How data analytics is revolutionizing the NBA
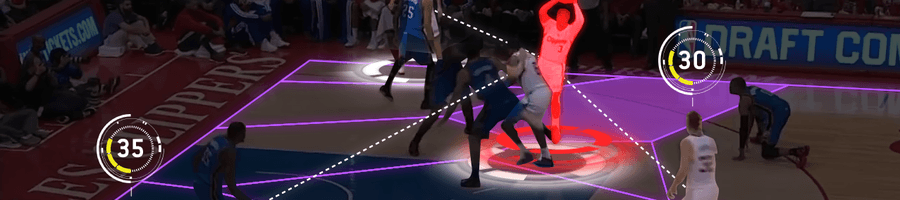
Professional basketball has looked very different over the past ten years thanks to the use of data analytics. It is almost as if these Analytics models have inputted the conventional NBA and outputted an entirely new game. A game where every team now has at least one data analyst. A game where every move is calculated with the aim to optimize for a winning strategy. A game where DATA is king. So how is data analytics being used in the context of the NBA? Is it really creating value or has it turned the basketball game from fun and eventful to predictable and boring?
Value creation through use of analytics in the NBA:
Data has become front and center for NBA games where almost every decision is now based on analytics. Teams have been using high-tech analytics mainly in three ways: designing winning strategies, predicting and avoiding player injury, and scouting.
- Designing winning strategies: the NBA has installed six cameras in every Basketball court to collect granular data on players’ movements. This data is being analyzed by machine learning models to recommend the winning strategy accordingly. Historically, teams were only able to collect basic stats on their players like points scored and assists made, but today, and thanks to the video system installed, teams are now looking at more in-depth data such as the frequency of which players go forward with their right or left foot, or certain ‘tells’ players make before specific moves. This helped coaches better plan defensive strategies based on their opponents, decide on which players should be matched up against who, and recommend “smarter” offensive moves for their players such as how they should be positioned and how to better optimize for a three-point shot.
Breakdown of how Stephen Curry fairs against the rest of the league when taking shots from certain areas of the court. Clearly Stephen Curry prefers to take his shots from the 3 point line and in the paint (area between free throw and basket) - Predicting and avoiding player injury: teams have been collecting sophisticated data points about its players through wearables, sleep monitors, and even saliva samples to asses their fatigue level and predict their performance going forward. The revolutionary application of such data analytics was the “resting” of players even without “obvious” justifications in order to avoid injury. The idea is the more tired a player is, the more prone they are to injury. For example, studies have shown that the likelihood of being injured is less if players rest for 30 days after playing 30 straight games. Hence, NBA teams have been making tough decisions to rest key players to avoid injury (even when the fans don’t like it!).
Example of fatigue report - Scouting: data analytics has become critical for scouting as coaches are now relying on predictive analytics when making draft selections. Drafting is a very important decision in the NBA as teams could be stuck with their expensive selection for years, so it is key that they minimize the risk as much as possible and analytics has been helping with this. Coaches now can easily look up video clips of players to analyze their tracked data (e.g. efficiency with which a certain player drives to the basket with their left hand) and even predict the kind of player they would be (e.g. probability of player becoming an All-Star).
Value capture:
Although there is no direct correlation between use of data analytics and winning, it is clear that teams have already started capturing value from use of data analytics. The biggest game changer to date is the rise of the three-point shot as a result of simple math. Data analytics models showed that three pointers have a 35% chance of going in and hence, could lead to more points than a two-point jump shot taken closer to the basket. As a result, the average 3-point shots taken per team has increased by 50% since 2012.

Moreover, teams are now better at assessing defense. Coaches can identify which players are best at blocking specific types of shots (dunks, three pointers)
Challenges:
Although the NBA has been able to capture value from the use of data analytics by optimizing player performance, it faces two main challenges. First, their games have become too calculated and hence too predictable which is not what the fans are looking for. Basketball is all about suspense and unexpected moves. However, games today have become “boring” and “repetitive” as data models have been predicting the same strategy. This could lead to a drop in NBA revenues where viewership is critical for ticket revenues, sponsorship, and player compensation. In addition to being repetitive, games have been missing their top players recently as data analytics models have recommended to “rest” them. This received a huge backlash from fans who are paying lots of money to see their favorite players on the court. Second, data models do not holistically assess the players’ performance as they are missing key aspects like their psychology, state of mind, and relation with other teammates. For example, how can models predict whether a player will be a good team player? Basketball is predominantly based on the chemistry between players; a player could be a star player but may not excel when put in the wrong team.
Suggestions going forward:
Use of data analytics has definitely changed NBA games, but until data models are perfect, analysts should consider other factors when making decisions especially those that involve human psychology. Moreover, teams should make judgement calls on when they should listen to their models to preserve the “fun” and “unpredictable” aspects of the game.
Awesome article! The shooting chart of Curry is fascinating.
I agree its awful to buy a ticket for a game, and realize after that the star player is not playing whereas he was able to. I think it depends on what the club went to optimize whether the game results or the fans’ expectation.
I am not sure games are too predictive to be boring yet. As you said, these algorithms have limits, but also the public doesn’t have access to as many insights as the teams. For example odds that you can find online are not very trustworthy, as they tend to reflect more the ‘gut feeling’ of the crowd. I believe that data analytics could be used on real time to enhance the experience of the fans, like displaying the shooting chart of players during a timeout.
Interesting article – I wonder, do you think the NBA needs to make changes to the rules based on the use of data? A good example being the increased use of the 3 pointer, which has obviously changed the game tremendously – should the 3 point line be moved back or removed altogether to encourage certain types of players or shot selection?
Thanks Petra for your analysis! Another idea to add additional revenue stream and “fun” for the fans is “sports betting”. It has been around a while in e-sports given the digital native nature, but is also expanding to physical sports which means sports team, analytics providers and sports betters would have more information, more customers and more entertainment. https://sportshandle.com/sports-betting-in-us-data-analytics-industry/
I totally agree with your view that in order to make more informed decision, intangible factors should be taken into consideration as well, psychology, emotions, dynamics with other team members as basketball is a team sport after all.
Great article, thank you! Another potential challenge I see is in data collection. As the low hanging fruit of data collection has passed, to really gain an edge in analytics would require more granular data on individual moves or team performance. While there are technological strides being made in sports data collection, it is still very challenging at times to collect accurate data from live sports events. For instance, some sports data companies that sell data to betting companies will send physical scouts to each individual soccer game and, using their cell phones, document what is happening on the pitch (pass vs. corner vs. foul etc.). It will be interesting to see whether data collection becomes a bottleneck for this exciting industry.