The Dark Side of People Analytics
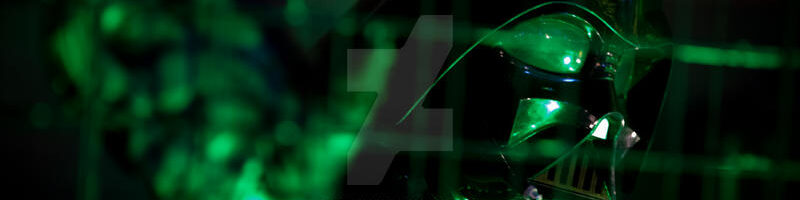
People Analytics is transforming the modern-day HR department by spearheading a new era of data-driven decision-making. Yet, if technology is to replace human judgement, talking about the dark side of people analytics is necessary if not critical.
Modern-day organizations increasingly rely on people analytics to inform the way they identify, develop, manage, and control their workforce. Advances in the field artificial intelligence – such as natural language processing – are enabling companies to link data on human behavior, social relationships, and employee characteristics to internal or external business information. Such advances are praised and adopted widely by organizations seeking to replace human judgement and streamline processes that have traditionally relied on human capital. Yet sometimes people analytics can have unintended and harmful consequences for organizations and employees. It is the dark side of people analytics that I would like to focus on in this article. What should one be aware and cautious of when deploying people analytics to advance any HR function?
1. People analytics can lead to estimated predictions and self-fulfilling prophecies
A major assumption that people analytics rests upon is that human behavior can be explained, predicted, and modified based on past events. Yet the algorithms and models people analytics deploys are merely conditional probabilities for occurrence of an even, not the event itself. This means that people analytics assesses on the probability of an employee showing a certain behavior rather than the actual behaviors.
Furthermore, applying people analytics may also lead to self-fulfilling prophesies. Imagine an organization using people analytics to forecast performance, the expected retentions, and the return on investment of new hires. Based on these predictions, the organization might allocate more resources to employees who are perceived as promising, leading to these employees outperforming their peers.
2. People analytics can foster a deference to precedent and hinder innovation
Predictive models of people analytics rely on historical data to predict future outcomes. This approach is meant to help companies solve critical problems, identify and predict future trends, and facilitate strategic workforce planning among others. However, this approach embodies a profound deference to the precedent”. Thus, people analytics helps organizations extrapolate the past to the future, which might be a dangerous practice and should be used judiciously as it might lead to biases and discrimination.
For example, Amazon set out to automate its talent search by relying on a prescriptive analytics tool to identify top candidates based on profiles of successful hires over the past decade. The data showed that the overwhelming majority of successful hires had previously been (white) men. As a result, the analytics tool predicted white male candidates are more likely to be a good fit than women. As this example underscores, hiring decisions based on historical data could lead to biases and discriminatory practices.
What do you think are some other perils and dark sides of people analytics? How can we, as future users of big data and AI enabled decision-making tools, make better use of these technologies while avoiding the dark side?
Elena, really liked your perspective on the “dark side” of people analytics. What was for me behind all of this is perhaps even people analytics (and the rigour it can bring to the workplace) as the enemy of creativity?
Hi Elena, your article is delightful very informative, and introduces the side effects of data analytics. Sometimes we stop to think about the dark side and look at the bright side. I enjoyed and remembered many essential things that we should have in our minds. The closing questions are wonderful and provide plenty of food for thought.
Hi Elena! I really love the examples you share about how an overreliance on analytics can lead to biased decision making. I totally agree with you. As you highlight, the problem seems to be a lack of clarity around what the tools are actually doing, and an assumption that they can overcome certain human biases (which as you also point out, is very much not the case!). I wonder if there are ways to grapple with these limitations? Do you think additional trainings on the tools is helpful? Or maybe it’s about thinking of people analytics as one of our many tools that should be used only when appropriate (like how a hammer and screwdriver have unique functions)?
Hi Elena,
Great title and post! I appreciate you highlighting how, in the absence of a cautious, substance-sensitive approach, relying upon data of past behavior that indicates probabilities—but not certainties—about future behavior, and may be encoded with human bias, might serve to promote confirmation bias and stymie an organization’s ability to envision and pursue novel opportunities for success.
To answer your final question, it seems as though we, as future users of Big Data and AI, can make a better use of these technologies by doing so in a critical, reflective manner that is characterized by an understanding that data interpretation is an inherently subjective process to an extent, so a thoughtful interrogation of the goal(s) and inputs of any given model is necessary. Merely receiving data-produced outcomes and regarding them as wholly objective outputs free of noise or bias runs the risk of perpetuating the perils that you note.