Capital One: Transforming traditional banking to an AI-first experience
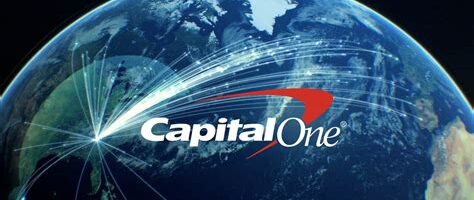
Capital One’s history of adopting an information-based strategy is helping it achieve an unassailable competitive lead as an AI-first financial services firm leveraging machine learning for delivering superior customer experience.
Ever since Capital One Financial Corp. was founded as a credit card company in 1988, it has focused on leveraging data analytics to understand consumer spending patterns. As a diversified bank with more than 65 million customers, Capital One has been a pioneer in digital technologies. It was the first bank to appoint a chief data officer in 2002 and by 2011, was conducting 80,000 big data experiments a year.
Capital One has leveraged these historical strengths to become an AI-first company in the past ten years and transformed itself into an agile, cloud-native, large-scale engineering organization.
Capital One’s holistic AI-powered approach
Today, Capital One applies AI/ML to almost every facet of its business; from customer-facing personal assistants, to call center operations, and even pre-emptive maintenance of its digital assets apart from credit decisioning.
Real-time, Intelligent, Automated Customer Experiences
Eno by Capital One, was the banking industry’s first, natural language SMS chatbot that has evolved into a multichannel solution with numerous capabilities. It helps customers check their bank balances, track purchases, pay bills online, connect with spending, and proactively monitor their accounts and keep them secure.
One of the recent additions to Eno is a fraud detection engine. Eno automatically alerts customers about potential frauds in real-time, and if required, locks their card. Eno uniquely communicates these decisions proactively and allows customers to respond in their own terms and words. This unlocks a critical network effect of customers training Eno with more data about their intentions, which in turn improves the algorithms, leading to lesser frauds and a better, seamless customer experience.
Enhancing user experience around virtual card numbers through EDGE ML
While making online purchases, the Eno browser extension binds a unique virtual card number (VCN) to a specific merchant in lieu of the customers’ physical card numbers. Each VCN mapped to a specific merchant provides safety to customers against original physical card details being leaked in case of a breach. Eno browser is required to identify payment pages and fields using rules and autocomplete payment processor patterns. However, non-standardized HTML markups on payment pages of different merchant sites make it necessary for Eno’s rules to be configured for each merchant site. Capital One’s Eno team solved this problem by creating two models; one detects when the customer is on a payment page so that the Eno browser extension can be popped up, and the second classifies input fields within the payment page so that the Eno browser extension can automatically fill in the appropriate payment information.
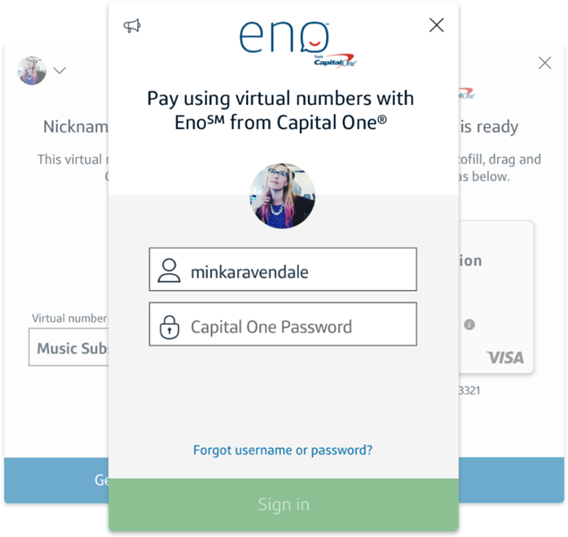
These models have enabled Capital One to seamlessly extend their VCN to a large number of online sites thereby improving response times while preserving customer privacy and security.
Using ML for quick turnaround during mobile app failure
Capital One Mobile App is an integral part of the firm’s strategy to deliver seamless, digital services to its millions of customers. This requires the firm to be highly responsive in resolving technical failures to minimize credibility loss in such an eventuality.
To achieve the same, Capital One has created an ML-based intelligence layer on top of its industry-leading monitoring tools observing mobile app failures. Capital One mobile app is essentially a complex interconnected system built as a mesh of APIs, microservices, databases, and compute & storage infrastructure. Monitoring tools capture these components’ health but store that data in a siloed manner. So, Capital One’s ML team created an inference engine by integrating these siloed datasets from different monitoring tools and linking it with appropriate metadata to help engineers identify the failed component with its reason. This enabled them to reduce the turnaround time for resolving incidents by up to 50%, thereby giving Capital One a major competitive advantage.
Machine learning for anti-money laundering
As per the United Nations Office on Drugs and Crime (UNODC) estimate, 2.7 percent of global GDP is being laundered. Capital One, like many other traditional banks, used rule-based, deterministic tools to monitor transactions for money laundering. However, this required the AML team to constantly keep adding parameters and conditions for triggering alerts, making it difficult to maintain the system. Also, these alerts weren’t prioritized for investigators based on contextual parameters which needed machine learning intervention.
Capital One formed a multidisciplinary team of AML experts and data scientists to train a random forest model on a hundred thousand past investigations and validated it using a several hundred features based on customer and transaction attributes linked to money laundering activities. The model processes a customer’s identifiers to generate a score that represents the likelihood of a customer’s activity being suspicious and allows AML teams to prioritize alerts for further investigations.
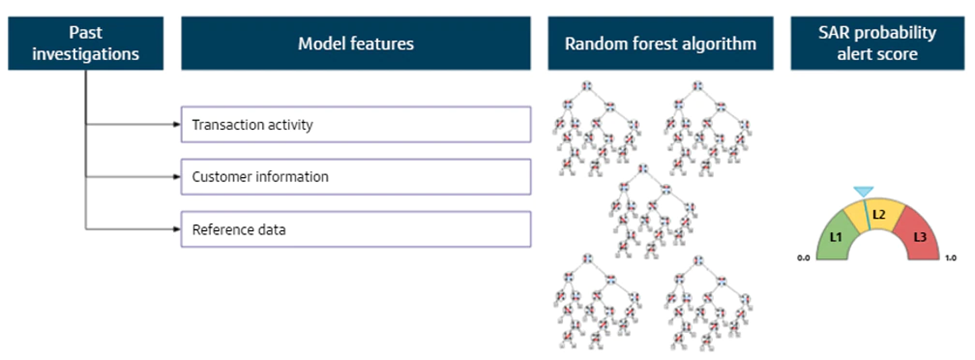
This replaces the traditional first-in, first-out workflow with a risk-based investigative procedure that leads to better, more efficient investigative results.
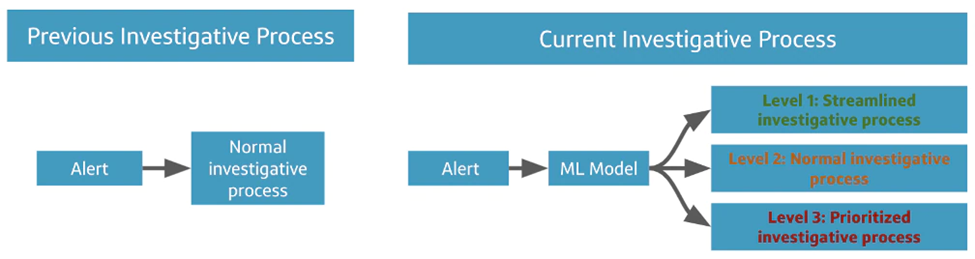
To reduce false positives, Capital One team used diversified AML team from five different countries to train the model representatively. This combined with monthly model monitoring, strict QA standards, and cross-testing are used to continually improve its performance and stability.
Currents Challenges & Recommendations
Given the highly regulated nature of their sector, ensuring transparency and explainability in their evolving deep learning models, especially in credit decisioning, is a key challenge. To address the same, internally, they can have explainability experts embedded in each product team, whereas externally, they can be involved in cross-industry explainability forums collectively working on visualizing deep learning models, and collaborating with regulators.
Another challenge is further entrenching ML operations as business-as-usual across the organization. To foster this AI-first culture, they can launch a skill development programs to equip their product managers with the necessary skills to identify opportunities for AI deployment and become model owners in collaboration with data scientists.
Conclusion
Capital One’s relentless focus on becoming an AI-first company has pushed the frontiers in automating important operational steps demonstrating significant efficiency gains. Going forward, as increasingly more self-evolving deep learning algorithms take charge of critical decision-making, Capital One needs to build a broader industry consensus and sensitize advocacy groups and regulators on the future of AI in banking and financial services. Capital One needs to reposition itself from a leader to an industry orchestrator.
References
(2) https://www.capitalone.com/tech/machine-learning/
(3) https://medium.com/capital-one-tech/this-is-machine-learning-at-capital-one-9329838cbcd0
(5) https://www.capitalone.com/tech/machine-learning/product-management-machine-learning-program/
(7) https://aws.amazon.com/solutions/case-studies/capital-one-machine-learning/
(8) https://www.unodc.org/unodc/en/frontpage/2011/October/illicit-money_-how-much-is-out-there.html
(11) https://www.capitalone.com/tech/machine-learning/edge-machine-learning-eno-virtual-card-numbers/
Thank you for sharing! I enjoy learning about how deeply AI/ML is rooted in Capital one by getting to know that you can basically find AI/ML-enabled technologies in every part of its business. This has led me to think about how they attract these talents in the industry as I am not sure if the bank is the first thing that a data analyst would think about when recruiting.
Thanks for the post, Anand. Capital One is certainly a leader in FinTech. Eno sounds very interesting – one number per merchant definitely mitigates fraud and risk. I’m assuming it’s also great for free trial subscription services, especially those that may be hard to cancel as I’m guessing you could easily close the virtual card to stop a service from repeatedly charging you. P.S: Fun fact – “Eno” is One spelled backwards.
Thanks for the post, Anand. I am a Capital One customer and can attest to their seamless integration of tech in their financial product offering. An additional service they have which I use weekly and I am sure has some level of AI/ML integration is Capital One Shopping, a Chrome plug-in that screens every single e-commerce checkout page you navigate into in order to offer you discount codes and alternative sites with cheaper prices. It is one of my favorite Capital One products. It allows Capital One to collect massive amounts of data from customers browsing the internet, which can then be leveraged by advertisers to provide targeted discounts and convert high-likelihood shoppers.