Verizon: Battling crime through Machine Learning
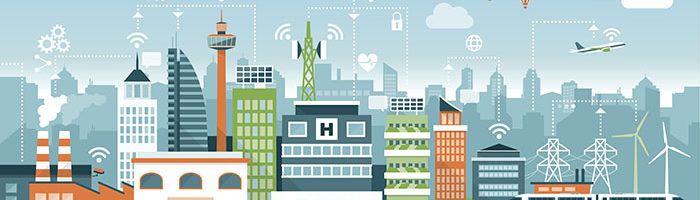
How Verizon is trying to predict and prevent crime by building smart cities
Every second counts
In an emergency, every second counts. After a crime occurs, there is little time to assess the situation and determine the best solution. In large cities, multiple agencies have to coordinate and reach a consensus before anyone can act. Therefore, agencies have to be nimble enough to act on incomplete and changing information. These challenges lead to long response times, high fatality rate, and inefficient allocation of resources.
Annually, Emergency medical service (EMS) responds to about 37 million emergency calls [1]. The average response time is eight minutes but can be as high as 14 minutes [2]. Moreover, the response time and number of emergency calls continue to increase as cities become denser and more citizens, especially in rural areas, gain access to technology [3].
What if first responders didn’t have to wait for an emergency to occur and could react proactively? That’s the theory behind Verizon’s Smart Communities initiative.
Battling crime with machine learning
Verizon Smart Communities is an initiative aimed at “helping communities stay smart, connected, and safe” [4] by utilizing data connectivity and machine learning to help cities better provide for its citizens.
As part of this initiative, Verizon aims to make police, hospitals, and first responders more efficient by helping them predict and respond to emergencies and crimes before they occur. Verizon’s Real Time Response System incorporates data from multiple sources and provides a data-based recommendation of when and where an emergency is likely to occur [5]. The Real Time Response System utilizes machine learning to analyze trends, perform risk analysis, and measure intervention efficiency. For instance, Verizon uses machine learning to analyze more than 1 million security events every day to help authorities better plan and respond to emergencies [5].
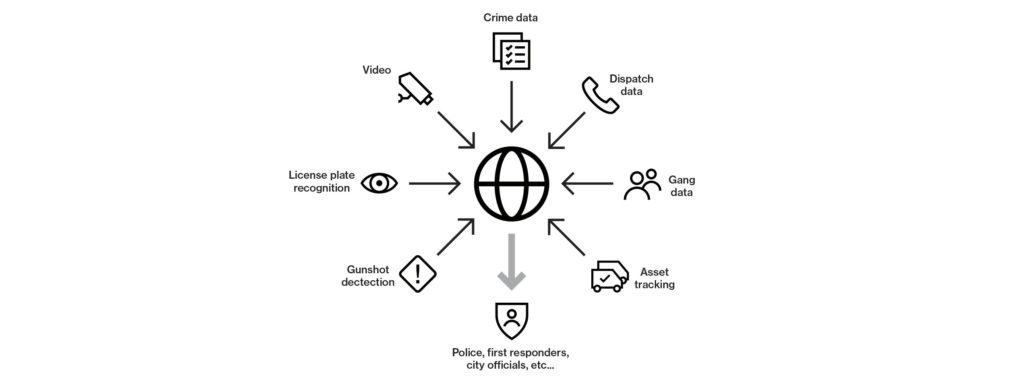
Verizon’s machine learning algorithm aims to improve resource allocation, highlight crime patterns, and even prevent criminal activity. According to a McKinsey report, implementing machine learning at the city level has the power to reduce crime rate by 30 – 40%, save 30 – 300 lives per year, and improve first respondent’s arrival time by 2 – 17 minutes [6]. As Verizon expands to more cities and establishes more data centers, the algorithm becomes increasingly more accurate.
Verizon is doubling down on its commitment to collect and analyze data in order to provide actionable insights. With over 2.58 million square miles of coverage, Verizon is able to attain and monitor data in real time in every city in the world. Moreover, the company has invested more than $126B in network infrastructure since 2000 and continues to invest at an exponential rate [5].
As Verizon continues to develop its Real Time Response System, there are multiple challenges the company faces:
- Verizon needs to establish and test a backup system that can continue to function during an emergency situation. For instance, the response system cannot have any downtime as authorities rely on it for daily activities and routines. A viable solution is to partner with a reliable data management organization such as FirstNet to ensure reliability [5].
- The data management systems have to become a scalable solution that are able to process exponentially more data as more cities start to utilize the network. While Verizon is trying to improve its current technology and infrastructure, they should focus more on adding to their 5G network. 5G network has bandwidth to store, analyze, and process data [5].
- Verizon needs to find a way to carefully navigate the grey area of data privacy. Consumers are inherently skeptical of corporations utilizing private data, but the system needs as many inputs as possible to generate reliable recommendations [7]. Verizon should start a campaign that explains consumers the positive impact the system can have on cities and the importance of their initiative.
Questions to consider:
- The Real Time Response System utilizes historical crime data, license plate recognition, gang data, and various other data sources to determine the likelihood of a crime occurring. Similar machine learning analytics have been found to be biased against blacks and other minorities [8]. What role does/should Verizon have in addressing these concerns?
- Data is a commodity that is increasingly becoming more valuable. Due to Verizon’s business model, they are able to collect data as a byproduct of their core business. Should Verizon have to pay/reimburse consumers for utilizing the consumer’s personal data?
Word Count [722]
Sources
[1] Mell, H., Mumma, S., Hiestand, B., Carr, B., Holland, T. and Stopyra, J. (2017). Emergency Medical Services Response Times in Rural, Suburban, and Urban Areas. JAMA Surgery, 152(10), p.983.
[2] Resourcecenter.911.gov. (2018). National 911 Profile Database. [online] Available at: https://resourcecenter.911.gov/code/9-1-1ProfileDatabase.aspx [Accessed 13 Nov. 2018].
[3] U.S. (2018). Be prepared for ambulance wait times. [online] Available at: https://www.reuters.com/article/us-health-emergency-response-times/be-prepared-for-ambulance-wait-times-idUSKBN1A42KQ [Accessed 13 Nov. 2018].
[4]Products, S., Accounts, M. and Solutions, S. (2018). Smart Communities. [online] Verizon Enterprise Solutions. Available at: http://www.verizonenterprise.com/view/solnsbriefs/12376/smart-communities-solutions [Accessed 13 Nov. 2018].
[5]Verizon Enterprise. (2018). Real Time Response Center. [online] Available at: https://enterprise.verizon.com/products/internet-of-things/smart-cities-and-communities/real-time-response-system/ [Accessed 13 Nov. 2018].
[6] McKinsey & Company. (2018). Smart cities: Digital solutions for a more livable future. [online] Available at: https://www.mckinsey.com/industries/capital-projects-and-infrastructure/our-insights/smart-cities-digital-solutions-for-a-more-livable-future [Accessed 13 Nov. 2018].
[7]The Independent. (2018). With smart cities, your every step will be recorded. [online] Available at: https://www.independent.co.uk/life-style/gadgets-and-tech/news/smart-cities-big-data-technology-mobile-phones-internet-computers-macs-laptops-a8308566.html [Accessed 13 Nov. 2018].
[8] Julia Angwin, S. (2018). Machine Bias — ProPublica. [online] ProPublica. Available at: https://www.propublica.org/article/machine-bias-risk-assessments-in-criminal-sentencing [Accessed 13 Nov. 2018].
Very interesting article. It’s inspiring to see how large companies are leveraging technology not only for profit but also to better society. As I read the article, I kept thinking about data bias in relation to the question you posed at the end. Until machine learning improves to the point where biases can be self-addressed within the system, it should not be used as a stand-alone solution but rather as a supplement to human operators.
Thank you for the interesting article. This technology reminds me of Tom Cruise’s 2004 film, “Minority Report”, where the police is able to predict and prevent violent crime from happening. I have two comments that I would like to pose:
a) In regards to your second question, in my opinion customers should be reimbursed for providing Verizon their personal data. Even now, data is a valuable commodity, and this should be recognized. Furthermore, by reimbursing customers, this would incentivize adoption. However, given the social benefit of this product, I would recommend Verizon appeal for financial benefits from the government, such as tax breaks.
b) I would also argue that a strong data governance model is required for this technology. Verizon would be receiving a lot of personal data from customers, and it would be prudent that a certain processes are put in place to regulate its use and to prevent abuse.