (or not) should patients pay for a service that would not have existed without their generous, and possibly unknowing, contribution of personal information? These and other questions will certainly be an ongoing subject of debate in the years ahead.
(word count: 784)
References:
- News Releases. IBM, 22 Mar. 2012, www-03.ibm.com/press/us/en/pressrelease/37235.wss. Accessed 11 Nov. 2017.
- Williams, Brian. “Enabling better healthcare with artificial intelligence.” Next In Tech, PWC, 28 Aug. 2017, usblogs.pwc.com/emerging-technology/ai-in-healthcare/. Accessed 11 Nov. 2017.
- “Health Data Volumes Skyrocket, Legacy Data Archives On The Rise.” Health Data Archiver, 3 May 2017, www.healthdataarchiver.com/health-data-volumes-skyrocket-legacy-data-archives-rise-hie/. Accessed 11 Nov. 2017.
- “Cancer Statistics.” National Cancer Institute, 22 Mar. 2017, www.cancer.gov/about-cancer/understanding/statistics. Accessed 11 Nov. 2017.
- Vaios, Eugene J., et al. “Bone marrow response as a potential biomarker of outcomes in glioblastoma patients.” Journal of neurosurgery(2016): 1-7.
- “Memorial Sloan Kettering Trains IBM Watson to Help Doctors Make Better Cancer Treatment Choices.” Memorial Sloan Kettering Cancer Center, 11 Apr. 2014, www.mskcc.org/blog/msk-trains-ibm-watson-help-doctors-make-better-treatment-choices. Accessed 11 Nov. 2017.
- “At ASCO 2017 Clinicians Present New Evidence about Watson Cognitive Technology and Cancer Care.” News Releases, IBM, 1 June 2017, www-03.ibm.com/press/us/en/pressrelease/52502.wss. Accessed 11 Nov. 2017.
- Collier, Matthew, et al. “Artificial Intelligence: Healthcare’s New Nervous System.” Accenture Consulting, 2016, www.accenture.com/us-en/insight-artificial-intelligence-healthcare. Accessed 11 Nov. 2017.
- Freedman, David H. “A Reality Check for IBM’s AI Ambitions.” MIT Technology Review, 27 June 2017, www.technologyreview.com/s/607965/a-reality-check-for-ibms-ai-ambitions/. Accessed 11 Nov. 2017.
- Rao, Anand S., Dr., and Gerard Verweij. “Sizing the Prize.” Publications, PWC, 2016, www.pwc.com/gx/en/issues/data-and-analytics/publications/artificial-intelligence-study.html. Accessed 11 Nov. 2017.
- Jaklevic, Mary Chris Jaklevic Chris. “MD Anderson Cancer Center’s IBM Watson project fails, and so did the journalism related to it.” Health News Review, Apr. 2016, www.healthnewsreview.org/2017/02/md-anderson-cancer-centers-ibm-watson-project-fails-journalism-related/. Accessed 11 Nov. 2017.
Great essay Eugene, really captures a challenge which is both ethical and economical. You are raising two important questions: who owns the AI technology? How much should patients pay for a technology that would not exist without the data they provided? I believe that the power of this technology combined with its intended use for health care purposes will eventually make a version of it operated by governments to ensure both economic accessibility and data protection for the patients. This directly answers your second question: the natural path to AI in healthcare would be a virtuous circle of patients giving their data to receive effective treatments in return.
Eugene, thanks for the great read! The amount of potential impact AI can make on the healthcare system is really exciting, but the concerns you raise are major ones that need to be addressed. I agree with Sasha in that ideally, patients should own their data and offer it knowingly to train an AI platform like Watson in exchange for the expected benefits. Realistically, though, at least in the medium-term, hospitals will continue to have ownership over patient data and (I believe, though I’m no legal expert) can share it as they see fit excluding any personally identifiable information — I don’t see why that couldn’t continue. Then a key lever will be Watson or its competitors building partnerships focused on scale, to get access to the maximum amount of data and build a competitive advantage that way.
I suspect that another huge lever to win in this space will be ease of use for physicians. I am very curious as to how Watson will fit in to physician workflows and how much resistance to expect. The physician community, at least in part, is already significantly frustrated about electronic health records and other technologies being too complex, not user-friendly, and drawing too much physician time away from actual patient care. Do you think Watson will be different?
This is a very interesting topic. One question I had in reading is whether this AI technology is yet cost effective, and if not when (and via what levers) we can expect it to be so? One area I’m more familiar with in this space is predictive radiology, which companies like Zebra [1] and other imaging technologies are starting to take to market. These companies claim that by using their technology, physicians can get to much quicker (and cheaper) diagnostics. However, my understanding of these technologies is they are only being utilized by large, research hospitals because the ROI is not there yet for mass market adoption. In other words, the implementation and utilization costs do not yet make up for any gained productivity, and as a result only hospitals with deep pockets that are excited about the innovation potential and are willing to sacrifice on the bottom line to utilize these products. Additionally, I think there are questions over who will be able to create a large enough data set to have an effective AI machine over time? Do you expect we will see more collaboration between hospitals to get this data set, as no one hospital will produce enough data to make the algorithms and predictive technology work? What are some of the barriers to hospital collaboration?
[1] https://www.zebra-med.com/