Freeport pioneers data analytics in the mining industry to drive operational performance
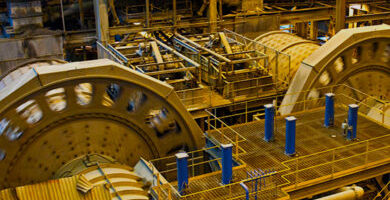
Freeport pioneers data analytics to gain a competitive advantage in a commoditized industry facing cost pressures.
Freeport-McMoRan (FCX) pioneers data analytics in the mining industry to drive performance at its copper operations
Copper Mining Industry Background
Copper is the third most valuable metal used worldwide[i]. Its malleability and conductive properties make it a critical material in industrial and high technology applications such as construction, transportation, and the grid[ii].
Copper miners produce and sell a commodity at benchmark international prices (i.e. they are price takers), and so they compete mainly on operating costs to extract a unit of copper relative to other miners.
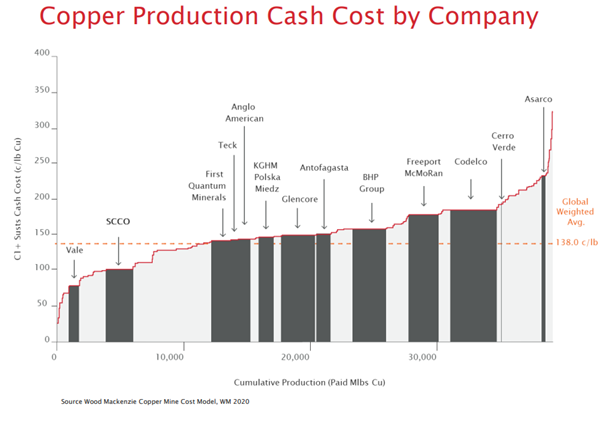
The concentration of copper in ore mined (i.e. copper grade) is a key cost driver for miners. As the best, highest-grade deposits were the first ones to be discovered and mined, the industry has consistently seen a downward trend in grades, as mines age and lower grade deposits are developed to sustain production (i.e. more tonnes of ore are mined and processed to obtain the same tonnes of contained copper, and recovery rates tend to be lower for lower grade deposits). This has resulted in cost pressures in the industry, with miners having to revise their cost structures to remain competitive and profitable.

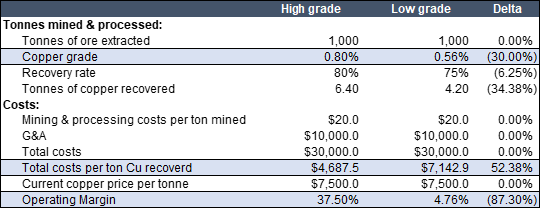
FCX partnered with McKinsey to transform its Bagdad mine in Arizona through AI
FCX’s Bagdad copper operation in Arizona was first mined in 1882. Its current average reserve copper grade is 0.32%[v] (one of the lowest in the industry). As one of its oldest and highest cost operations, Bagdad was selected by FCX to implement data analytics and AI to unlock performance and improve its competitive position. It did so in 2018-19 in partnership with McKinsey, leveraging the extensive historical operating data available for the complex.
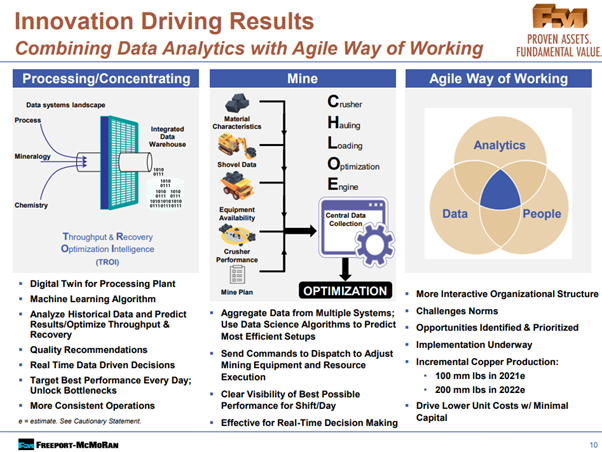
The TROI ML Model
The Bagdad team started by building a ML model to optimize Bagdad’s copper processing mill in terms of throughput and recovery rates. As described by McKinsey, the model “consisted of an ensemble of thousands of decision trees that had been engineered to include a great deal of metallurgical knowledge”[vii]. The operators had historically run the mill as if all ore tonnes fed were homogenous, when its characteristics varied in terms of size, PH and impurities levels, etc.
The model classified ore feed into 7 different types based on data collected from sensors measuring the ore’s different specifications. According to McKinsey, the team’s analysis suggested that modifying the mill’s settings to suit each of the 7 ore types could result in production increases of over 10%.
With FCX management’s support, the team proceeded to develop algorithms that differentiated relationships between ore type, readings from the sensors, production rates, and copper recovered. The algorithms predicted plant performance based on these factors with an accuracy of 96%[viii].
The team iterated and optimized the model (named TROI) with input from plant operators and metallurgists. TROI produced recommendations during every shift to adjust mill settings to maximize output depending on the ore type. Equipment operators and metallurgists ultimately decided which recommendations to follow. As operators implemented TROI’s recommendations and logged their results, the ML algorithms learned which recommendations yielded improved performance and which ones didn’t and the team adjusted the model accordingly. Ultimately, metallurgists adopted the majority of TROI’s recommendations, which resulted in a 10% increase in throughput and 1ppt increase in recoveries.

The success of TROI at Bagdad motivated FCX management to roll-out similar initiatives at their America’s operations that are estimated to increase the company’s copper production by 90kt of copper per annum[x] (an incremental $675M in revenues p.a. at today’s copper prices) without the need to deploy multi-million-dollar capital projects to open new deposits that would also scare the environment permanently. The FCX team has since bolstered its data-analytics and agile working capabilities and reduced their reliance on external consultants.
Some critical decisions from FCX allowed Bagdad to leverage data analytics to improve performance:
- Built a central data-science group and a company-wide data warehouse that logged operating information in a standardized manner.
- Installed sensors to collect data from equipment and processing facilities across operations.
- Management supported data-analytics initiatives and fostered a culture that empowered operators and encouraged them to test with operational changes using a data-driven, agile approach.
The operational and financial outcomes of introducing data analytics across FCX’s operations has been remarkable and a landmark for the industry. To achieve this, FCX had to overcome non-trivial challenges:
- Change in culture at operations, where experienced frontline operators were accustomed to old ways of doing things and focused on maintaining operational continuity given significant losses in case of operational disruptions.
- Implement new technology and processes at operations that have high safety hazards without compromising operator’s health & safety.
- Get buy-in from plant operators to utilize “black-box” models to adjust mill settings based on recommendations that sometimes contradicted long-standing practices.
- Bring outside consultants to supplement data-analytics capabilities and introduce agile working methods, which could have been taken as threatening to long-standing operators.
- Risk missing production and cost guidance provided to investors when testing ML model.
Overall, FCX set an example of how to successfully introduce AI and data-analytics in an industry that faces significant cost pressures and that has been historically inflexible when it comes to innovating in production processes and mine management.
[i] Statista: https://www.statista.com/statistics/655194/commodity-metals-global-market-size/
[ii] Geology.com: https://geology.com/usgs/uses-of-copper/
[iii] Southern Copper February 2022 presentation: https://southerncoppercorp.com/wp-content/uploads/2022/03/p220215.pdf
[iv] MOI Global: https://moiglobal.com/copper-mining-articulating-a-contrarian-thesis/
[v] FCX Annual Report: https://www.annualreports.com/HostedData/AnnualReports/PDF/NYSE_FCX_2021.pdf
[vi] FCX investor presentation Q4 2019: https://s22.q4cdn.com/529358580/files/doc_presentations/2020/FCX_4Q19_Conf_Call_JAN20.pdf
[vii] McKinsey “Inside a mining company’s AI transformation” report February 2020: https://www.mckinsey.com/~/media/McKinsey/Industries/Metals%20and%20Mining/Working%20With%20Us/How%20we%20help%20clients/Inside%20a%20mining%20companys%20AI%20transformation/Inside-a-mining-companys-AI-transformation-vF.pdf
[viii] Ibid. McKinsey report.
[ix] Ibid. McKinsey report.
[x] IM mining: https://im-mining.com/2020/01/24/freeport-invest-data-science-ai-programs-north-south-america-mines/
Thanks for the great content, Carlos!
What FCX accomplished by deploying data analytics in its mining operations is nothing short of extraordinary. A few questions come to mind:
1. Would FCX be able to develop and enact such an initiative without having to hire external consultants (McKinsey)? I think this question is important as we think about potential avenues of disruption for more ‘traditional’ industries, and how they may or may not be well-disposed to new ways of doing things (ie. data analytics).
2. How scalable are the AI models that FCX developed for the Bagdad mine? Can they quickly and efficiently be implemented in other FCX mines across the globe, or do they need months worth of work before being deployed somewhere else?