AstraZeneca: AI in Drug Discovery & Development
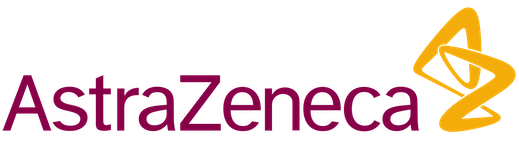
Currently, it takes a staggering 10-15 years and costs $2.6 billion to develop a new drug, and an astonishing proportion of this is lost in the 90% of candidates that fail. AstraZeneca is one of the world’s largest Pharmaceutical companies and is incorporating AI in every step of the R&D chain from drug discovery to launch to make this process safer, quicker and less costly.
Introduction
Currently, it takes a staggering 10-15 years and costs $2.6 billion to develop a new drug, and an astonishing proportion of this is lost in the 90% of candidates that fail. AstraZeneca (AZ) is one of the world’s largest Pharmaceutical companies and is incorporating AI in every step of the R&D chain from drug discovery to launch to make this process safer, quicker and less costly.
Applications of AI in Drug R&D
The first step is learning more about diseases. AZ is using knowledge graphs (networks of vast scientific data and the relationship between these) to gather new insights about diseases which would allow for more focused research. In order to learn more about pathology, we must gain a firm understanding of human genomes. While it would take humans several years to analyze and interpret this colossal data, AI can perform this more quickly and accurately. AZ’s pathologists spend hours analyzing histology samples, checking for biomarkers, in the hope of identifying patients who may be more responsive to drug candidates.
AZ’s AI-enabled image analysis (similar technology to self-driving cars) can identify biomarkers 30% faster than human pathologists.
https://www.youtube.com/watch?v=jRd1psHY-XU
After this, AZ uses AI to streamline efforts in drug discovery. It typically takes years of scientific research and costs millions of dollars to discover potential compounds that target diseases. Machine Learning and Chemistry automation can drastically shorten this process and cut costs by 75% by finding patterns in large datasets. AI in drug discovery is not replacing scientists but allowing them to use their time better and make more calculated decisions.
The next step is drug development. Randomized controlled trials (RCTs) are complex, expensive and time-consuming. AZ is using data analytics to mine through electronic health records (EHR) to improve clinical trial processes through faster patient identification and recruitment. Additionally, AZ is implementing AI and machine learning to capture more value from clinical trial data. While researchers are already proficient in analyzing and interpreting trial data to make recommendations, AI and ML can maximize the value of data collected in previous trials. Reusing data can aid in designing more robust trials and ultimately manufacture better treatments for patients.
Value Creation and Value Capture
AstraZeneca is a first mover in Big Pharma to implement AI and data analytics in every step of the drug R&D process. A more efficient drug discovery and development process utilizing AI not only reduces the time taken to get a viable product to market but cuts costs. This is eventually transferred to the patient. AZ creates value for the patient by launching a much-needed drug quicker and cheaper— the faster to market, the sooner lives are saved.
The utilization of AI in drug R&D provides opportunities for identifying more novel molecules, more quickly, and at less cost than the competition, thus capturing value with healthy profit margins. However, the purpose of AZ’s AI applications is not just to save time and money, but to provide its researchers with better data and more accurate analysis.
Challenges and Opportunities and Recommendations
Despite the brilliant applications of AI to bring a safer drug to market much faster and at a lower cost, its adoption remains low, and this is largely due to lack of trust. AI algorithms, especially in the early stages make mistakes and only become reinforced as the machine learns, and this often requires human refinement. Since many AI tools are still immature, there will continue to be widespread skepticism of the technology.
Another issue is that the complexity of AI and its various models make it very difficult to explain how it works, making adoption harder. Even within companies that adopt AI, employees feel threatened that machines will replace them, and this is another reason why adoption is low.
As more companies start to implement AI capabilities, there is a necessity to recruit data scientists and AI experts. Unfortunately, these highly skilled individuals are scarce, which creates a barrier for Pharma companies. AstraZeneca could invest in their current scientists to obtain further training in AI and reassure staff that technology will not replace staff as long as they are willing to work with AI.
In the US, the FDA is responsible for drug regulation. The current R&D process is tightly regulated with RCTs being the gold standard for drug development. As Pharma companies try and reinvent the wheel by implementing AI to increase efficiencies, they must overcome regulatory hurdles from the FDA. AZ must work with the FDA and comply with established policies regarding the use of AI.
While there are several challenges, AI in Pharma creates opportunities. With the current COVID-19 pandemic, there is an urgent need for a vaccine or therapeutic treatment, and the world cannot wait 10-15 years for a solution. AstraZeneca already has comprehensive AI capabilities that can speed up the entire R&D process. Smaller biotech companies with fewer resources and capital have already discovered molecules using AI that can potentially be effective against COVID-19. There is no reason why AZ cannot either use their own AI technology or partner with these biotech companies to combat the pandemic.
Sources
https://www.wired.com/brandlab/2019/07/machine-learning-is-transforming-drug-development/
https://www.astrazeneca.com/what-science-can-do/artificial-intelligence.html#datatoknowledge
https://www.statnews.com/2019/11/01/ai-revolutionize-drug-discovery-experts-involved/
Great post, Partha! In the artificial intelligence age, what do you see as the biggest competitive advantages for individual pharma companies? Access to data, the algorithms themselves, operating model, or something different?
I am also excited by future prospects of using unsupervised machine learning algorithms to identify pathology features that might serve as targets for new therapeutic intervention and drug features that might indicate better safety/efficacy profile. I agree with you that the current success rate is too low, costs too high, and timeline too long in drug discovery.
Hi Joe,
Thanks for your kind words. Very interesting question. From what I gather, access to data is the most valuable resource. It is my understanding that external data is not nearly as valuable since its reliability and accuracy cannot be verified, so the more internal data a company has, the better. Therefore, Life Sciences companies that are nurturing their own AI capabilities such as AstraZeneca and Phillips are ahead of the game when compared to Big Pharma companies that are partnering with AI-enabled biotechs. As the wealth of data strengthens, the more robust the machine learning models become (from my understanding anyway). One thing I think that AstraZeneca is doing that is beyond its competition is that it is utilizing AI in all steps of the R&D chain, whereas some of the competitors are only using the technology for discovery or development.
I too am excited at the prospect of healthcare shifting from supervised to unsupervised machine learning and seeing how the life sciences industry capitalizes on it.
Thanks for sharing, Partha! It’s definitely interesting to think about how AI can increase the speed of drug development in the times of COVID-19. As some regulations have been relaxed in health care during this crisis (particularly around telehealth), I am curious if resistance to AI will remain as people start to recognize the importance of increasing speed to market in times like these. I still see clinical trials as a challenge that is difficult to speed up, but using AI in other parts of the development process could greatly improve drug development speed.