Twine Health: Pioneering Machine Learning in Chronic Disease Management
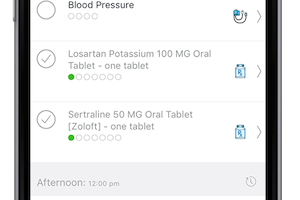
Twine Health is a digital health company with a chronic disease management application. Its platform draws heavily on machine learning to improve patient outcomes and save costs.
Twine Health is a digital health company that developed a cloud-based chronic disease management platform. This platform supports patients who have been diagnosed with a disease like hypertension (high blood pressure). Patients work with their physician and a health coach to develop an individual treatment plan, and the health coach monitors their progress with regards to the treatment protocol [1]. For example, if a patient stopped taking his or her medications, a health coach would work with then work with him or her to develop solutions (e.g., adjusting the timing of medication) [2]. Studies have shown that use of Twine Health improves health outcomes and reduces costs [2].
Twine Health has relied heavily on machine learning in its product development. Its platform has special functionality that allows health coaches to identify patients in need. In other words, the platform flags patients that are high priority (e.g., if a patient is consistently not taking his or her medications, or if their health data – such as blood pressure readings – are worrisome) [3]. The ability to rank patients with regards to their health is rooted in Twine’s use of machine learning techniques. Twine used feedback from clinicians to identify high-risk patients based on healthcare platform data. These inputs were then used to develop a smart algorithm that triages patients based on their health [3]. Health coaches can view a list of their patients ranked from highest priority to lowest priority, and they can use this information to structure their workflow accordingly [2].
Twine’s use of machine learning has been essential to its value proposition. Per Twine’s company materials, typical health coaching platforms used to support health coaches have poorer clinical outcomes (56% blood pressure goal achievement vs. 86% through Twine) and lower cost savings ($2,693 required for hypertension care per patient per year vs. $1,498 through Twine) [3]. Additionally, health coaches that use traditional chronic disease management platforms can only manage ~100 patients at a time, while health coaches that use Twine’s platform can manage ~300 patients at a time [3]. Twine’s reliance on machine learning is therefore a key differentiator from its competitors.
With regards to what Twine is doing to address machine learning in the future, it is continuing to expand its capabilities to improve the performance and efficiency of health coaches. Over the short term, Twine hopes to introduce features that can triage messages from patients [4]. Additionally, Twine is using machine learning to develop predictions regarding patient health outcomes [4]. For example, the platform may one day be able to predict a patient’s blood pressure results based on medication adherence data. Over the medium term, the company plans to expand into conversational artificial intelligence [4]. In other words, Twine will use voice recognition software and natural language processing to allow coaches and patients to communicate verbally through the platform. In order to facilitate this feature, the platform needs to use smart algorithms that recognize medical language and appropriately categorize this language into structured data housed on the Twine platform.
In terms of other steps Twine should take, I think the platform should begin to use machine learning to provide treatment recommendations. As noted above, each patient on the platform has an individual treatment plan. When issues arise (e.g., a patient not remaining adherent to their medications), health coaches have to contact physicians to adjust that patient’s plan. These physicians adjust the plan based on their own clinical expertise. However, if Twine were able to successfully apply machine learning to analysis of past treatment patterns, then coaches and physicians could use outputs such as personalized medicine recommendations to adjust a patient’s plan.
Another area Twine could explore with regards to machine learning relates to its recent acquisition by Fitbit in February 2018 [1]. The healthcare industry as a whole is still struggling to determine how to incorporate non-traditional data sources (e.g., Fitbit wearables) into clinical decision-making. Twine could potentially work on integrating these data sources into its existing machine learning algorithms (which identify which patients are at highest risk for poor health outcomes). In this sense, data from these sources could be leveraged to enhance Twine’s existing machine learning algorithms.
Finally, some open questions regarding this issue include the following: 1) How should machine learning algorithms in healthcare delivery be treated from a regulatory perspective? For example, should Twine be required to seek FDA approval given that its patient triage function directly influences patient care? 2) What degree of oversight from a physician is required in the implementation of this tool? For instance, if the Twine platform is able to use machine learning to recommend changes in when patients take their medications, does a physician need to sign off on these changes every time? (784 words)
[1] Huckman, R.S., and A.D. Stern. “Why Apps for Managing Chronic Disease Haven’t Been Widely Used, and How to Fix It.” Harvard Business Review Digital Articles (April 4, 2018).
[2] Huckman, R.S., Stern, A.D., and M.G. Preble. “Twine Health.” HBS No. 9-615-068 (Boston: Harvard Business School Publishing, 2015).
[3] Moore, John. “Part Machine, Part Human: Artificial Intelligence, Health Coaching & Behavior Change.” May 10, 2018. Massachusetts Medical Society, The Promise and Pitfalls of Transforming Health Through Technology and Information course. http://www.massmed.org/Continuing-Education-and-Events/Conference-Proceeding-Archive/John-Moore,-MD,-PhD-Slides-2017/, accessed November 2018.
[4] Arndt, Rachel. “Bridging the healthcare gap with digital coaching.” Modern Healthcare, December 9, 2017. https://www.modernhealthcare.com/article/20171209/TRANSFORMATION03/171209944, accessed November 2018.
This is so cool! I love solutions that focus on helping people manage their health and the fact that Twine is figuring out how to do it in an economically and sustainable way is amazing. In addition to the questions you raised, I’m also curious about a couple of others:
1. What participation is required on the part of the patient, and how do you ensure/motivate patient participation?
2. How does Twine account for patient-reported information that could potentially harm the validity of the data that is being used as the input to their machine learning algorithms?
Excellent introduction to this novel chronic disease management tool! Making health coaches more efficient and effective is critical to delivering patients the best possible care. Clearly using machine learning to direct patient care will require some sort of oversight to ensure quality care. I can easily imagine a world where a machine learning tool would provide care recommendations and a physician would be required to approve them. Alternatively, for things like adjusting IV infusion rates in ICU patients, a machine learning based tool could independently adjust patient care. Such a scenario would of course require much stronger regulatory oversight. Liability would probably be a central issue. This is all good food for thought.
Great essay! I really appreciate your thoroughness on a very important topic. I am really interested in what the patient involvement needs to be. Coming from the healthcare industry as well, I see how difficult it is in chronic disease management to get patients to adhere to their medicine. I wonder if additional technology can help bridge the gap and integrate seamlessly with Twine Health.
Eli Lilly recently developed a pill bottle with sensors for Alzheimer’s patients. Unfortunately, these patients often have co-morbidities (like diabetes) and can often forget their medication. At the time medication needs to be taken, the pill bottle sensor lets the patient know where the pill bottle is located and reminds them to take the pill. If the pill bottle moves and is located close to the patient, then the sensor sends a notification to the caregiver that the pill was most likely taken. I wonder if a technology like this could be used with the Twine Health app to allow health coaches to monitor patients.
Source: https://www.zdnet.com/article/how-sensors-enabled-eli-lilly-to-improve-the-patient-experience/
This is fascinating and I hope that the application of machine learning in the health care industry will reduce the cost of health care around the world and lead to better outcomes. It looks like Twine Health is doing exactly this. I think you are right in thinking that Twine should look into partnerships with Fitbit etc. The real opportunity I see there is better patient tracking and preventative care. For example, by collecting this type of data physicians can notify patients when they are not keeping to their exercise or diet regimes. They can also find the correlation between certain life-styles and the benefits they may or may not offer in managing chronic diseases. Consumer data can also be used to benchmark patients so they can be warned of health risks that result from their particular life-style data profile. I think another major issue to consider here is data privacy and who will have access to this very personal data about people’s lives. Will health insurance companies also have access and be able to charge different rates based off your data profile?
I loved this essay so much, Sam! Particularly because, when I was at McKinsey, we designed a very similar application to be used by Ministry of Health in one of the emerging markets. I really believe that due to the cost effectiveness and ease of monitoring, leveraging such applications for treating chronic diseases is the future of treating these diseases. These applications are especially important for emerging countries, because of lack of transportation in rural areas, scarcity of doctors and lack of awareness among patients. I believe that thanks to the advancements in machine learning in health sector, we are going to have healthier communities in the future.