Insitro: Discovery New Medicines with AI

Insitro is one of the pioneers using machine learning to design and discover new medicines
About Insitro: An AI-Based Drug Discovery Company
Insitro is an AI-based drug discovery company headquartered in San Francisco, CA. Founded in 2018, it has made waves in the biotech industry by merging advanced high-throughput biology and cutting edge machine learning. To date, the company has secured over $743M and entered drug discovery and development contracts with the largest global Big Pharma companies – including Gilead and BMS.
About the AI-Platform Deployment: What is Insitro building and selling?
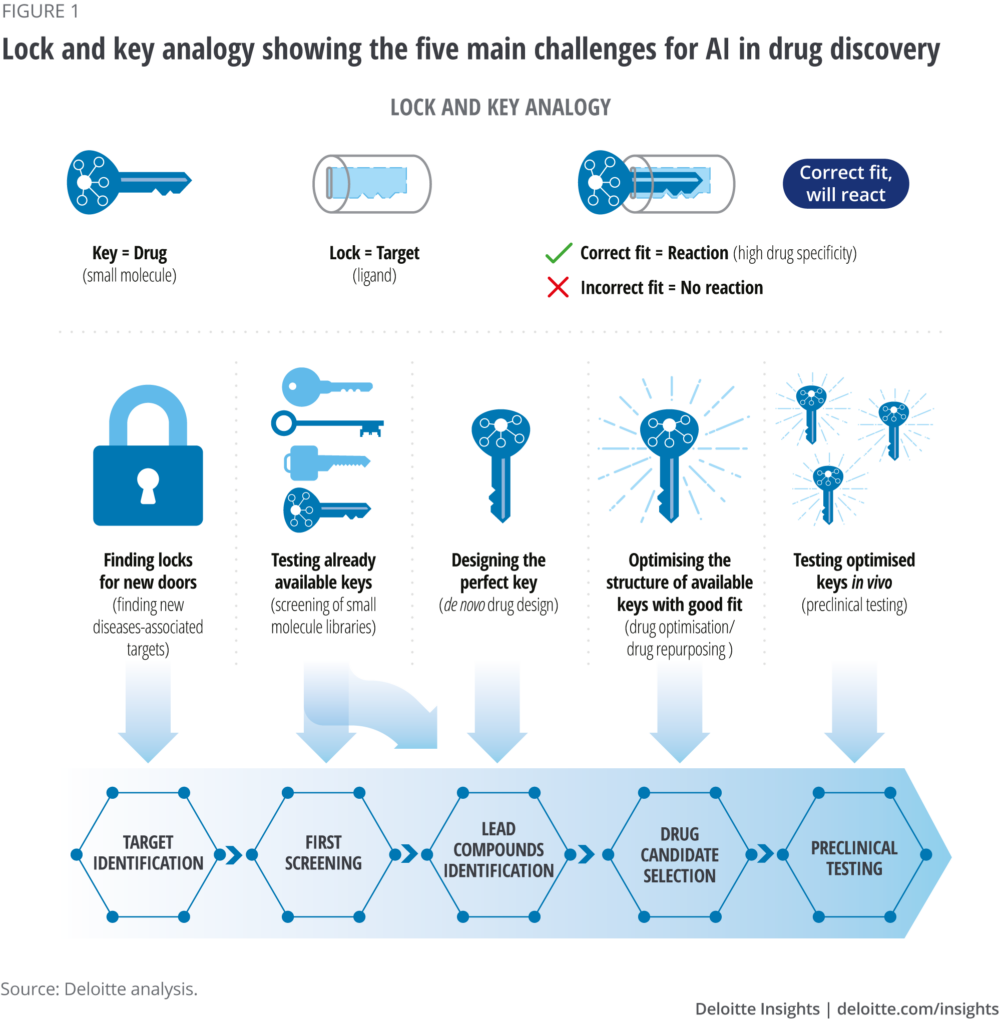
Insitro is building a new process to drug discovery that is disrupting the way drugs are traditionally discovered.
Traditionally, as seen at the top of Figure 1, you can imagine that a drug (or “key” in this analogy) acts on a receptor (or “lock”) in the human body to then prompt a therapeutic effect against a person’s disease condition. In the past, drug companies would run drug discovery experiments where they metaphorically would blindly throw hundreds (if not thousands) of possible keys at a lock just to see which ones might fit.
With the advent of more advanced chemistry and computational methods – particularly machine learning – Insitro has built a more bespoke model. Using large databases of these “locks”, “keys”, and results of those experimental fittings, Insitro can leverage machine learning models to design what the “perfect key” might look like in order to unlock the best therapeutic effect for patients. Based on these designs, Insitro can intelligently design these smart keys and iterate until a final lead drug candidate is identified for in-human clinical trials.
Insitro’s value creation lies in the speed and cost-savings that it offers to its biopharma clients. Industry experts cite that drug discovery platforms like Insitro’s could reduce preclinical R&D costs by 20-40% and increase the probability of success that a drug candidate successfully makes it to market as a potential $1B/year blockbuster product. Across the industry, AI drug discovery platforms are projected to drive nearly a 15% increase in annual drug approvals in the United States, raising domestic drug sales from the existing $300B to $340B.
To-date, with its nearly $750M in funding, Insitro has built both one of the largest drug libraries and databases on drug-target interactions the necessary infrastructure (e.g., complex cell-based human biology models) to continually test these drug candidates on human cells. With both these computational and physical assets, Insitro has positioned itself as one of the leading R&D partners for pharma companies that can deliver cost and time savings.
Challenges & Opportunities for Insitro
Despite the tremendous potential of this field, there are massive challenges for Insitro:
- High requirements for database comprehensiveness to employ ML. For many players, to even generate functional machine learning models, it takes at least 2-3 years to build a large enough dataset of drug-target interactions, drug biochemical data, patient profile data, and clinical trial data and testing records to develop ML models functional enough for a commercial contract with large biopharma clients.
- Lack of industry data standards and labeling. Additionally given the nascent state of the industry, there isn’t a set industry standard on how drug-target experimental data should be measured and recorded. This means that Insitro can’t just acquire public or third-party data to train its drug design ML models. Instead, most of this data collection and training is done in silos – with each AI discovery player competing in its own small fiefdom (e.g., cardiovascular, neurology, dermatology, etc.)
- Regulation. As expected, the pharma industry is heavily regulated, requiring each research, development, and manufacturing process to be auditable by the FDA at any point. Any player in this space must blend the perfect mix of bureaucracy for FDA compliance and innovation as a start-up.
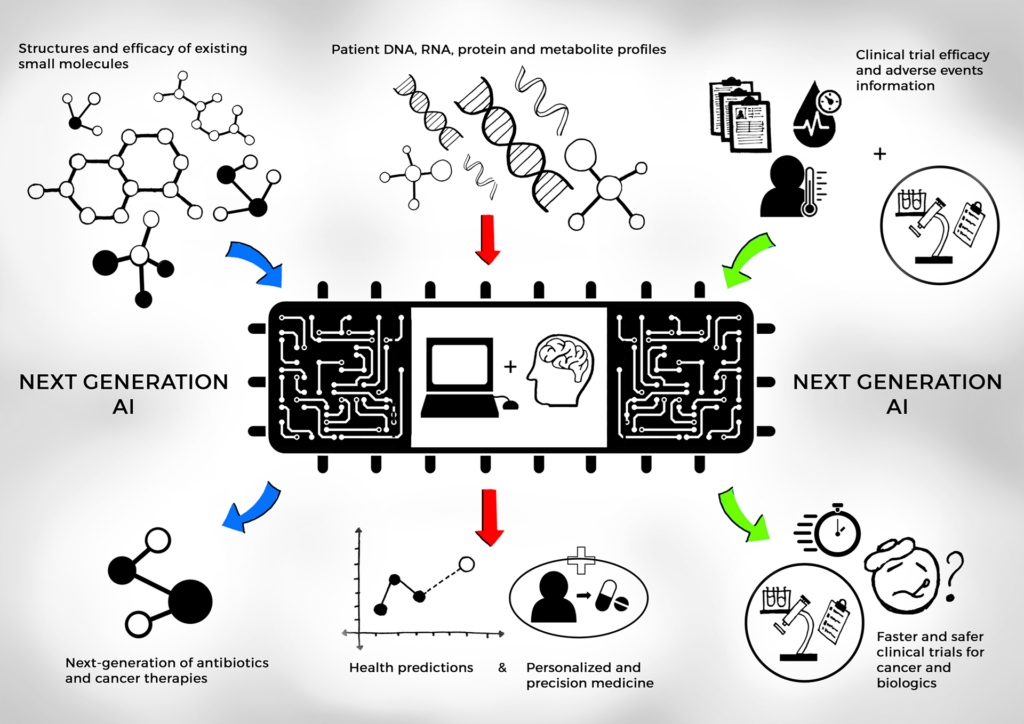
Of course, these challenges also present unique opportunities for Insitro. These high data requirements mean that the barrier to entry is extremely high, preventing competitors in the same subsector. Once one establishes a leading position in a certain discovery area (e.g., neurology), it’s extremely disadvantageous for a competitor to also enter given it would be behind 2-3 years with a weaker data position. And on the topic of poor industry data labeling standards, as the industry as grown in prominence, key market leaders like Insitro have the opportunity to define what is the standard for its competitors and customers. This means that future drug discovery companies will have to design their businesses with Insitro as its model, lending for easier M&A opportunities that are well-fit for Insitro as opposed to its competitors.
Necessary Steps to Achieve Success
I see that in order to both address the challenges and seize the opportunities, Insitro should employ the following steps and changes:
- Invest heavily in database development for highly lucrative disease areas. Given that this industry (or at least the disease related sub-sectors of the industry) is a winner-take-all with a high data entry barrier, Insitro must design its company to focus on a small subset of disease areas with the highest market potential. Spreading itself too widely and accumulating small subsets across a wide breadth of diseases and potential drug-target interaction would be extremely unattractive to pharma partners.
- Build up physical chemistry labs alongside data capabilities. Given the fragmented nature of the company and limited opportunities to acquire useful third-party data, the biggest determinant for success is how quickly Insitro can generate data to train its ML models. Having the complementary physical chemistry and biology labs to test its ML-design drugs and continuously iterate will be essential to building a robust ML drug design model for clients.
- Publish thought leadership to set favorable industry standards. Insitro, being one of the early and most successful players so far, is in a position to set industry standard on data labeling and scientific best practices. By doing so, Insitro can obtain an advantage over its other pioneer competitors because any acquisition for Insitro could be more compatible with Insitro’s data infrastructure than others.
- Employ an organizational design compatible with M&A in 3-5 years. Keeping in mind how acquisitive the biopharma industry is, the company should design is culture and team to be amenable to M&A activity. For instance, making a database that can be easily integrated with new datasets or building a highly collaborative culture.
- Pricing R&D partnerships to incentivize sticky, long-term relationships. Lastly, as Insitro thinks of its customers, because often these R&D contracts last for 5+ years, Insitro should think of its partners not as short-term transaction relationships but long-term sticky engagements. By structuring with lower upfront fees, but later + higher milestone and success payments, Insitro can form relationships that are predicated on the success of the relationship. This meaningful relationship can lock-up its clients and prevent these clients from contracting with its competitors.
Thank you for the post, Patric! I was very excited to read about Insitro, understanding the difficulties of developing new drugs using traditional approaches and how Insitro uses ML to optimize drug discovery, speeding the process while lowering costs. As you mentioned identifying plausible compounds and quickly testing them to see which regions of chemical space are worth additional exploration to predict what molecules are likely to be good binders to a protein target. If they succeed in their mission with all your necessary steps, they will ultimately become one of the world’s most important drug companies 😀
This was super interesting to read! A reason often touted for high drug prices is the costs associated with production and pharma companies need to recoup losses from drugs that did not pass the development phase. If this tech is able to help reduce those costs, I wonder if some cost-savings can be passed on to the end consumer.
Great post Patric, I think its very interesting to think about how Insitro can think about structing their deals with pharma. How can they best align their incentives with major pharma players? Is it worth exploring licensing deals or equity sharing structures (sort of like Ginko) or is it better to price more like a CRO where you provide a service for a fee?
Hi Patrick, thank you for your blog post! I believe this is an area where there is a great need to explore and deploy machine learning to streamline processes and time costs for the pharmaceutical industry. I’m curious if they are starting to look at potential partnerships with drug discovery companies. They seem to need large databases to be able to generate appropriate models for specific disease areas, and I wonder if they plan to focus on a specific class of diseases or if they want to start with broader terms.