Upstart: Using machine learning to transform the personal loan experience
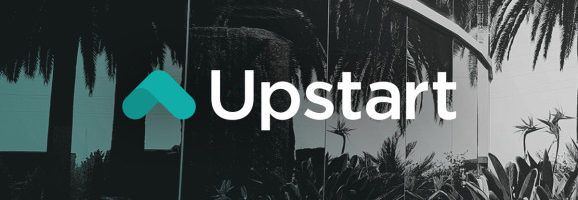
Upstart's mission is to democratize the market of personal loans by using the power of AI technology to simplify customer acquisition processes and automate approvals. However, how far can this technology go to benefit customers and capture the value of growing segments of the economy?
While in terms of market offering there are countless companies offering personal loans to people with short credit histories, like payday companies such as Amscot or Cash Store, the overall consumer experience has been plagued by manual processes to pay back the loans and in some cases, “shark-like” behaviors from lenders to capture loan payments. Due to the limited number of lenders for people without credit histories, consumers tend to negotiate less on the terms and conditions of their loans and accept higher interest rates to compensate for their perceived “low creditworthiness”. Noticing this customer pain point, Upstart and wave of new startups have used the power of digital platforms to disrupt the market and create new offerings. By understanding how Upstart creates value for its users through AI, we will delve into potential new markets where the startup could venture, as well as the potential challenges that it would face as a disruptor.
Value creation from underserved borrowers and capture through machine learning algorithms
Upstart is a startup founded by ex-Googlers that uses the power of AI to provide personal loans to users with short credit histories but regular income and high earning potential. What differentiates this lender from the rest is its ability to use machine learning in the process of underwriting the loans, which is the process through with new users get accepted or denied access to a personal loan. By relying on a larger set of user data points that include non-traditional variables like someone’s GPA in college or the name of the company where a new applicant works, Upstart is able to build a comprehensive profile for risk assessment[1]. As showcased in the graph below, another key to this formula is the company’s reliance on behavioral data that constructs a better assessment of risk, rather than the traditional FICO scores that measure static variables, such as the number of accounts a user has or the average length history of their overall. Despite the lower average weighted scores from Upstart borrowers, even at the lowest end of the spectrum of 600-670 scores, the estimated average annual loss is just under 10% on average, compared to their competitors who face higher annual loss rates higher than 15% on average[2].
The theory behind this trend is that most personal lenders undervalue new applicants with low creditworthiness under standard variables, and fail to understand the economic potential of their users. Not only that, but FICO scores have been heavily scrutinized for their strong correlations to extrinsic variables such as the applicant’s race or zip code, which many deem to be a cause of inherent bias in the provision of loans[3].
Instead of relying on manual processes that may add biased opinions during risk assessment, the company has automated the process of loan approvals. As of April 2019, Upstart has provisioned over $5B in platform originations, of which 67% were fully automated through the ML underwriting process. As the trend progresses towards a theoretical maximum of 99% of automated approvals, one could expect that the ML algorithm would improve over time as it enters new markets and assesses new types of risk profiles[4].
Overall, Upstart’s ability to increase the accuracy of its risk assessment process and its consumer focus on users with high growth potential has allowed the company to reduce operating costs compared to other lenders. Not only has machine learning allowed the company to face lower default rates at similar approval rates, but it has also allowed the company to accept more new applicants at the same loss rate[5].
Future challenges and opportunities of scaling the startup
As ML technologies disrupt traditional markets, regulatory efforts will likely grow as AI-driven lending practices gain visibility. This must be accounted for in the growth strategies of firms in this space. One particular case would be the compliance challenge that traditional firms already face to prove their elimination of bias in the approval process, which is enforced by the Consumer Financial Protection Bureau (CFPB). Despite the company’s ability to obtain a No Action Letter, which is a temporary safeguard that protects “innovative technologies that may bring significant consumer benefit”, this sort of approval is limited to US Jurisdictions, and similar requests would need to be created if the company attempts to expand globally. Also, since No Action Letters are not laws, they can be overturned under certain circumstances and political environments that would be outside Upstart’s immediate contingency plan. As companies face higher scrutiny in a world post-Cambridge Analytica scandals and an increase in data privacy issues, it would is imperative for startups to measure the uncertainty of future conditions as they face new legislative bodies in markets that may not be as friendly to their new technologies[6].
Planning for the future by acting, rather than reacting, to new trends
In a world where millennials continue to be a growing force in the economy, holding over $1.4 trillion in added value, a strategic priority of any fin-tech startup should be to increase their access to new loans and capture the growth of their consumer lifetime value[7]. But, this should include millennials and early professionals from all walks of life, especially those who may have faced bias in the traditional market of personal loans. While machine learning algorithms have been hailed as non-biased ways to make decisions, a new wave of research has shown that they can actually perpetuate similar bias towards specific groups[8]. As such, I would recommend Upstart to look at the growing markets of US Latino and African-American millennials, who represent over 50% of their individual demographic groups, as key targets of their expansion strategy[9]. If it is in their mission to open the floodgates of opportunity to new applicants, then they should be able to devise solutions to improve their ML algorithm and further eliminate the bias already present in the loan provision process and capture the growth of the nonconsumption market. In sum, if the theory of disruption holds any value, it is precisely in its application to growing markets that were previously relegated and to finally realize the hidden gains that technology can express.
Works cited:
[1]https://www.upstart.com/about#who-we-are
[2]https://www.nerdwallet.com/reviews/loans/personal-loans/upstart-personal-loans
[3]https://www.pymnts.com/disbursements/2019/instant-payments-lending-industry-rise/
[4]Ibid
[5]https://www.upstart.com/about#result-to-date-1b
[6]https://files.consumerfinance.gov/f/documents/cfpb_final-policy-on-no-action-letters.pdf
[7]https://www.inc.com/marla-tabaka/forget-millennial-purchasing-power-gen-z-is-where-its-at.html
Some of the challenges for these types of applications is how to avoid the biases that AI creates based on data. In the absence of a credit score history, other available data like ZIP code, age or type of employer for sure can help assess the creditworthiness of an individual. But what if the algorithm ends up favoring one stereotype of users vs others, surfacing gender, ethnicity or nationality biases? Other similar startups have found through AI that people with Android phones (instead of iPhone) is more price sensitive, thus less creditworthy… It seems that tech can act as a double-edge sword, enabling financial inclusion but at the same time discriminating some types of “data profiles”.
Thanks for the well-referenced article, Raimu… I mean Short Apple! It certainly seems like credit startups are becoming increasingly common, especially in countries with less than efficient credit markets. After all, the main advantage that these startups have over incumbents is the ability to price credit risk better using machine learning. I would be curious to know why the larger consumer banks are not doing the same thing. Perhaps it is a regulation issue?
Very interesting article. Agreed with previous postings re: potential discrimination risk. Additionally, am also wondering whether Upstart’s loan decisions can bridge recipients to other sources of capital (mortgages etc.). Specifically, I worry about whether a lender’s use of proprietary and non-industry normalized data makes their decisions irrelevant to subsequent lenders.
My undergraduate college classmate is one of the founders of this firm. I have a vague memory that one of the missions of this company is to reduce the burden of educational debts by offering better terms to students. While I think that the value add is a net positive, I do wonder if the company is ultimately expanding funding to individuals that wouldn’t have had access to it otherwise. From the look of it, basing risk on college GPA, college name, company name, etc might just be giving better priced loans to those who already have access to loans.