When the FBI gets help from inspector AI
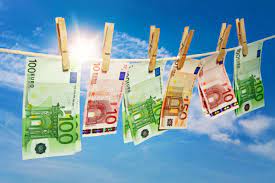
How banks leverage AI to make our planet a safer place.
Retail banking is a competitive environment to do business in. Customers do expect an Amazon-like user experience at a low cost while the low-interest rates do put the traditional revenue model of banks under pressure. On top of that, regulators expect banks to play their role in the fight against financial crime. Checking ultimate beneficial owners of a legal entity and monitoring suspicious transactions costs banks up to 40 basis points of their total revenue1, further putting pressure on the profit of these institutions.
Before joining the Sloan Fellows MBA program at the MIT Sloan School of Management, I was leading the software development unit for “Onboarding & Know Your Customer” of ING, a large European retail bank. Back in 2018, ING agreed on a settlement of $900 mio with the Dutch regulator after having violated laws on preventing money laundering and financing terrorism “structurally and for years” by not properly vetting the beneficial owners of client accounts and by not noticing unusual transactions through them.2 Although I only took up my responsibilities in 2018 and violations dated from the past, I was facing a tremendous challenge: how to better protect society while safeguarding ING’s banking license without further penalizing the ING shareholder?
Adding additional people to monitor millions of customers and their transactions was not an option: talent is scarce, (semi-) manual checks are labor-intensive and training of new hires requires too much time.
Building additional rule engines turned out not to be the best solution either. Technology is evolving fast and criminals are one step ahead. More advanced techniques than the one explained below are needed to protect society. As a result, artificial Intelligence was the way forward.
Using artificial intelligence for customer onboarding and transaction monitoring purposes in a large retail bank is easier said than done. As an IT Director, I had to deal with 3 challenges: correctness and completeness of data sources, reliability of the AI model and obtaining approval from the regulator to use the algorithm (instead of the manual checks).
To feed the algorithm with the correct data, sources needed to be consolidated into a “data lake”. Main input providers of the data lake for KYC purposes were, for obvious reasons, customer and payment databases. The main challenge was how do we make sure these data meaningful. For instance, a date of birth in dd/mm/yyyy format and a transaction date in mm/dd/yy format is confusing. A customer address without postal code or country puts the outcome of the algorithm at risk.
A major data cleaning exercise and the implementation of a company-wide Esperanto was to solution to overcome these challenges.
The reliability of the model is the next challenge. How to make sure the algorithm detects all (!) suspicious transactions? One single false negative will further jeopardize the reputation of ING. At the same time, offboarding trustworthy parties or not allowing a faithful person to open a bank account brings bad press too. It’s a bit of a tightrope, really.
At ING, we have been running the AI algorithm in parallel with the human processes for several months. Any discrepancies between the existing process and AI solution have been analyzed in detail to either correct the human analysis or finetune the self-learning algorithm. And after all that work, we were convinced internally the accuracy of the AI model highly exceeded that of the manual operations.
Last hurdle to take… get approval from the regulator. It goes without saying that the settlement not only came with a breach of trust. Several milestones and covenants were imposed as part of the settlement agreement too. As a result, official approval of the regulator was needed before the AI model could start replacing human activities.
Key to success? Co-creation! Involve all stakeholders from the start. Incorporate their feedback and show, evidence-based, the results of the self-learning algorithm. Even then, it takes a lot of time, energy and persuasion power before the final go is given!
Looking back at all of this, I would like to share 3 main lessons learned and/ or best practices. Firstly, many applications for AI in a B2C context are situated in the value creation part of the equation (e.g. recommendation engines). There is many more out there! Companies should leverage AI to capture its value and improve internal efficiency too. Secondly, large corporations with a long history and tradition do take a legacy with them. Leaders in these organizations should continuously invest in data correctness and completeness to make their digital transformation efforts a success. Last but not least, the proof of the pudding is in the eating, definitely in highly regulated environments. Compare the outcome of an algorithm with the results of previous processes or systems. It will increase confidence and keep stakeholders on board at the inflection points of your implementation.
References:
- Financial crime and fraud in the age of cybersecurity: https://www.mckinsey.com/business-functions/risk/our-insights/financial-crime-and-fraud-in-the-age-of-cybersecurity
- Dutch bank ING fined $900 million for failing to spot money laundering: https://www.reuters.com/article/us-ing-groep-settlement-money-laundering-idUSKCN1LK0PE
Tim, this is a great post. It adds more excitement to learn it was your personal professional experience. The settlement of $900 mio penalty is a great case for change and searching for reliable solutions.
Thanks Tim for sharing this experience. Has the AI uncovered any major fraud cases since being enacted?
thanks Tim! I wonder about the fraud split split on B2B transactions versus consumer. In Europe, are fraud rings more prevalent in one space versus the other and how do algorithms manage risk scoring based on those varied profile of customer?