January AI: Feeding Your “Digital Twin” to Enhance Metabolic Health
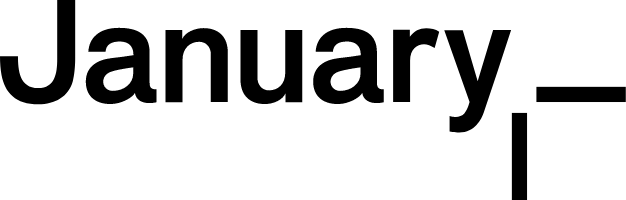
Can ML help to improve metabolic health?
The $327B Metabolic Health Crisis
The US is currently in the midst of what some have dubbed a “Metabolic Health Crisis.” According to the CDC, among US adults 18 or older, 37.3 million people have diabetes, and 96 million US adults have prediabetes. The CDC further posits that the estimated costs from diagnosed diabetes alone was $327 billion in medical costs and lost productivity, with costs expected to surge to $600 billion by 2030.
January AI: Personalized Predictive Analytics for Regulating Blood Glucose
January AI is one of the new health tech start ups aiming to combat the crisis. The company uses continuous glucose monitoring (CGM) in combination with artificial intelligence to help consumers, “…make decisions about what to eat, including predictions about how they might react to different foods before they even eat them.” While other competitors such as Abbott, Dexcom, and Levels Health have aimed to incrementally improve the measurement accuracy or software interfaces for CGM technology, January aspires to replace CGMs altogether with a “virtual continuous glucose monitor.”
In order to enable its predictive analytics, January first focused on aggregating and labeling a “food atlas” comprising over 16 million items. January created nutritional labels and glycemic index ratings for each of the foods as a starting point for its machine learning algorithms. But January quickly recognized that users’ blood glucose responses to food varied based on numerous other factors, including variance in glycemic responses to specific foods, meal timing, exercise, and sleep. To account for individual variation, January’s interdisciplinary team of physicians, data scientists, and engineers spent 3 years refining its machine learning models. The team ultimately applied its model to over 1,000 people during a clinical study, during which January was able to create an individualized predictive model for each participant after 4 days of input. Furthermore, over the course of 10 days, January was able to improve 70% of participants’ time in a healthy target heart rate zone by predicting blood glucose “spikers” and offering precise activity recommendations for blunting participants’ glucose responses.
January has continued to incrementally refine its data science and predictive modeling capabilities to further reduce the friction necessary for glucose monitoring. For one, January has patented its techniques for nutritional inference from text, or its techniques for extracting nutritional labels from menu item descriptions. January has also further attempted to obviate the need for a physical CGM device by creating what it has called a “digital twin” model of its users. After receiving a user’s CGM and other lifestyle data for a brief period of time, January attempts to predict how a user’s “digital twin” would respond to various potential future activities. For example, a user could use January to predict how their blood glucose levels would change in response to certain foods they might eat or certain activity they might engage in later in the day. Alternatively, a user could scan certain foods at the grocery store to see how their blood glucose curve is affected by the food, or a user could see how eating a 1/2 or 1/4 portion might affect her curve. In this manner, over time January aspires to eliminate the need for physical CGM devices in providing users with personalized feedback and insight for managing their blood glucose levels.
Challenges and Opportunities
January faces numerous challenges in gaining widespread adoption of its technology. The cost of physical CGM devices continues to decline, enabling competitors such as Levels Health and Abbott to continue to reduce their prices. Concurrently, January faces challenges with its own product. Because January does not manufacture its own CGM device, and because a CGM is still necessary for initial user calibration, January needs to partner with CGM providers in order to get initial data inputs from users. Furthermore, January is dependent on integrations with CGM providers’ apps to get blood glucose data into its own app, which has sometimes resulted in strange data readings and complaints from users about having to switch back and forth between applications to sync data. Despite these headwinds, January continues to lead the charge towards a future similar to that predicted by Dr. Eric Topol–one in which AI serves an essential function in guiding users towards health.
Louis, this is a fascinating company. It seems super interesting to consider the potential for this sort of data to be used as all the diabetes companies are searching for the holy grail of T1D and late stage T2D – the artificial pancreas. That can be moderate your insulin levels to keep your blood glucose within appropriate ranges. I’d also be very interested to learn how other metabolic information could be used as well to better understand our health (sodium levels, BP, etc.). Real-time tracking could expose so many interesting things we don’t even know to look for!