Predictive Analytics at Intel: Using data analysis to boost sales team performance
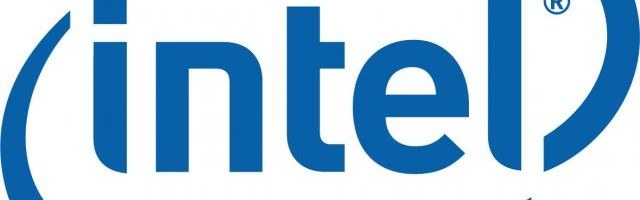
Intel’s sales organization contacted the IT division to help them optimize and improve sales. They wanted to be able to identify which of their customers had the greatest potential for increasing revenues, when was the optimal time in their buying cycle to contact them, and what products or services should be pushed towards them.
Although this sounds like a problem most companies must face, the way Intel’s sales process was developed, made it harder for the sales team to visualize their final customer’s needs, and how to support them in order to grow their business (create value) and be part of it (capturing it).
Intel components are sold to original design manufacturers (ODM) and original equipment manufacturers (OEM), who build the different kind of products: laptops, tablets or smartphones. Then, they sell that product to an authorized distributor, who sells it to a reseller. Resellers are the ones that sell to the end user, and Intel provides them with online support, warranty service and marketing support. Basically, the sales chain looks this way:
The sales team had data about components that were sold to ODMs and OEMs, but they were partially blind after that: they didn’t know exactly the account size of every reseller, which Intel technology were they selling, what products were resellers selling the most, and which of them were better potential target to offer new products and support services.
With this task in mind, the IT team started working on a predictive analytics engine, that could help the sales organization to improve their performance. By using data analysis, they could create additional value by selling more to actual customers or selling to some accounts that were passed by because of this lack of visualization.
To tackle the problem, IT started by creating a data warehouse with all the information they could find about resellers, to create customer profiles. To classify this profiles, Intel used two data mining techniques and apply them together. The first process (called unsupervised clustering) is based on finding hidden patters in unlabeled data. They used an algorithm to identify common characteristics among customers’ profiles, to classify them in clusters. The second technique (supervised classification), adds business rules (based on high volume customers’ characteristics), to make classification more accurate, searching for similarities between known and unknown high sales potential customers. By mixing both techniques, they created a ranking of possible highly attractive customers:
This ranking of customers was given to the sales team to contact. After they engaged with them, the model was fed with sales information, to identify the most important business rules and clustering variables. This enabled the model to be constantly improving, giving more accurate and significant information to the sales team.
The predictive engine has been, in words of Kimberly Stevenson, Intel CIO, highly successful: “By placing together that information, we have been able to (…) identify customers that were more likely to purchase than others. In a very short amount of time, we were able to cover customers that weren’t previously covered and generate millions of dollars of incremental revenues”.
Thanks to this data analytics effort, Intel’s sales force has a powerful tool on their hands, that lets them know who to contact, when to do it, and what should they offer them. Sounds like something every salesperson would like to have!
Great read, AG. Thanks!
Interesting that Intel had to invent this tool, as this problem probably applies to many other companies out there. I wonder if they’ll try to license or sell it to other parties.
Also curious if they’ve considered A/B testing – rather than trying to use existing data. This would take the form of segmenting the potential customers to many groups, along multiple dimensions, randomly allocating sales efforts, and tracking the results. As always, a more costly process, but gives more confidence in the results. With Intel probably having a large sales force, Intel could try many hypotheses simultaneously.