Data analytics is a blow to global warming
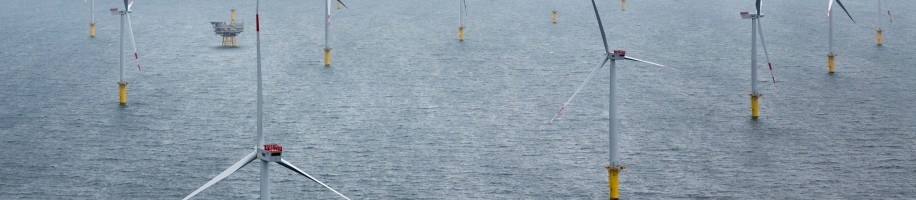
Siemens has built a data analytics team to increase efficiency and reduce maintenance costs in its wind power business.
Wind power plays a critical role in transitioning from fossil fuels to renewable energy production. However, efficiently operating wind turbines with a diameter of over 500 feet in remote off-shore locations is a huge challenge.[1] Siemens, the market leader in manufacturing wind turbines, has built a data analytics team to increase energy production efficiency and reduce maintenance costs in its wind power business. Along the way, the company has developed new ways of creating and capturing value in an intensely competitive business environment.
Value Creation and Value Capture
The traditional way of creating and capturing value in the wind turbine business was straight forward. Companies like Siemens focused on design, manufacturing and installation using their engineering capabilities and global scale to earn attractive margins from selling hardware. As incremental returns from these traditional levers of value creation have diminished over time, Siemens has put more and more emphasize on helping customers to efficiently operate their wind power asset. The company now captures significant value from service contracts offering technical maintenance and optimization of power production. However, creating value by servicing wind turbines requires a very different operating model in which data analytics plays a key role.
Operating Model
Siemens opened a data analytics center in September 2014 to collect and analyze the data of more than 7,500 wind turbines located all around the world.[2] Every turbine is equipped with up to 9 sensors constantly feeding the center with data on the weather, the power grid and the vibration of main components such as the rotor blades. Around 3,200 parameters are monitored for each turbine and the center’s dataset is expected to grow to 268 terabytes by the end of 2015. This data is analyzed by a team of 130 data scientists with two main goals: detection of defects to trigger maintenance and optimization of power production.
Data Analytics for Maintenance
Maintenance is the main factor influencing a wind turbine’s operating costs. This is particularly true for off-shore wind parks or turbines in equally remote locations. A team of highly specialized engineers has to climb a shaft that is a couple of hundred feet tall to fix a rather complicated piece of technology often under harsh weather conditions. To reduce maintenance costs the Siemens data center uses predictive analytics and machine learning to detect defects long before they happen. The vibration data of every wind turbine is constantly compared to a growing reference data base of vibration patterns. By detecting tiny deviations from normal operations and checking the reference data base for past instances with a similar pattern, the data scientist can predict when a turbine is likely to fail and what might be causing the defect. Maintenance engineers provide feedback for every defect they fix to constantly improve the algorithm similar to companies providing feedback on an employee’s performance at Aspiring Minds. This allows Siemens to schedule maintenance work much more efficiently and shut down wind turbines before they are seriously damaged. Furthermore, data scientists can also distinguish different types of defects to decide if a maintenance team is really needed or whether the issue can be resolved remotely for example by simply shutting of a turbine for a certain period of time. Today, 85% of defects can be fixed remotely from one central location.
Data Analytics for Optimization
More recently the Siemens data scientists have started to use a similar approach to optimize power production by comparing patterns of operating data with weather data.[3] The vast amount of data they are gathering allows them to constantly improve the settings of rotor blades for various conditions such as small changes in sunshine or haze. This has led to an average increase of 1% in annual energy production.[4] Although this doesn’t sound much, a similar effect is very hard to achieve by traditional ways of optimizing the design or choice of materials.[5]
Outlook
Siemens is certainly enjoying a first-mover advantage by applying data analytics in the wind power business. The company’s vast dataset and machine learning algorithms enable constant improvements which is making it hard for competitors to catch up. However, by obtaining an increasingly complete picture of all kind of defects and by moving closer to the optimum settings under all weather conditions Siemens will likely experience diminishing returns on data analytics at some point.
[1] http://energy.gov/eere/wind/offshore-wind-research-and-development
[2] https://www.siemens.com/innovation/en/home/pictures-of-the-future/digitalization-and-software/from-big-data-to-smart-data-remote-service-wind-turbines.html
[3] https://www.siemens.com/innovation/en/home/pictures-of-the-future/digitalization-and-software/from-big-data-to-smart-data-machine-learning-in-windturbines.html
[4] https://w3.siemens.com/topics/global/en/events/hannover-messe/program/Documents/pdf/Smart-Data-to-Business-Michal-Skubacz.pdf
[5] http://biarri.com/optimising-big-data/
Great move for them to make these more like “smart” devices. If you have the data, you might as well use it, right? I agree that a 1% improvement is nothing to scoff at. With how variable weather is, I’m not sure whether they will figure out the “optimum” solution for every possible weather scenario, but rather be in a constant state of adjustment (perhaps this could be automated at some point). I wonder if the data that they’re collecting could be useful for anyone else as well — they have a huge network of these devices capturing weather data, but more importantly the changes in this data over time. It seems like this might be useful in some sort of modeling or predictive behavior that weather and government organizations might find valuable. I will be interested to see if they could get an additional revenue stream through the sale and provision of this data.