DeepL – Neural Networks for Translations
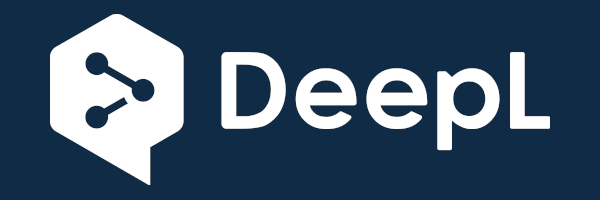
The small company that has outdone TechGiants like Facebook, Microsoft and Google with their translation tool using AI and machine learning.
DeepL – Better Translations based on AI
The companies mission statement is to become Europe´s leading AI company. DeepL was founded in Cologne, Germany. Since the translator was launched in 2017 is has been setting records for machine translation quality. It is the 146th most visited website globally based on Alexa and more than a billion people have used its services.
How does DeepL AI ensure this quality of translation?
DeepL uses deep learning on the basis of neural networks to translate. The researchers in the DeepL team explain they were able to make improvements to the overall neural network technology in four areas:
1. Network architecture
The translator uses artificial neural networks, which are trained on millions of translated texts. According to the company, DeepL was trained using one billion translations. These are bilingual sentences that Linguee’s web crawler has collected from the Internet. DeepL improved the network architecture quality by internally training and comparing the architecture. One of the crucial differences to earlier translations is that neural networks do not translate word by word but have the whole sentence in mind. Convolutional neural networks have many layers that allow them to process all the words in a sentence in parallel rather than one after the other. This makes the DeepL translations faster and more accurate.
2. Training data
The training data for DeepL was focused on achieving higher translation quality. The company developed crawlers that search the internet for translations and assess their quality. In comparison to their competition, they had to make an effort to find training data, because they are not a major tech company with access to lots of training data.
3. Training methodology
DeepLs network compares its own translations with those from training data. It does this repeatedly and adjusts the weights of the network accordingly. On top of that other methods of machine learning are used.
4. Network size
DeepL claims they focus on efficient use of the parameters with which they train the network. This is what allows them to achieve translation quality with a smaller and faster network. This allows the value creation for the consumer. The translations not only have high quality, DeepL can also work with high volumes in seconds. Users value how close the translations get to the natural speech used in the country. All the translations make sense and sound correct, compared with some other translators a big advantage. DeepL can save users time, because they don´t necessarily need to go over the full translation again to double check it – DeepL provides high accuracy and is reliable and consistent.
Users have very low multi homing costs for translators. The switching costs are also low. Users could easily go online and translate the same text using different translators, but the DeepL AI offers the most important values for translations of consistent high quality and high speed that creates a user experience which makes users come back.

How does the company capture value?
The majority of the DeepL users use the free version of the translator. The company captures value through advertisements on the free translator website. But DeepL works with a freemium model. DeepL released a pro version in March 2018, within one year of the launch of the free translator. DeepL Pro allows users access to the full capacity of the AI technology. Subscribers use an optimised web translator, integrate algorithms in translation software and new apps or services with the API. The pro version also offers tighter data security.
Current Opportunities and Challenges for DeepL
DeepL runs billions of mathematical calculations and needs computing power sufficient for this. Together with Verne Global, they build a data center in Iceland. The cool climate and abundant energy offered a solution with low-cost power. The supercomputer, according to DeepL press materials, is the world’s 23rd largest, capable of translating a million words in under a second. With increasing usage of the translator and improvements of the features the computing power might need to be increased as well.
Do we need the app? – DeepL offers the translator on the website and on the app. There are a few main advantages of using the app over the web interface, like shortcuts, faster document translation, saved translations, automatic text capture, faster alternative words, or instant insert.
Despite these advantages users often prefer the online version for translations, so they don´t need to download the app and take time to set it up. This raises the question going forward if it is worth for DeepL to keep improving the app and putting time and money into it.
Current challenges that the company faces include limited coverage outside of European languages. DeepL already added many new languages successfully, but they could offer even more languages than nine. The translator can also be a little more expensive than other services depending on a customer’s needs. DeepL is hiring engineers, product developers and accountant and customer service functions at the moment. The company is growing and could think about expanding to new product categories.
A major opportunity for value capture for DeepL could be an expansion and focus of their client base to enterprise customers. These customers can use the service for example to translate full WordPress websites with the plugin. This gives the opportunity to create a multilingual website without any technical knowledge. They could also focus more on developers. Developers can build their own custom implementations. A change of focus of the target group would be connected to a change in the company’s business model to recurring revenue from subscriptions.
References
Vielen Dank, dass Sie dies mit uns teilen!
As an international and language-enthusiast, I have experienced the long evolution of online translators from not really useful / too literal (nonsense word by word) to the current stage that seems pretty good basic functioning. I’m curious how this performs against Google Translate (my default) in a head-to-head match on multiple scenarios of conversational/informal text and more technical/industry-context content. The way it was designed and trained to prioritize quality makes me think that the focus on enterprise customers seems like a great opportunity, but I wonder how competitive and sustainable the freemium model could be against giants like Google and Microsoft that benefit from deep pockets, massive data and embedded/convenience in many daily products.