The Artificially Intelligent Brewer: Carlsberg’s Breakthrough Project
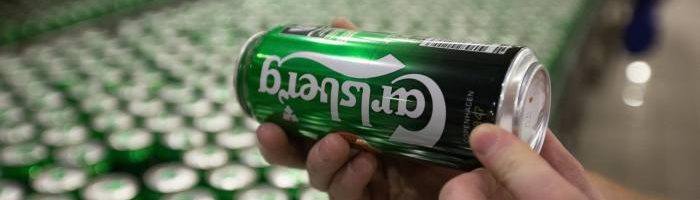
Humans have been producing beer for over 5,000 years, and it has become our most consumed alcoholic beverage worldwide. But is brewing an art or a science? The rise of the craft beer segment suggests that many of us perceive it as an art, but machine learning is gradually reinventing both the process and product development aspects of this traditional industry.
Humans have been producing beer for over 5,000 years [1], and it has become our most consumed alcoholic beverage worldwide [2]. But is brewing an art or a science? The rise of the craft beer segment, which represents 13% of the US beer market by volume and is growing volume at 5% per year despite a decline in the overall market [3], suggests that many of us perceive it as an art.
However, machine learning is gradually reinventing both the process and product development aspects of this traditional industry. Carlsberg, a global brewing company headquartered in Denmark, is eager to capitalize on the benefits of these trends to gain an edge in this highly competitive market. In recent years, the Danish brewer has struggled due to stagnating consumption in its key markets, Russia and China, as well as fierce competition from industry leader Anheuser-Busch InBev [4] and an increasing number of local microbreweries.
In response, at the beginning of 2018, Carlsberg launched an ambitious “beer fingerprinting project” that is estimated to cost $4 million and will occupy its researchers for the next 3 years [5]. The goal of the project is to map out the flavor and aroma profiles of a wide range of beer ingredients, then build a predictive engine that could indicate the taste of a new beer based on a set of specified ingredients. To achieve this mission, Carlsberg’s Research Laboratory has teamed up with Aarhus University to develop high-tech sensors, the Technical University of Denmark to figure out how to implement them in different fermentation scenarios, and Microsoft to analyze their signals using machine learning algorithms [6].
Jochen Förster, Director and Professor of Yeast Fermentation at Carlsberg Research Laboratory, believes that eventually these sensors can cut the new product development process – which typically takes 8 to 24 months – by one third [7]. The traditional beer innovation process is highly iterative, propelling Carlsberg to create hundreds of small microliter brews daily [6]. By reducing waste, Carlsberg’s “fingerprinting” technology could significantly reduce R&D costs in addition to shortening the time-to-market for new products.
While no such rapid technology for the discrimination of complex flavor mixtures is currently used in industry [7], the Journal of Food Science published a paper in March 2018 that directly links the sensory attributes of beer to its foam-related parameters and color. Faculty at the University of Melbourne used a robotic pourer (RoboBEER) to measure these visible attributes, then developed an artificial neural network regression model that predicts the intensity levels of 10 sensory descriptors: yeast, grains and hops aromas, hops flavor, bitterness, sourness, sweetness, viscosity, carbonation, and astringency [8]. The correlation between the outputs of this model and an expert panel was 0.91 [8], indicating that a refined version of the algorithm may be able to replace human opinion in the future.
Given its strong cultural emphasis on research [6], Carlsberg is well-positioned to be the first brewing company to apply this machine learning technology and should continue to pursue the “beer fingerprinting project” in the medium term. However, a lot more work needs to be done to train an algorithm to detect the nuanced flavor differences between hundreds of beer ingredients and predict the resulting flavors when those ingredients interact in complex mixtures.
While pursuing this breakthrough technology in product development, Carlsberg management should also consider using machine learning to improve the efficiency of its production process – especially with regards to quality control. For example, the sensors that Carlsberg is developing could be used to rapidly screen and evaluate beer quality at the end of a production line [8]. They could also be embedded throughout the production process to track vital metrics, automatically detect problems, and trigger the necessary steps to save the work-in-process batch of beer, an approach that Deschutes Brewery, a craft brewery in Oregon, is already experimenting with [9]. These applications could improve the yield and output rate of Carlsberg’s brewing process, while decreasing labor content.
In the medium term, Carlsberg should research ways to connect consumer feedback to its flavor prediction engine, such that the system could suggest recipes that optimize for consumer tastes or forecast demand for a given recipe. This idea is already being explored by IntelligentX, a craft brewery in London, which inputs consumer feedback into a “complex machine-learning algorithm” to tweak its beer recipes [10].
As the world’s fourth largest brewer [11], Carlsberg has a lot to gain if it successfully advances the application of machine learning to beer production and innovation. However, given rapid growth of craft breweries, which amplify the role of human artistry and tend to be less consistent from a quality standpoint, it is still an open question whether consumers would react positively to beers that represent even more science than art. (Word count: 781)
[1] Andrews, Evan. “Who Invented Beer?” History.com. January 8, 2014. Accessed November 12, 2018. https://www.history.com/news/who-invented-beer.
[2] Rutishauser, Estevez, Stefan Rickert, and Frank Sänger. “A Perfect Storm Brewing in the Global Beer Business.” McKinsey & Company. June 2015. Accessed November 9, 2018. https://www.mckinsey.com/business-functions/marketing-and-sales/our-insights/a-perfect-storm-brewing-in-the-global-beer-business.
[3] Watson, Bart. “National Beer Sales & Production Data.” Brewers Association. Accessed November 14, 2018. https://www.brewersassociation.org/statistics/national-beer-sales-production-data/.
[4] Milne, Richard. “Carlsberg Turns to AI to Help Develop Beers.” Financial Times. December 26, 2017. Accessed November 9, 2018. https://www.ft.com/content/be042eb2-e4cf-11e7-97e2-916d4fbac0da.
[5] “Denmark: Carlsberg Kicks off Beer Fingerprinting Project.” Inside Beer. Accessed November 12, 2018. https://www.inside.beer/news/detail/denmark-carlsberg-kicks-off-beer-fingerprinting-project.html.
[6] Ray, Susanna. “Can AI Help Brewers Predict How New Beer Varieties Will Taste? Carlsberg Says “probably”.” Microsoft. July 16, 2018. Accessed November 14, 2018. https://news.microsoft.com/transform/can-ai-help-brewers-predict-how-new-beer-varieties-will-taste-carlsberg-says-probably/.
[7] “Carlsberg Research Laboratory behind Beer Research Project Based on Artificial Intelligence.” Carlsberg Group. July 11, 2017. Accessed November 9, 2018. https://carlsberggroup.com/newsroom/carlsberg-research-laboratory-behind-beer-research-project-based-on-artificial-intelligence/.
[8] Viejo, Claudia Gonzalez, Sigfredo Fuentes, Damir D. Torrico, Kate Howell, and Frank R. Dunshea. “Assessment of Beer Quality Based on a Robotic Pourer, Computer Vision, and Machine Learning Algorithms Using Commercial Beers.” Journal of Food Science 83, no. 5 (2018): 1381-388. Accessed November 9, 2018. doi:10.1111/1750-3841.14114.
[9] Bass, Dina, and Mark Bergen. “Beer, Bots and Broadcasts: Companies Start Using AI in the Cloud.” Bloomberg.com. April 6, 2017. Accessed November 14, 2018. https://www.bloomberg.com/news/articles/2017-04-06/beer-bots-and-broadcasts-companies-start-using-ai-in-the-cloud.
[10] Hawkes, Will. “Beer 2.0 – Can AI Make Your Pint Taste Better?” Financial Times. June 02, 2017. Accessed November 9, 2018. https://www.ft.com/content/ffc3a680-4582-11e7-8d27-59b4dd6296b8.
[11] Milne, Richard. “Carlsberg Weighed down by Russian Restrictions on Beer Sales.” Financial Times. February 07, 2018. Accessed November 14, 2018. https://www.ft.com/content/2798e4be-0c01-11e8-8eb7-42f857ea9f09.
This seems like a very interesting potential solution for a large brewer like Carlsberg managing significant inventory and waste costs. I do wonder about the efficacy of a machine-learning solution being able to create new flavors that match the varying tastes of the day, such as bitter IPAs of the US West Coast or the more hazy, floral IPAs of the Northeast. Does a machine solution just tend toward the mean, thereby creating a relatively forgettable taste experience?
Super interesting concept to allow Carlsberg to test far more strains than their competitors in the same period of time. I would be curious, however, what the average cost is to acquire a small microbrewery. Given that Carlsberg is putting $4M into this project plus the cost of numerous research and learning hours, you wonder if they could have acquired a group of smaller microbreweries for the same cost that in the end could fulfill the same goal, new beer flavors and a younger outward appearance for the brand.
This is a great post! I think it’s fascinating to see how artificial intelligence could potentially lend itself to basically anything. Regarding your closing remarks – I think that it would be amazing to see an algorithm designing the “perfect beer” based on consumer feedback. Yet, as you mentioned, I do think that there is a big “creativity” / “artistic” factor that makes people’s relationship to beer so strong, especially nowadays with the increasing demand for more artisanal / craft beers.
Great article!
I love “Carlsberg” beer not only because of the taste but also the beer looks good! What I am trying to say is that some people might buy and drink beer because of experience. People are obsessed with microbreweries even though their beer quality is not that good or consistent as you mentioned. There would be tones of work for marketers to do for that reason even if the company has an AI brewing system, I guess.
I also wonder how they can measure the preference of every people from the globe. Can people say “I wan’ 3% more acidity and 5% less bitterness.”? Thanks for the fascinating topic and I love to see how Carlsberg’s innovation goes!
Really interesting, would love to try some of this. It almost feels like the new Spotify for beer. Maybe a machine can predict what I want before I know I want that thing myself, and while I am perfectly ok with that in other areas of my life (retail, music, general advertising), consumption somehow feels different. I do think there is a barrier, at least in craft beer, that is related to the art of creation and consumption. On the other hand, there has been resistance in every area where AI is prominent today, so this could very well be the next one.
In terms of ideas/questions, I wonder if Carlsberg and other brewers will have different regional taste experts input flavor data for different types of beer. I think the point above about NE IPAs vs West Coast IPAs is really interesting. Here, a machine couldn’t be properly trained by one individual, because each individual will taste slightly different notes in hops/other ingredients. If will have regional “taste data input-ers”, then how scale-able is this new form of recipe creation?
This is really interesting and reminds me of the Big Data case we read about Gap or even how Netflix leverages machine learning to develop new movies and television shows. I think it makes sense for these more “artistic” fields to leverage machine learning to better predict consumer tastes and develop products accordingly. However, I wonder what the consumer reaction will be in the future as more brands begin to leverage machine learning for product development. Will there be a reversion back to authenticity vs. perfection? Perhaps in the future, consumers may appreciate products that may not get it “right” but produced their product in a way that was not reliant on big data.
In the meantime though, a very cool and interesting topic!
Really interesting concept and well-written post! However, I do wonder if machine learning can replace the efficacy of human taste buds and if it can take into account the “creativity” of developing new recipes which might always be as per the scientific algorithms the “best match”. I’m also curious to know as you mentioned, how such algorithms can take into account changing consumer preferences. It lead me to think if there’s a potential to actually take this concept to create more personalized brews/beers for every individual and what the operational complexities and unit economics of those will looks like.
This is a fascinating article – and I love to have another TOM project related to beer! This article actually brings to mind another experiment which took place in the aging process of whiskey (https://qz.com/907619/the-many-scientific-ways-to-age-whiskey-many-years-in-just-a-few-days/). It seems to me that as we are able to break down the components of taste into their scientific precursors, the ability to artificially build high-quality products for quicker and cheaper will accelerate. However, I wonder if consumer tastes will evolve to fit this new paradigm. Craft beer, for example, is often a very emotional purchase in which craft beer aficionados purchase beer that they know has been created over a long development process by a master brewer. Convincing mustachioed hipsters in Somerville or Brooklyn to buy beer that was developed by an algorithm may be the most difficult challenge for a company like Carlsberg going forward.
Great article! My main curiosity revolves around the actual application of machine learning in a space dominated by “mind share”, or brand awareness. The highest market share beer is Bud Light, but I can guarantee that Bud Light is not the beer recognized for best taste – rather brand awareness and relative ubiquity. So my point is… although I understand how machine learning is helping Carlsberg test consumer tastes / reactions effectively and efficiently, but I would postulate that most beer consumers already have a perception on Carlsberg’s taste, and so improving the taste to be more geared toward consumer preference would not be as effective as “buying” new tastes – i.e., buying up smaller craft- and microbreweries. This would be faster and is a way to completely differentiate from the existing brand’s taste. Also, how much of the beer-drinking population knows the difference between “acidity” vs. “hoppy” vs. [other beer measurement]? As we have seen in our multiple beer cases, a majority of beer brands are owned by a conglomerate – namely AB InBev and SABMiller. However, this could also be a marketing tool – e.g., “Carlsberg beer has reinvented the product by defining the ‘perfect’ beer by using machine learning.” Gimmicky? Yes. Effective? Maybe.
I love the applications in terms of reducing waste and improving late-stage production testing efficiencies. I think this could mean huge things for process improvement and cost-cutting in the beer industry. Where I struggle is with machine learning in product development – it is hard to believe that a machine can create complex/nuanced flavors in ways that humans will appreciate (with their perceptive palettes and discerning tastes). I think your question of art vs. science is very appropriate here. Would AI beer be fairly standardized and one-dimensional? I’d be interested to see what the computers came up with. This would also extend to food/drink industries more broadly – would the artistry of cooking be become obsolete after the age of machine learning?
Such an interesting topic. I agree with each of your points, but my bigger picture takeaway is a bit of a ‘wow’ reaction that Carlsberg leadership jumped straight to ‘optimal taste’ as their initial application of ML. More “traditional” applications of ML like production quality and customer feedback seem like low hanging fruit for Carlsberg to cut their teeth on and develop the data science competency needed to take on such a complex tasting algorithm.