LinkedIn – AI helps companies and professionals find each other faster
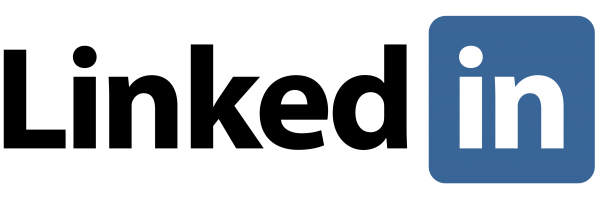
LinkedIn uses AI and the large amount of data to help professionals find the right job and companies to hire the right people much faster than before and hopefully completely eliminate the hiring biases in the future.
LinkedIn is a two-sided platform used for professional networking where people create a profile with their work history, connect with others working in similar industries and follow company profiles. Individuals use it to find jobs and HR to find candidates for open positions.
LinkedIn uses data analytics and AI extensively to improve their members’ and customers’ experiences. Members see it every day from getting job recommendations, suggestions to connect with people to reading useful content in their feed. Enterprise customers such as recruiters are helped from AI to find new talent pools and get informative notifications. The AI systems have had a huge impact on both sides of the platforms creating a lot of value. There was a 30% increase in job applications after improvement of the personalization tool which recommends jobs that members might be interested in. In general, job applications overall have grown more than 40% year-over-year, based on a variety of AI-driven optimizations that have been made to both sides of the member-recruiter ecosystem. The e-mail responses to cold e-mails from recruiters have increased by 45% while at the same time cutting down on the notifications needed to send to the job seekers that have received such messages. Even better, the total number of continuing, two-way conversations started in LinkedIn Recruiter has doubled. Every message a recruiter sends is twice as likely to turn into a hire after the most powerful LinkedIn AI tools are implemented. AI has also improved article recommendations in the feed by 10-20% based on click-through rate. With all of these amazing statistics the platform has reached 660 million users and engagement has increased 50% year over year. The value capture consists in increasing the number of users and specifically paid users and the number enterprise subscriptions from companies.
LinkedIn is also adding tremendous value by using AI to make recruiting diverse candidates a no brainer. Studies have shown that diverse teams see higher profit, have better focus and are more innovative, leading companies to pay more attention to diverse hiring. Unconscious bias can hinder hiring people that are very different from the recruiters themselves which might base their decision on stereotypes rather than the candidate skills. LinkedIn search will bring up diverse candidates and not based on what the biased recruiter might have selected in their previous searches. With the aggregation tools that LinkedIn provides, the companies have better insight on the talent landscape as a whole, such as how skill sets change over time and finding talent pools. Companies can also compare their own gender breakdown to their peers in the industry and identify how to tap into a more representative pool.
Machine learning techniques to detect and remove inappropriate information have been a trend followed by the tech giants lately. LinkedIn has also invested resources in detecting and preventing abuse in a scalable manner. The correspondent AI automatically detect and remediate behaviors that violate the terms of service of the company and maintain a safe and trusted community. According to reports, between January and June in 2019, the platform took action against 21.6 million fake accounts.
There are a few challenges on the AI use. There is an AI talent shortage in the world so scaling the companies is significantly hindered from not being able to hire qualified AI engineers. A centralized organization model helps with having the experts in one place and involving them in different products across the organization using cross collaboration.
The most controversial challenge is critics arguing that such AI systems can introduce bias, lack accountability and transparency, and aren’t guaranteed to be accurate. The AI systems are only as good as the data they’re trained on and the humans that build them. If you train a system using previous hires resumes, the system will inherit both the conscious and unconscious preferences of the hiring managers who made those selections. In order to solve these biases, the designers need to be extra careful on the rules that they use and there should be regular extensive auditing not only during design phase but also during operation. There should be frequent checking on facts such as new hires don’t disproportionately represent one demographic group. Closely monitoring statistics, will make sure that the algorithms stay clear of biased data to train them.
In summary LinkedIn has been using AI to create tremendous value for individuals looking for jobs making it so much easier and for recruiters hiring the right people so much faster. They continue to use AI on helping companies hire diverse people and not allowing spam or negativity in the platform. If they continue to be very vigilant on training the algorithms without biases and hire great AI talent, AI will continue to help both sides of the platform, increase the number of subscribers and capture more profits.
https://engineering.linkedin.com/blog/2018/10/an-introduction-to-ai-at-linkedinh
https://www.businessinsider.com/linkedin-new-ai-feature-increase-diversity-hiring-2018-10
https://analyticsindiamag.com/how-linkedin-is-using-ai-to-detect-handle-abuse/
https://www.vox.com/recode/2019/12/12/20993665/artificial-intelligence-ai-job-screen
Jona, thanks for sharing this. I had no idea LinkedIn used AI, but it certainly does explain a lot. These are some really interesting metrics you’ve provided. However, I wonder how much of these impressive statistics are due to LinkedIn’s AI capabilities alone. Do you know if LinkedIn uses more comprehensive AI technology in the premium version when compared to the free version?
Thanks Jona for your interesting observations! In general I’m a bit skeptical in terms of how much value can LinkedIn create:
1) their recommendation / matching engine is as good as the data inputs are, however, the top candidates on LinkedIn are the ones who don’t worry about looking for new jobs and therefore don’t provide detailed descriptions in their roles, and a lot of top engineers use academic profiles or Google Scholar rather than LinkedIn;
2) ML models are more helpful in increasing the upper funnel and make people aware of the blind spot / unconscious biases, but the accuracy of their predictive models is still limited;
3) a friend who is C-level at LinkedIn said many times ML sit in the backseat unless it can be proved by regression models, as BoD decisions at big enterprises need explainability, which is still somewhat limited today.
Great article Jona – Thank you!
I completely agree with the challenges mentioned regarding bias in the data. As you mentioned, the company should really invest in data cleansing and feature engineering. Even though removing variables associated with bias (e.g. gender, race) could help alleviate this problem, it is enough. A study showed that most algorithms will still find other variables that will lead to the same bias (ZIP codes, club participation: e.g. “women in investing”, etc.), some of which are not obvious. As you mentioned, continuously monitoring your algorithms and actively looking for bias and then adjusting accordingly will potentially help alleviate some of that problem.
Another worry I have, also related to the data, is the common effect referred to as the “LinkedIn Alter Ego”. This refers to most people over-inflating their credentials on LinkedIn which could cause some negative network effects. Could LinkedIn use AI to try to identify these people? or use some sort of other mechanisms to do so? (e.g. manager input, business card scanning)