BlackRock: Is the Future of Investing in Machine Learning?
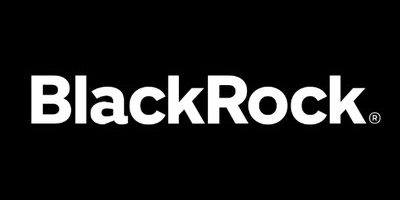
This post discusses how BlackRock is leveraging AI more broadly and ML specifically for process improvement and product development, which could result in potentially significant revenue generation.
The recent revolution in Big Data due to exponential increases in the amount of data, computing power, and data storage at lower costs, coupled with advances in machine learning (ML) that can analyze this data, has led to a resurgence in Artificial Intelligence (AI) [1]. One of AI’s biggest use cases, specifically in terms of revenue generation, is investment management (see Figure A).
Figure A [2]
How can the adoption of AI more broadly and ML specifically lead to such significant revenue? First, it can lead to new product development as Big Data broadens investment managers’ viewpoints and ML techniques help portfolio managers comb through and apply said data. Second, it can help process improvement as humans become smarter when coupled with computers, and ML itself improves efficiencies and reduces errors, freeing up humans to focus on investor needs [3].
Given these potential use cases of AI and ML, what are firms in the space currently doing? According to a 2017 Barclays report, the most popular use case of ML is cleaning traditional data sets. While 88% of managers who use ML use it to process data, only 25% use it as part of their investment decisions. Reasons for this include concerns that the price of Big Data is greater than its usefulness, assessing the data itself is challenging, and while ML is good at finding correlations, it is difficult to determine causation [4]. While BlackRock has historically been a forerunner in adopting AI, potential competition exists from traditional firms and fintech startups [2].
BlackRock has taken many steps to address the AI revolution in the near term. First, the BlackRock Lab for Artificial Intelligence was established in winter 2018 to “augment our current teams and accelerate our efforts to bring the benefits of these technologies to the entirety of the firm and to our clients,” said COO Rob Goldstein [5]. Second, the firm announced the creation of Data Science Core in its 2017 letter to shareholders. The core is meant to formalize data science efforts already underway and leverage AI in solving high priority issues [6]. Third, BlackRock aims to use AI to automate back-office functions which will improve processes through speed, fewer errors, and lower costs [5].
BlackRock has also taken steps to incorporate ML into its investment process. First, it is collecting new types of data for potential use in decision making. Examples include analyzing corporate website traffic as an indicator of a company’s future growth prospects, text analysis on earnings calls transcripts to glean management team sentiment, and geolocation data to assess retailer popularity [5]. Some of this data may prove to have no value as complex techniques do not necessarily result in better forecasting [1]. BlackRock should learn over time what constitutes good data and how to incorporate it in meaningful ways. Additionally, BlackRock released seven AI-powered sector ETFs in March 2018. ML techniques are being used to parse language in public filings to determine how to weight each company within the sector ETF [7]. Again, it will take time to assess the validity of this approach.
BlackRock also aims to create a data-driven feedback loop in its sales and product development process [6]. This will allow BlackRock to improve efficiency as it responds in real-time to client needs and will lead to the creation of desired products. Lastly, BlackRock also plans to use AI to deliver investment capabilities to the masses in due time, providing investors access to analytics previously only available to larger, institutional clients [8].
In the short term, hiring data scientists to employ ML and AI will likely be an issue as data science is a relatively nascent field and BlackRock is one of many companies vying for this talent. BlackRock will also need to continually assess the usefulness of new data sources. BlackRock must also stay abreast of any developments in the AI space, which is difficult to do given rapid changes.
In the medium term, BlackRock could acquire companies producing applicable technology or fintech competitors. BlackRock should contribute to academic research in the ML/AI space to solidify its reputation as a thought leader and lend credence to its AI efforts. Any investment process using AI will need to be validated and understood by portfolio managers and explained well to clients. The path to the successful application of AI, in process improvement and particularly in product development, will not be linear. Portfolio managers must accept potential setbacks and inform investors in their fiduciary duties of highlighting key risks.
The largest open question related to ML/AI in investment management is whether AI can ultimately replace human managers. Would humans tolerate an AI investor who outperforms the market but whose methods are indecipherable or unintuitive? Does human plus machine truly beat machine?
(Word Count: 790)
References:
[1] JP Morgan, “Informing Investment Decisions Using Machine Learning and Artificial Intelligence,” https://www.jpmorgan.com/global/cib/research/investment-decisions-using-machine-learning-ai, accessed November 2018.
[2] Lea Nonninger, “BlackRock Further Strengthens its AI Efforts,” Business Insider, February 21, 2018, https://www.businessinsider.com/blackrock-further-strengthens-its-ai-efforts-2018-2, accessed November 2018.
[3] Jody Kochansky, “The Promise of Artificial Intelligence and What it Means to BlackRock,” BlackRock Blog, March 8, 2018, https://www.blackrockblog.com/2018/03/08/artificial-intelligence-blackrock/, accessed November 2018.
[4] Sviatoslav Rosov, “Behind the Hype: Barclays Report on Machine Learning in Investment Management,” CFA Institute Blog, July 24, 2017, https://blogs.cfainstitute.org/marketintegrity/2017/07/24/behind-the-hype-barclays-report-on-machine-learning-in-investment-management/, accessed November 2018.
[5] Robin Wigglesworth & Chris Flood, “BlackRock Bulks Up Research into Artificial Intelligence,” Financial Times, February 19, 2018, https://www.ft.com/content/4f5720ce-1552-11e8-9376-4a6390addb44, accessed November 2018.
[6] BlackRock, Inc., December 31, 2017 Form DEF 14A, http://ir.blackrock.com/Cache/392942428.pdf, accessed November 2018.
[7] Crystal Kim, “BlackRock Unveils 7 AI-Powered Sector ETFs,” Barron’s, March 23, 2018, https://www.barrons.com/articles/blackrock-unveils-7-a-i-powered-sector-etfs-1521826251, accessed November 2018.
[8] Jody Kochansky, “Artificial Intelligence: An Evolution or a Revolution?” BlackRock Blog, August 3, 2018, https://www.blackrockblog.com/2017/08/03/artificial-intelligence-evolution/, accessed November 2018.
Hi CG,
I find this to be a really exciting topic. Great job covering all the different angles for AI to benefit Blackrock (investing, process improvement, etc.). The use cases you shared such as text analysis on earnings calls transcripts are interesting. I would have been curious to know, in your opinion, which use case is most promising, and why would Blackrock be best positioned to win? It seems to me like Blackrock has little proprietary data, so barriers to entry for applying ML to publically accessible data are low (e.g. using Tensorflow).
Hi CG. Amazing topic choice, really interesting perspective on how Blackrock is taking the lead in the AI space within Finance. Some thoughts on this: firstly, I think most of the advancements in Machine Learning in this space until today have been related to structured learning, meaning that data scientists are more or less guiding the systems in terms of goals and available datasets. My intuition is that the big leap forward in investing will come from unstructured learning. Once an algorithm can successfully scan the whole web in search of market predictors I think we will start seeing real advances: for example measuring people’s mood in real time or establishing ultra complex relationships in real time between an article the newspaper in Nepal and the share price of GAP.
To your question on would humans tolerate an AI investor who outperforms the market but whose methods are indecipherable or unintuitive? I would 100%. I currently fly on an airplane 30000 feet from the ground completely managed by a computer while the pilots are taking naps… I think that’s way more risky than having a computer manage my 401k!