AI on the Frontline: The Future of Warfare
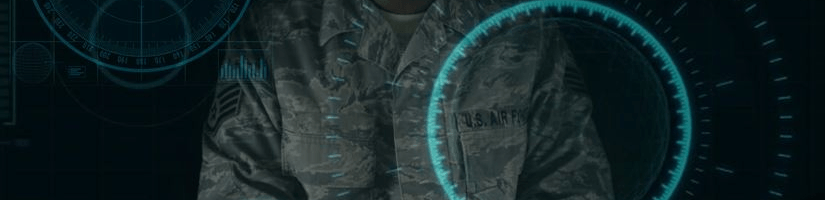
What does the future of warfare look like? What boundaries need to be implemented as AI is introduced to combat environments? Explore how machine learning is helping the US military adapt to the modern battlefield.
AI on the Frontline: The Future of Warfare
Since its inception, the US military has been engaged in an arms race driven by advancing technology – the catalyst for the ever-evolving frontierof war. As the rate of military automation adoption accelerates, increased scrutiny of data and its collection will force US soldiers and policy makers alike, to embrace the trend of machine learning. With the frontline being transformed by small decentralized enemy threats, status quo conventional warfare models are quickly creating knowledge gaps that demand innovation. Notwithstanding, the era of big data will force the US military to question how to adopt and implement machine learning using drones and predictive intelligence analysis to maintain its technological superiority.
Machine Learning in Combat
In an underground bunker in Kabul, Afghanistan, live drone footage is projected on wall-to-wall screens. Near the front of the room, drone strike authorities are deferred to a Two-Star General who approves the launch based on intelligence reports from multiple sources. The process to identify combatants proves to be accurate, yet time consuming. In an effort to explore how to utilize machine learning to decrease strike throughput time for the short term, the Department of Defense (DoD) has launched an initiative titled “Project Maven”. Project Maven uses deep learning from hours of previous drone footage and algorithms to identify cars, buildings, and other objects that simplify tracking enemy threats.[1] With the end state to decrease collateral damage, the intent is not to eliminate human analysts from the decision process, but rather reduce the variability of the time taken to make those decisions.
As drone usage has increased within combat environments, machine learning has also been implemented to compliment decades-old intelligence practices on the dynamic battlefield. In June of 2017, the Pentagon led an initiative that focused on using algorithms and the analysis of big data to strengthen military simulation practices to better predict global threats. Known as the Task Force on Gaming, Exercising, Modeling, and Simulation (GEMS), this new enterprise looks to use an empirical approach to help soldiers focus on relevant mission tasks rather than other variables like rank or historical and cultural biases when making decisions. From analyzing data like insurgent movement patterns to learning to negotiate with a villager elder of a certain province, machine learning can lead to a better understanding of how to interpret the different cultural factions and language idiosyncrasies found in diverse anthropologic regions. [2] The implication of the advancement of GEMS, lies in its potential to change the foundational practices of the intelligence community for decades to come.
Trouble on the Horizon
Although the demand for machine learning is ubiquitous throughout the defense community, the ethical and political opinions of the contributions from private corporations remain divided. In June 2018, Google elected to pull out of its DoD contract with Project Maven following public and internal backlash due to the initiative’s military purpose. [3] Continuity errors between contracted companies and opinions on the ethical use of technology, need to be addressed in the short term to build a coherent front towards further innovation. In the case of Google, even future lucrative defense contracts could not assuage conflicted employees to continue its support. As the DoD moves forward, it needs to address its retention of contracted entities – higher cash premiums may no longer be enough when battling the popular opinions of activist watch dogs.
With intelligence predicting algorithms advancing over the next decade, the fine balance between human reasoning and machine-driven decisions will also weigh heavily on troops in combat environments. As adversarial example (models intentionally designed to force AI mistakes) experiments have shown, machine learning systems can be prone to false alarms, forcing analysts to make decisions on what should be considered actionable intelligence.[4] The advancement of machines explaining their reasoning for these high-stake decisions will be crucial in implementing human authorities. “Explainability” will serve as the next objective in convincing the wide spread adoption of machine learning over the medium term.[5]
The Evolution of War
The advancement of machine learning will continue to shape warfare, but several questions over its deployment remain. As incidents involving collateral damage may occur, how will DoD policy and US legislation react to define the authorities of armed AI? Similarly, what is an acceptable confidence interval to physically endanger the lives of troops based on a strategy chosen by machine learning?
Warfare has historically served as a catalyst for innovation and has produced technology spanning from microwaves to GPS devices. The advancement of machine learning for defense measures is inevitable – the military’s ability to nurture the R&D process however, is critical in determining that rate of evolution.
(Word Count: 772)
[1] Pellerin, Cheryl, “Project Maven to Deploy Computer Algorithms to War Zone by Year’s End,” U.S. Department of Defense, June 21, 2017, https://dod.defense.gov/News/Article/Article/1254719/project-maven-to-deploy-computer-algorithms-to-war-zone-by-years-end/, accessed November 2018.
[2] Source: InsideDefense.com’s SitRep, “DOD panel exploring how machine-learning algorithms might assist military decision-making,” InsideDefense.com’s SitRep, 2017, ABI/INFORM via ProQuest, accessed November 2018.
[3] Daisuke Wakabayashi and Scott Shane, “Google Will Not Renew Pentagon Contract That Upset Employees”, The New York Times, June 1, 2018, https://www.nytimes.com/2018/06/01/technology/google-pentagon-project-maven.html, accessed November 2018.
[4] Goodfellow, Papernot, Huang, “Attacking Machine Learning with Adversarial Examples,” Open AI, February 24, 2017, https://blog.openai.com/adversarial-example-research/, accessed November 2018.
[5] Source: Knight, Will, “The Dark Secret at the Heart of AI,” Technology Review, 54-63, 2017, ABI/INFORM via ProQuest, accessed November 2018.
This is very interesting. A bit unsettling, but it leads me to believe we truly need to understand AI better before making big decisions.
I have little (no…) background in the military, but I would wonder whether there is enough data (more importantly, enough similar data) for machine learning to properly predict and analyze specific situations. I would be concerned that each situation is so unique that you might risk projecting what happened in the past on what you think might happen in the future, even if there may be a better alternative. I think the more information and data points, the better the predictions will be and hope that continued improvements in technology can help save lives and avoid more challenging conflicts, but would wonder how long it takes to get there and how accurate the information can ever be independently. I agree with your point that the technology should remain as a support to human intelligence rather than replacing it, and am not sure at what point that could potentially change (if ever).
I think it will be a very long time before computer algorithms can make dynamic decisions on the battlefield. The information is “fuzzy” and often ambiguous, requiring the best human intuition and judgment to avoid catastrophic mistakes. However, it could certainly be useful in lesser capacities! We often took radar-generated images of the ground and were required to manually pick out the bad guys from blobs of green pixels, which was sometimes extremely difficult to do. A trained algorithm would be excellent at this type of tasking, allowing the human to perform the task they are better suited for–qualitative judgment.
I believe that the required confidence interval for a machine to make decisions on the battlefield needs to be in line with what we know about the accuracy / success rate for humans most of the time. I think there is a bias that humans actually make logically superior decisions, when in fact they often face emotional biases which in some times are helpful but can also be harmful. My main concerns with this is the ability for a malicious party to hack the algorithm and intentionally cause mistakes, and the availability of sufficient data for an algorithm to make unbiased decisions. I imagine that many decisions and tasks can be unique, in at least certain aspects, on the front line and therefore the algorithm would be limited by this.
Great topic! Your question rings familiar to the question raised during the IBM Watson case – do we, as humans, give machines a narrower confidence interval (or perhaps, a span of 0) than we give ourselves? Do we allow for greater leeway in the capacity for human error than we leave machines (in this case, drones) powered by machines? This instance of warfare, especially in the American context, is a tricky one, that will likely play out in the political landscape in the near future. The extent to which unexpected casualties can be minimized and technological advancements can be maximized will only occur in the private sphere. The American government, frankly speaking, does not have the talent or built-in infrastructure to nurture this kind of technological development. Private enterprises, and the innovation incubated within those private enterprises, are the only path forward for the next generation of AI powered warfare.
This is fascinating. However, when human lives are potentially at hand, I think the threshold for trusting machine learning algorithm guidance should be set higher. As you mentioned, there are adversarial examples where machine learning algorithms may be tricked into giving answers contrary to what they might usually provide. Because machine learning logic is still a “black box,” it might be difficult to discern the rationale behind strategy recommendations. The ethics behind AI-driven decisions are also interesting to consider – who will be taking the blame when AI makes an ill-guided suggestion?
I remember Obama saying during the 2012 election in the Romney-Obama debate, after Romney has criticised him for the Navy having fewer ships in 2012 than they had in 1916: “Well, Governor, we also have fewer horses and bayonets, because the nature of our military’s changed.” This articles showed me that even though machine learning bares high risks and still has a lot of errors, it will enhance and replace technology in warfare in the long-term.
Thank you for the essay on this fascinating topic. The most unsettling part of it, in my opinion, is understanding whether there is such a confidence interval to endanger people’s lives at all.
As we discussed in class, humans seem to be less tolerant with machine-led mistakes than to human error so I wonder whether AI could ever supersede human-made decision-making in warfare due to public opinion & human rights considerations.