Kensho – made in the Square, trades on the Street
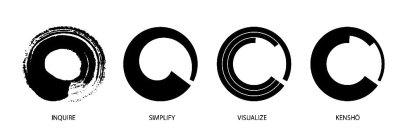
'Ken' means 'seeing'; 'sho' means 'nature' or 'essence'.
👀🌴
True to its name, Kensho employs AI to see the true nature of data. Kensho has disrupted the financial sector and has made its way into all of the major institutions.
Can Kensho continue to deliver value to its clients?
Less than a mile from here, an inconspicuous building in Harvard Square runs some of Wall Street’s most prestigious trading desks. Kensho Technologies has gone from strength to strength, redefining the way Wall Street’s trading desks operate with its vast data and powerful analytical tools.
Fig. 1 Kensho’s headquarters in Harvard Square
Kensho’s market forecasting tools have become ubiquitous. Only a few hours ago, CNBC used Kensho’s advanced algorithms to recommend asset classes for investors to seek refuge in as markets became increasingly volatile[1]. Kensho’s other high-profile clients include Goldman Sachs, Morgan Stanley, Citigroup, and BofA Merrill.
Fig. 2 Kensho’s recommended trades when VIX increases by >5 points
Data -> Kensho -> Value
Kensho’s customer value proposition is twofold. The first draws from Kensho’s extensive data sources. The Kensho Global Event Database constantly assimilates and compiles data about the world’s markets, and the Knowledge Graph restructures this data into a graph-based architecture so that it may be used to garner insights quickly and efficiently[2]. The second core component of Kensho’s CVP is an analytics platform driven by machine learning that offers data analysis and visualization services to clients. The synergy between the two offerings further enhances the value Kensho creates for its customers.
Kensho’s massive database has further been augmented by its strategic merger with S&P Global[2][3][4][5]. S&P’s Market Intelligence data can integrate seamlessly with Kensho’s platforms and drive value for both companies. This synergy is reflected in the increase in S&P Global’s share price by over 50% last year[5]. Despite being acquired, Kensho continues to operate as an autonomous unit from its Cambridge headquarters.
Fig. 3 S&P Global’s shares v/s market (2018 – 2019)
Kensho’s data platforms benefit from same-side network effects. As the number of clients goes up and the data they stream through Kensho’s tools increases, Kensho’s machine learning models predict more accurately and become less biased. Thus, the value derived by the clients increases with the number of clients. Although clients continue to own their data and privacy controls prevent Kensho from accessing any proprietary information pertaining to the client, the nature of Kensho’s models enables these network effects.
A corollary of these strong network effects is the high switching costs experienced by the customers, thereby increasing retention rates and improving the lifetime value of existing clients. These switching costs are further compounded by the vertical integration capabilities offered by Kensho. For instance, one of Kensho’s tools, Warren employs natural language processing to translate simple queries by clients to actionable insights based on Kensho’s datasets[11]. Warren is offered as a SaaS solution on the AWS marketplace so that it can easily integrate with the rest of the client’s workflow.
Kensho also offers other value-added services to clients. In particular, some of their high-ticket clients, including Goldman Sachs, benefit from having customized services that integrate with their internal workflows. The asset management division of Goldman Sachs, for instance, employs Kensho’s ‘cross-correlation engine’ to allow users to track correlations between asset classes in their portfolio[6]. Kensho’s capabilities span across several domains and also enable insights into the effects of geopolitical events on markets. For example, Kensho’s models leveraged historical reactions of markets to populist votes to predict the effect of Brexit on the GBP[6]. More recently, Kensho’s analytics have been widely used to understand the effect of President Trump’s tweets on crude oil prices[7].
Fig. 4 Effect of President Trump’s tweets on different asset classes
Kensho’s value creation opportunities lie primarily in the information arbitrage market[8]. Incumbent financial institutions have historically exploited the information asymmetry from the large swathes of proprietary data they own. However, AIaaS (Artificial Intelligence as a Service) in general, and Kensho in particular, have been instrumental in democratizing access to cutting-edge AI. Therefore, the downstream market that consumes Kensho’s data and analytics has become more competitive, and financial institutions are now being forced to move towards a business model that relies more heavily on value creation for the end consumer.
Fig. 5 Kensho’s business model – shown on a business canvas[9]
Opportunities and Challenges
Kensho’s is clearly a success story. The line between finance and technology is blurring rapidly, and Kensho has cemented its place as a leader in this intersection. With most major financial institutions already within its customer base, however, Kensho is now at a crossroads. While a valuation of over $500 million and the strategic partnership with S&P Global provide Kensho with great opportunities to innovate, Kensho faces some obstacles to capture the value it creates through AIaaS.
One key obstacle is the customers’ bargaining power. The large financial institutions Kensho serves may demand more customized products and services, thereby using up all the company’s bandwidth and resources and limiting its propensity to innovate. Second is their presence on Kensho’s Board. Since the customers are also key investors, and several of them hold board seats, Kensho’s management may have limited autonomy in strategic decision-making[10].
Fig. 6 Some of Kensho’s investors
A third obstacle is the squeeze Kensho will likely increasingly face, as customers aim to organically build out their personalized suite of machine learning-driven analytics products by leveraging the know-how from their relationship with Kensho. Another challenge is the rapidly evolving regulatory environment. As the clamor for ‘explainable AI’ gains momentum, Kensho will have to be cognizant of the challenge it poses. If regulation requires machine learning models to be explainable and transparent to avoid biases that may even upend the entire financial system, Kensho’s models will have to be revamped. This will annihilate the competitive moat they built around them and will leave them exposed to competitive threat from entrants.
Action Plan
To deal with these imminent threats, I recommend the following action plan –
- Product/market diversification – Kensho should actively attempt to build new products or target previously untapped market segments, and diversify their revenue streams, so they are not overly reliant on a handful of powerful customers.
- Board management – While the capital from the major financial institutions may be valuable, Kensho’s management should attempt to create a more unbiased board, so that board members representing customers may not have too strong a position on the board.
- Explainable AI – Kensho will have to work closely with regulators in the different markets they operate in so that they can build machine learning models compliant with regulations that may be imposed in the future. This may also need them to invest more heavily in R&D.
References
- https://www.cnbc.com/2019/12/03/if-we-are-in-for-another-december-market-plunge-here-are-the-places-to-hide-out.html
- https://www.spglobal.com/marketintelligence/en/media-center/press-release/sp-global-announces-strategic-relationship-and-investment-in-kensho
- https://www.forbes.com/sites/antoinegara/2017/02/28/kensho-sp-500-million-valuation-jpmorgan-morgan-stanley/#2f2ad67f5cbf
- http://investor.spglobal.com/file/Index?KeyFile=392478574
- https://www.nasdaq.com/articles/sp-global-spgi-stock-rises-50.1-year-to-date%3A-heres-why-2019-11-13
- https://www.forbes.com/sites/antoinegara/2018/03/06/wall-street-tech-spree-with-kensho-acquisition-sp-global-makes-largest-a-i-deal-in-history/#5eb3b6167b85
- https://twitter.com/HermsTheWord/status/1111318731609772034
- https://www.forbes.com/companies/kensho/#5490ba6c198e
- https://vizologi.com/business-strategy-canvas/kensho-business-model-canvas/
- https://www.kensho.com/investors
- https://medium.com/@eevmiqmagazine/the-future-of-fintech-kenshos-warren-a-i-breaks-records-5e2e00bb2a16
I’m very excited to see this post! I’ve followed Kensho throughout the years, one area where they seemed to initially struggle in terms of customer adoption was product complexity. Users needed training to really understand how to use it. Based on what you’re describing it seems like their business model has shifted more to a B2B (in strategic partnerships with S&P for instance) than a B2C play. If so I wonder what a Porters analysis would say about how attractive this industry is and where it would lead us to in terms of growth. For instance, you mentioned the bargaining power of customers, I imagine S&P would probably like some form of exclusivity and is big enough to enforce it (vs competitors) thereby potentially limiting Kensho’s growth prospects.
Thanks a lot for the post, big data and AI in investments are always extremely interesting, because of immense scalability potential. This case represents an interesting twist but the question that I ask myself is why the model is professional services? They don’t just collect facts but they interpret facts as signals to buy or sell using AI algorithms, so why not just to use it and bet some money if it really works?