Free but Not Equal?

We expect all other consumer products to meet a set of requirements, but for some reason that’s not the case with technology
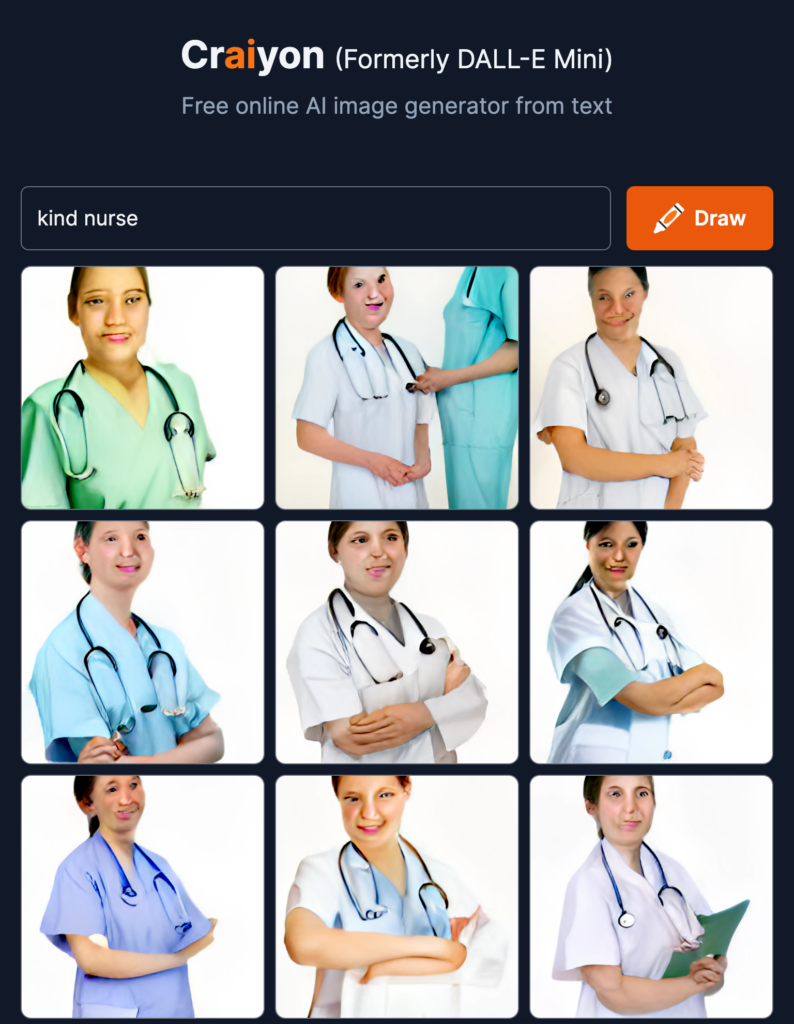
Craiyon at the bottom of the page has a warning that its results may “reinforce or exacerbate societal biases” because “the model was trained on unfiltered data from the Internet” and could well “generate images that contain stereotypes against minority groups.” So I decided to put it to the test.
Using a series of prompts ranging from antiquated racist terminology to single-word inputs, I found that Craiyon indeed often produces stereotypical or outright racist imagery. For the example screenshot, I typed in ‘Kind Nurse’ and the results all showed relatively light-skinned only women nurses. Even though what I tested underscores all the hard work that researchers have figured out on how to train a neural network, using a huge stack of data, and producing incredible results. However, we have been seeing these algorithms pick up hidden biases in that training data, resulting in an output that is technologically impressive but which reproduces the darkest prejudices of the human population for free.
Indeed, Chiwon! Some of these applications have accelerated our society’s biases like none before due to issues such as non-representative training data sets, inaccurate evaluation function definitions, and lack of developer diversity at the stage of algorithm development. Be it resume shortlisting (Amazon case) or processing of college admissions, voice-based personal assistants to text-to-image converters, we have seen that across use cases. How various evolving AI governance frameworks catch up to this reality would be interesting to see. Thanks for your post!
That is so strange! Even before reading your post, I noticed all the “kind nurses” that were generated were all female and light skinned. Also their hair styles are the same (bundled in a pony tail in the back) and with a brunette color. Arm positioning also seems to be the same. All of this makes you question what exactly is the informing data set and how can make sure there is sufficient diversity such that the results aren’t biased.
Thank you for the post! It really makes me think the data training is skewed because of the lack of diversity among creators that is reinforcing the societal biases in the AI result.
Hi Jiwon, thank you for your post! It’s disappointing that these hidden biases are really obvious, but when they try to train AI, no one really thinks about fixing the problem and even leaving the problem for the public.
Thank you, Jiwon. Yes – the homogeneity in the women in striking (racial, gender, stance, hairstyle, dress). The only diversity I see is the direction of the stethoscope around their neck… ha! What also surprises me is that Craiyon doesn’t display “kindness” in their batch images beyond the nurse’s smile. Kindness should conjure images of understanding, helpfulness and care… these images just show women standing alone with their arms crossed. The only image demonstrating human interaction is the top-middle image of a figure in scrubs grabbing a nurse by the arm – that doesn’t seem particularly compassionate! Your Craiyon images further show how AI struggles to grasp the softer elements of the human experience.
Thanks for the post, Jiwon. I wonder if AI can be trained to recognize these biases and the stereotypes that it reinforces. Is improving AI to be cognizant of societal biases and address them in outputs a lost cause that demands human intervention? Will data scientists and ML researchers need to “break” the automatic learning loop inherent in AI to fix this issue, or is it something we can train the AI itself to fix?
Jiwon, thank you for your post and for bringing this discussion to the table. It is very relevant to highlight the biases that these platforms still have and promote in their results. Last week I read a case about racial discrimination on Airbnb and how its algorithm also reinforced stereotypes to promote certain accommodations. I think it is time for users to start penalizing these actions and demand from companies taking actions to deal with this issue.