Driven by Data: HUK-Coburg’s Road to Insurance Success
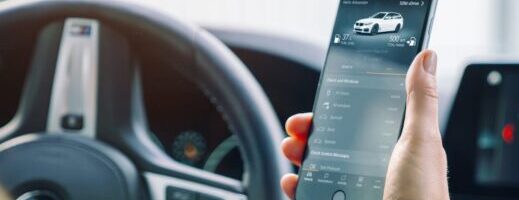
🚗📊 Unlocking Auto-Insurance Innovation: HUK-Coburg leverages telematics & AI to revolutionize premiums based on driving behavior 🛣️💡
Advanced telematics systems that track granular driving behaviour – paired with artificial intelligence algorithms that can predict user-specific risk from big data – have the potential to change the auto-insurance space. Though the US was by far the largest market in the initial years of adoption (McKinsey, 2018), many other countries have caught up as auto-insurers have recognised the value of big data and begun offering consumers incentives to install physical telematics devices in their cars, or apps that track granular acceleration, velocity, and geolocation data on their phones.
As one of the few insurers that offer a telematics option in Germany, HUK-Coburg — Germany’s largest auto insurer — hopes to capture value by adjusting premiums based on behavioral data. To incentivise drivers to download their telematics app, they offer up to a 30% discount off future premiums for good driving behaviour. Granular telematics data has the potential to create value for the company in several ways – first, safe drivers receive lower premiums, which encourages them to continue safe driving practices. Existing literature suggests that when telematics is offered, there is both selection into monitoring, and a positive effect of monitoring on driving behaviour (Jin and Vasserman, 2021). Telematics provides extra monitoring, reducing the risk of moral hazard and therefore the value of insurance claims. The value of telematics data can go beyond its effects on driver safety – a person’s data is useful for figuring out their “type”, imparting useful information on how to price future premiums. Moreover, there may be spillovers – e.g., knowing the driving behaviour of one particular driver might tell insurers something about who was at fault in an accident, and the driving behaviour of others similar to them (e.g., through collaborative filtering ML models), even if they had not opted into telematics. In that sense, their data can also potentially help HUK-Coburg determine how to price the premiums of those who did not opt into telematics.
However, there are several challenges that come with structuring and pricing optimal incentive contracts, especially when customers may face several behavioural biases that might affect their choices of whether or not they opt-in (or drop-out of) telematics, as well as their driving behaviour. In a country like Germany where privacy concerns are paramount, consumers may be reluctant to opt into the app even if the monetary benefits are high. Moreover, HUK-Coburg currently observes that those with lower predicted driving scores are more likely to drop out of telematics: this could potentially be from the disutility customers get from seeing that they are bad drivers, or the fact that they might update their beliefs downwards on whether on not they will get a bonus (therefore giving up data becomes less worth it relative to the costs of privacy concerns, or other demand frictions). Drivers may mispredict their own future driving behaviour, e.g., due to illusory bias (Horswill et al., 2004), potentially leading to higher opt-in than expected at the time of contracting but contributing to high attrition rates. Understanding why drivers drop out would be important towards the long term generation and renewal of telematics data as an asset.
Additional opportunities are also rife in this space. HUK could look at the corpus of granular data (trips, distance, driving hours, velocity/acceleration data, geo-location and associated road limits, accidents, etc.), and to predict what might actually be causing accidents that lead to high insurance claims. This would help HUK-Coburg refine what type of driving behaviour to specifically target, and to improve targeting alerts to drivers, as well as messages that help improve fuel consumption. Previous work has looked at the effects of warning text messages on handheld phone use behaviour (Jin, 2023), but there are many other behaviours to target. Moreover, there is a dynamic tension between limited attention (if alerts pop up frequently, drivers unlikely to respond to all of them) versus the costs of unsafe driving. How should HUK optimise and personalise messaging to drivers? There are many opportunities to improve outcomes for both drivers and HUK-Coburg here.
Lastly, there are many other ways to potentially structure this contract that could capture more value for HUK: for example, they could offer an even lower base premium and penalise bad drivers. Or one could reclassify customers in future periods, awarding the equivalent of both bonuses and/or maluses without making their counterfactual premium explicit. Behavioural economists have often proposed that loss aversion can incentivise higher productivity or other desired behaviour – e.g., if drivers are more averse to getting a penalty than when presented with a similar bonus, they might be more predisposed to drive safer. However, previous work has also found that in certain contexts, “loss framing” incentives can lead to “gaming” behaviours that lead to negative consequences (Pierce et al., 2022). In this case, loss aversion may help on the intensive margin – getting drivers to choose safer driving behaviour contingent on opting into telematics – but may lead customers to opt out of monitoring on the extensive margin, perhaps forgoing telematics altogether. HUK may have to consider other biases that are relevant in the setting of insurance contracts (see Baiker et al., 2015), including present-bias, where customers may value a high discount now whilst discounting potential future losses. There exists a tension here: whilst a firm would want to set a low base premium to attract a wider set of customers – especially if they face present-bias – loss aversion might drive customers away from accepting a plan with penalties. Any incentive structure that involves a malus will have to take into account these competing effects.
HUK-Coburg’s strategic use of telematics data and behavioral insurance principles positions them as a leader in the auto-insurance industry. By continually investing in technology, addressing consumer concerns, and adapting to regulatory changes, they are well-positioned to capitalise on the opportunities presented by data analytics.
Great blog, Ruru! I didn’t know this was being done in Germany- it was a great read. Not sure it came up at all in your research, but given the privacy risks, do you think it might make sense to start with commercial transportation (i.e., trucking) first? I don’t really know how insurance for large trucking corporations or drivers work, but I wonder if it could be a good POC for HUK-Coburg to use to test before going big.
Really interesting topic Ruru, nice job! Do you think as insurance companies gather more data around drivers through these opt in telemetric programs, we will likely end up in a situation similar to the lemon market for cars? Essentially, if all good drivers benefit from enrolling and are incentivized to do so through discounts, theoretically, the only ones who don’t enroll will be the bad drivers. So in that case, they don’t need everyone to sign up, just enough to start to draw that conclusion. You alluded to it already with companies using similar demographics to classify/evaluate drivers who aren’t on the program, curious if you think this is a logical end state or if we won’t end up there.
This is definitely an interesting read on how a company can utilize data to change the insurance premium space. I heard about Progressive in the US doing a driving-usage based car insurance plan, and I would be curious if there are any opportunities for cross-collaboration between the companies, or at least sharing of best practices. Especially since HUK-Coburg is one of the few that does this in Germany, this would be a great way to mitigate some of the potential risks you identified that are associated with tracking driving behavior. Thanks for sharing!
Thanks, Ruru! The issues you mention are very relevant. It’s interesting how behavioral biases significantly impact the implementation of telematics-based insurance contracts.
Considering the complexities and biases mentioned in the blog, I wonder how might HUK-Coburg effectively navigate the balance between offering attractive incentives for safe driving behavior and addressing customer concerns, particularly in a market like Germany where privacy is a significant consideration. I’m also curious how they would account for changes in behavior and potential biases if they were to apply this model in a global context.
Great minds think alike haha! I actually wrote about something similar in my Tesla post, given that Tesla has now started offering insurance in select states off of what is essentially built-in telematics data.
I like your analysis of the self-selective behavioral issues that arise from opt-in telematics, and I have to say that I’ll be surprised if telematics are not made mandatory at some point. After all, current insurance premiums are priced based on age, gender, and other demographics data- it’s imperfect and contains a fair amount of bias. What better system to gauge driver risk profile than to literally observer the driver actually driving? It’s true that the premiums for bad drivers will go up…but in an accurately priced insurance system, they should be paying more. The fact that they are currently being subsidized by better drivers in the present scheme is unsustainable, and I hope that this new trend can actually cause drivers to drive more carefully because they have no other choice if they do not want to be labeled a more dangerous driver.