Property Insurance in a time of Climate Change
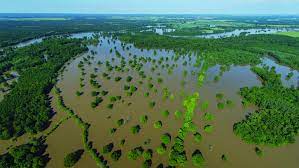
Increasing Access to Insurance and Accurately Pricing Risk
Ripe for Disruption: Property Insurance
The insurance industry is in a prime position to take advantage of big data and data analytics to better understand the risks they are underwriting and how to value these risks competitively. While the underpinning of insurance rests in actuarial science, big data and artificial intelligence represent next-frontier inputs into actuarial modeling. In theory, insurance companies can create value for their corporation and their stakeholders through using big data to more accurately price risks, expedite the claims process and avoid legal costs, and extend coverage to underserved communities.
Property Insurance in a Time of Climate Change
The science of Climate Change indicates that the frequency and intensity of extreme weather events has already increased and will continue to do so over the next 100 years. Many property insurance companies rely on FEMA’s floodplain maps to create a baseline amount of risk for communities. Yet, studies have found that FEMA has been unable to update these maps in a timely cadence, with at least one report finding that less than half of floodplain maps have been updated within the last five years.[1]
Lacking reliable micro-site data, insurers typically price coverage based off of worst case scenarios, instead of most likely scenarios, therefore making the price of insurance coverage out of reach for many property owners. Additionally, by using outdated floodplain and climactic models, insurers are not able to assess areas historically not prone to extreme weather events and don’t have appropriate policies for these regions; examples include the Winter Storm that ravaged Texas in 2021 and Hurricane Sandy in New York in 2012 which led to millions in uninsured property damage.
Scale of Problem
New Construction built in High Risk Areas is Increasing
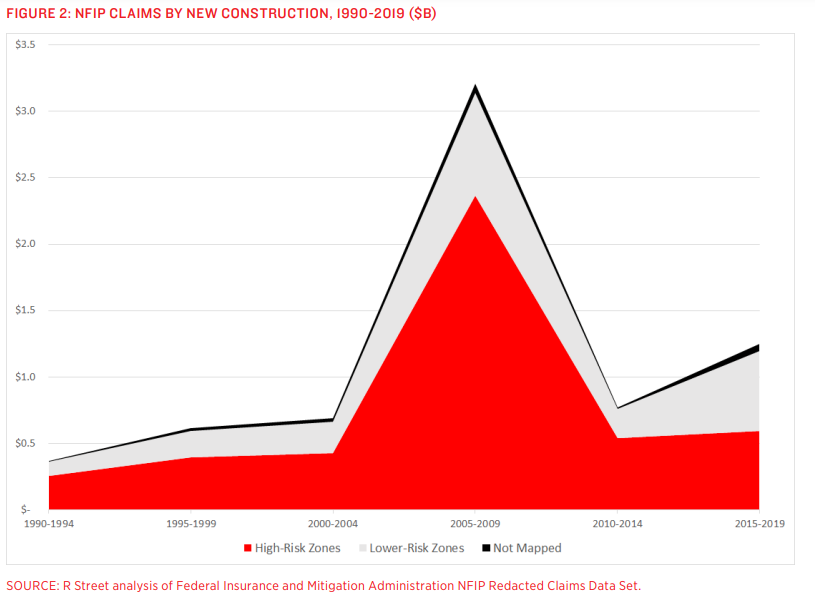
As climate change has taken years for public acceptance and understanding, the last two decades have seen a spike in claims from new construction in high-risk flood zones; by extension, there has also been a spike in new construction in high risk flood zones. In connection with the following graph, showing that the proportion of insured losses is decreasing, the amount of risk due to climactic events on the property market is increasing, and these properties are increasingly under-insured or uninsured.
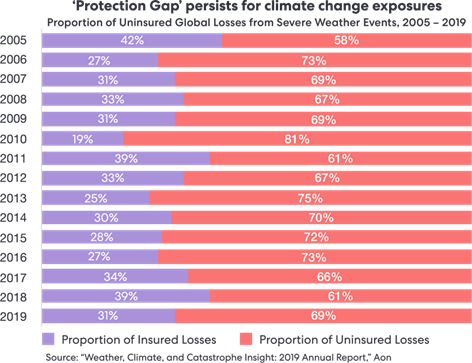
To address this problem of the “protection gap”, Understory is a startup using big data sourced from data from sensors and satellites to more accurately predict climate events resulting in insurance claims.
Where Big Data Comes in: Understory
Technology – How it works
Understory’s data analytics is fueled primarily by their network of wireless solar-powered sensors which capture 125,000 weather metrics per second, such as atmospheric pressure, humidity, solar radiation and hail size, force and angle. These sensors can be installed on residential or commercial rooftops, near electric towers, or on dams or areas critical to indicating flood, wind, or temperature, or other weather related damage.
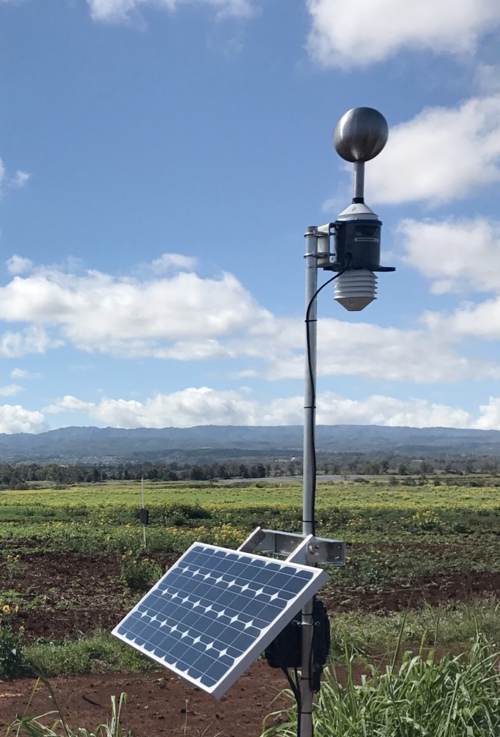
The sensors use solid-state storage and existing cellular networks to transmit data back to headquarters, and essentially operate as extremely compact weather stations. Additionally, these sensors are tamper and fraud proof, meaning their outputs have been recognized as trustworthy by property owners and insurers.
Modeling
Understory complements the millions of data points from the sensors with self-learning atmospheric intelligence and near real time third party radar & satellite imagery, soil characteristics, as well as scientific climate models. Using data analytics to decipher signals from noise, Understory is able to create hyper local or area-wide risk assessments for damage from climactic events in near real-time.
Through Understory’s sensors, they are able to attune risk assessments for micro-climactic and macro-climactic conditions; in other terms, Understory can either use data and predictions from one sensor to assess individual risk to a particular area, such as likelihood of hail damage to a car dealership, or they can use a local network of sensors to predict likelihood of flooding in an entire town.
To elucidate this point, Understory has published a paper about the frequency and intensity of hail storms in the US. From their sensor network of big data, satellite and radar images as well as historical data of hail storms, Understory created a neural network model to help better predict hail events. They found that “annual hail days are significantly increasing since 1979” and their model is able to “quantify the likelihood of damaging hail for any given day”. [3] The graph below summarizes their findings state by state.
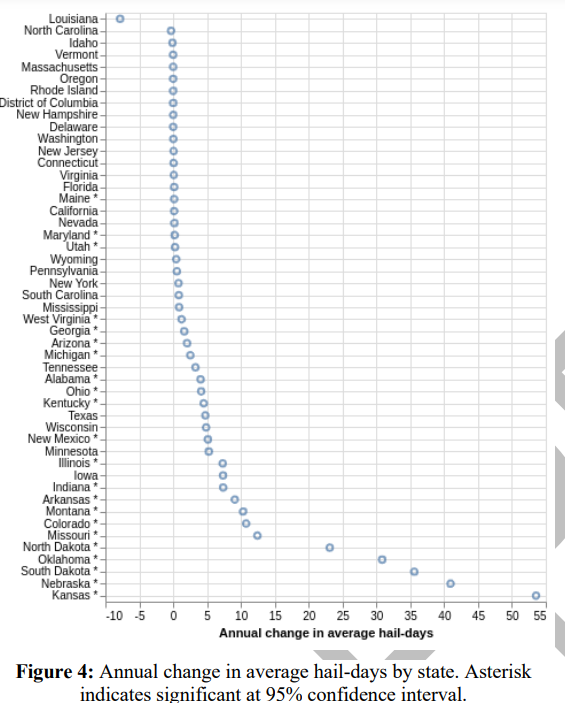
Outcomes
By predicting the likelihood of extreme weather events as well as their intensity and overlaying information such as property value and level of insurance in certain regions, Understory’s model can predict claim amounts before the storm ever comes, therefore allowing for more accurate pricing of climate related property risks.
[1] https://www.rstreet.org/wp-content/uploads/2020/02/195.pdf
[2]https://understoryweather.com/about
[3]https://understoryweather.com/papers/CONUS_Hail_Climate_1979-2018_PREPRINT.pdf
This is such a cool startup! The applications seem most direct in property insurance, but I also wonder if there could be applications in other parts of the insurance industry, such as crop insurance in agriculture, or even severe weather early warning systems for towns/cities.
You’re totally right! One of their other use cases is crop insurance and they’ve won AgTech Innovation Award for their tool’s ability to help farmers understand their likely crop yields. Early warning systems is a great idea too and I’d be hopeful to see them expand their customer set to local or stat egovernments.
Kate this was super interesting! I wonder who actually wins here – warning this is a dark thought. If climate change is resolved does this startup go out of business? Do they or will they face criticism from people for praying on a global crisis?
This was super interesting! I also look at insurance from the flip perspective – their entire competitive advantage is leveraging the mispricing of assets to monetize incorrectly priced risk – but data is growing; risk is becoming (maybe?) more predictable – if that is the case, will insurance even be around?
This is a really cool company! I have a bit of familiarity with the industry as (I believe?) there is the additional problem beyond the lack of micro data is that the standard maps used for assessing price/risk for areas are very out of date (and often in disagreement with each other). That plus the odd incentive system around providing cheaper insurance to higher risk flood zones. (Again could be wrong about that feel free to correct). Thanks for highlighting them!